Innovative data clustering method improves drought prediction in heterogeneous landscapes using GEE-derived remote sensing indices
REMOTE SENSING APPLICATIONS-SOCIETY AND ENVIRONMENT(2024)
摘要
Drought is a complex natural disaster characterized by unique features influenced by environ-mental factors, particularly at a regional scale. Remote Sensing (RS) indices have proven to be valuable in assessing drought conditions. In this study, we utilized the five most commonly used RS indices: Normalized Difference Vegetation Index (NDVI), Deviation/Anomaly of NDVI (NDVI-Dev/ANDVI), Temperature Condition Index (TCI), Vegetation Condition Index (VCI), and 2-band Enhanced Vegetation Index (EVI2). These indices were extracted over a 30-year period (1988-2017) using Google Earth Engine (GEE) for modeling the Standardized Precipitation Index (SPI) with various time-spans (1, 3, 6, 9, 12, and 24 months) as the Ground-Based (GB) drought indices. The models were trained from 1988 to 2014 and tested on data from 2015 to 2017. To enhance RS-based drought modeling over the complex and diverse study area of Iran, we pro-posed two different data clustering methods based on distinct environmental factors - climatic condition and land-cover type - and compared the results. The first method employed an en-hanced-climate-based approach to improve upon the standard-climate-based method. This ap-proach considered variations in the environment caused by seasonal changes in different climatic zones, which had received less attention in previous studies. Unique Support Vector Machine (SVM) models were trained using various clusters of inputs/outputs to assess the efficiency of the RS indices in predicting different drought conditions under various circumstances. Furthermore, we introduced an innovative data clustering method based on RS-derived land-cover similarity, which resulted in further improvements in drought modeling compared to agroclimatic zoning. The fusion-based drought modeling approach, using all RS indices as inputs in the enhanced-climate-based and land-cover-based methods, demonstrated an aggregated Overall Accuracy (OA) of 92.93% and 95.11% for predicting SPI-3, respectively. Notably, within the land-cover -based method, TCI exhibited the highest aggregated performance compared to other RS indices in predicting SPI-3 with an OA of approximately 95%.
更多查看译文
关键词
Remote sensing index,Drought,Climate,Land -cover,Google earth engine
AI 理解论文
溯源树
样例
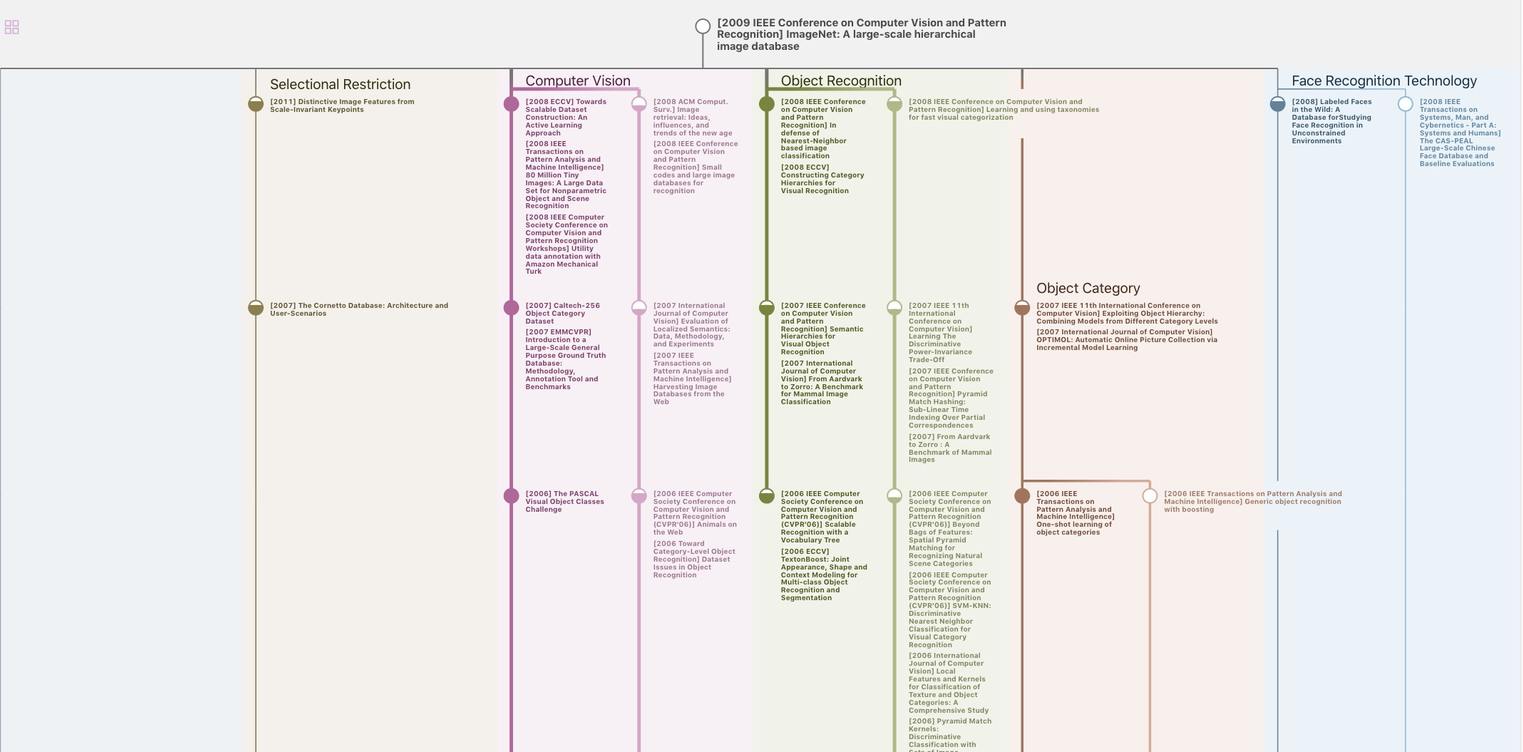
生成溯源树,研究论文发展脉络
Chat Paper
正在生成论文摘要