Detection of Abnormality in Cognitive Test Scores Using Metric Learning
2023 IEEE 4th International Conference on Pattern Recognition and Machine Learning (PRML)(2023)
摘要
We investigate deep learning neural networks to detect anomalies in cognitive test scores that are generated by either a trained human coder or a classification program based on the input of clock drawing images. We have developed a system, SN_AD (Siamese Networks for Abnormality Detection) in ordinal classification. SN_AD consists of three Siamese networks, one models class k, and the other two model the two neighboring classes, class k-1 and k+1, and an anomaly detection algorithm, OCAD (Ordinal Class Anomaly Detection). The Siamese networks were trained using metric learning and used to generate class distance distributions. The OCAD algorithm calculates the distances of each test image x to the distributions of class k, k-1, and k+1 using the Siamese networks and detects class score anomalies based on statistical analysis of x being out-of or indistributions of class k and its two neighboring class k-1 and k+1. Experiments were conducted on two sets of images collected through Clock Drawing Test (CDT) in the National Health and Aging Trends Study (NHATS), which is commonly used to detect cognitive impairment. The experimental results show that the proposed system, SN_AD is effective in metric learning of class-specific distributions, detecting coding inconsistencies, and correcting coding errors.
更多查看译文
关键词
anomaly detection,Siamese networks,metric learning,ordinal classification
AI 理解论文
溯源树
样例
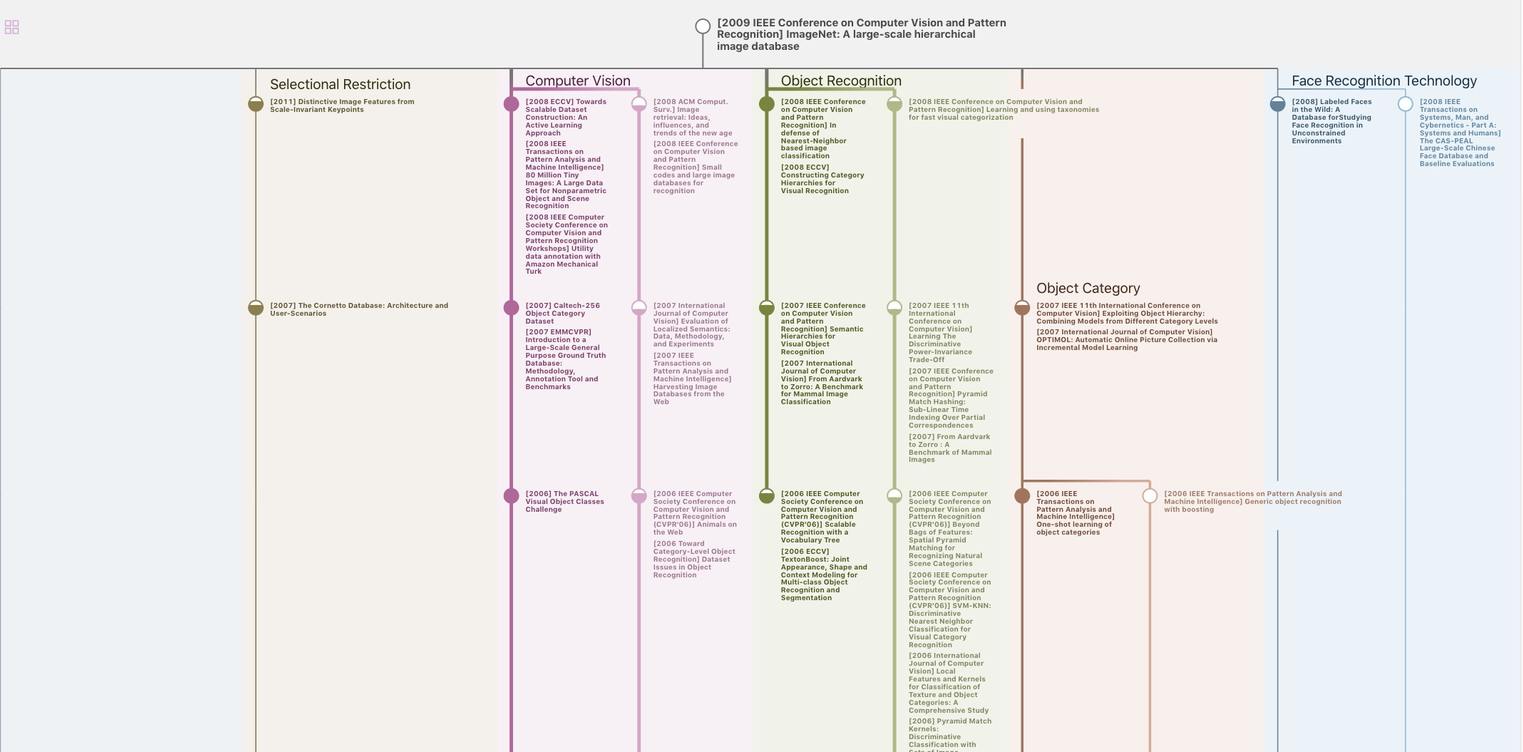
生成溯源树,研究论文发展脉络
Chat Paper
正在生成论文摘要