Now You See Me: Robust Approach to Partial Occlusions
2023 IEEE 4th International Conference on Pattern Recognition and Machine Learning (PRML)(2023)
摘要
Object occlusion presents a significant and complex challenge in computer vision, requiring effective solutions. While convolutional neural networks (CNNs) have shown success in regular image classification tasks, they often struggle when confronted with images containing partial occlusions. Partial occlusion refers to instances where an object is partially obscured by another object or space. Solving this problem holds immense potential for various applications, with a particular focus on the domain of autonomous driving. Autonomous vehicle research has emerged as a prominent topic in recent years, and it involves numerous situations where partial occlusions occur. These occlusions can manifest in the form of obstructed driving signs, pedestrians, or other objects, occurring at varying angles. Beyond the realm of autonomous driving, addressing the challenges of partial occlusion can also contribute to video analytics in traffic data, enabling crime prevention, income-level prediction for different groups, and more. In this study, we present our approach, which involves creating a synthetic dataset by augmenting the Stanford Car Dataset with occlusions of various sizes and types. Subsequently, we conduct a comprehensive analysis employing state-of-the-art models, investigating the impact of different occlusion proportions and types on model performance. Our research aims to shed light on the robustness of various models in the face of partial occlusions and their performance when trained on both occluded and unoccluded images. These insights can play a vital role in the development of more reliable autonomous driving systems and other computer vision applications.
更多查看译文
关键词
Partial Occlusion,Object detection,Deep learning
AI 理解论文
溯源树
样例
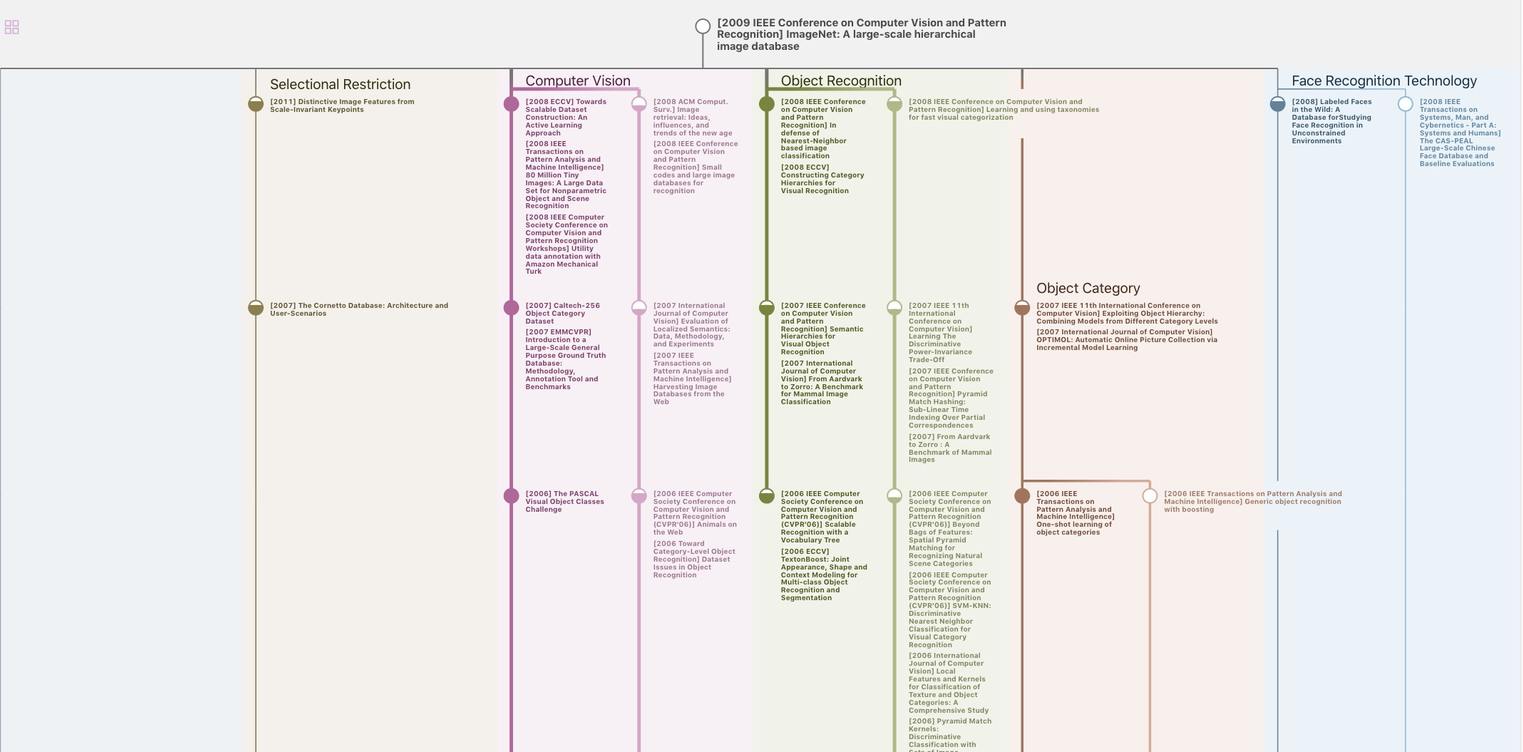
生成溯源树,研究论文发展脉络
Chat Paper
正在生成论文摘要