A hybrid model integrating artificial neural network with multiple GARCH-type models and EWMA for performing the optimal volatility forecasting of market risk factors
EXPERT SYSTEMS WITH APPLICATIONS(2024)
摘要
The 2008 financial crisis has highlighted the lack of precision in the market risk metrics that financial institutions must report to the regulator. The use of Machine Learning techniques in stock markets and the treasury (Front-Back Office) of financial institutions is a key tool for optimizing its own resources, internal processes and risk measures. We propose a hybrid methodology to better capture the volatility of market risk factors with Value-at-risk models in periods of stress, but also in periods of stability compared to traditional metrics. This hybrid model uses different types of artificial neural networks and traditional metrics to perform the optimal forecast of volatility applied to the main market risk nodes of the Spanish stock market. We use data from the following main market risk factors: returns on Santander Bank shares, the Spanish Stock Market Index, Euro/Dollar exchange rates and the index that measures the total return performance of a funded long credit position in the on-the-run iTraxx Crossover 5-Year-Index. Our contribution is a hybrid model that combines correct sequential pattern learnings with an improved prediction performance in the volatility of market risk factors. Our findings show that the Support Vector Machine and the Long-Short-Term Memory Model present better prediction results in all factors in the stability periods. Therefore, the proposed method is promising for application in risk management systems.
更多查看译文
关键词
Market risk,Value-at-risk,Machine learning,EWMA,GARCH,ANN LSTM,SVM
AI 理解论文
溯源树
样例
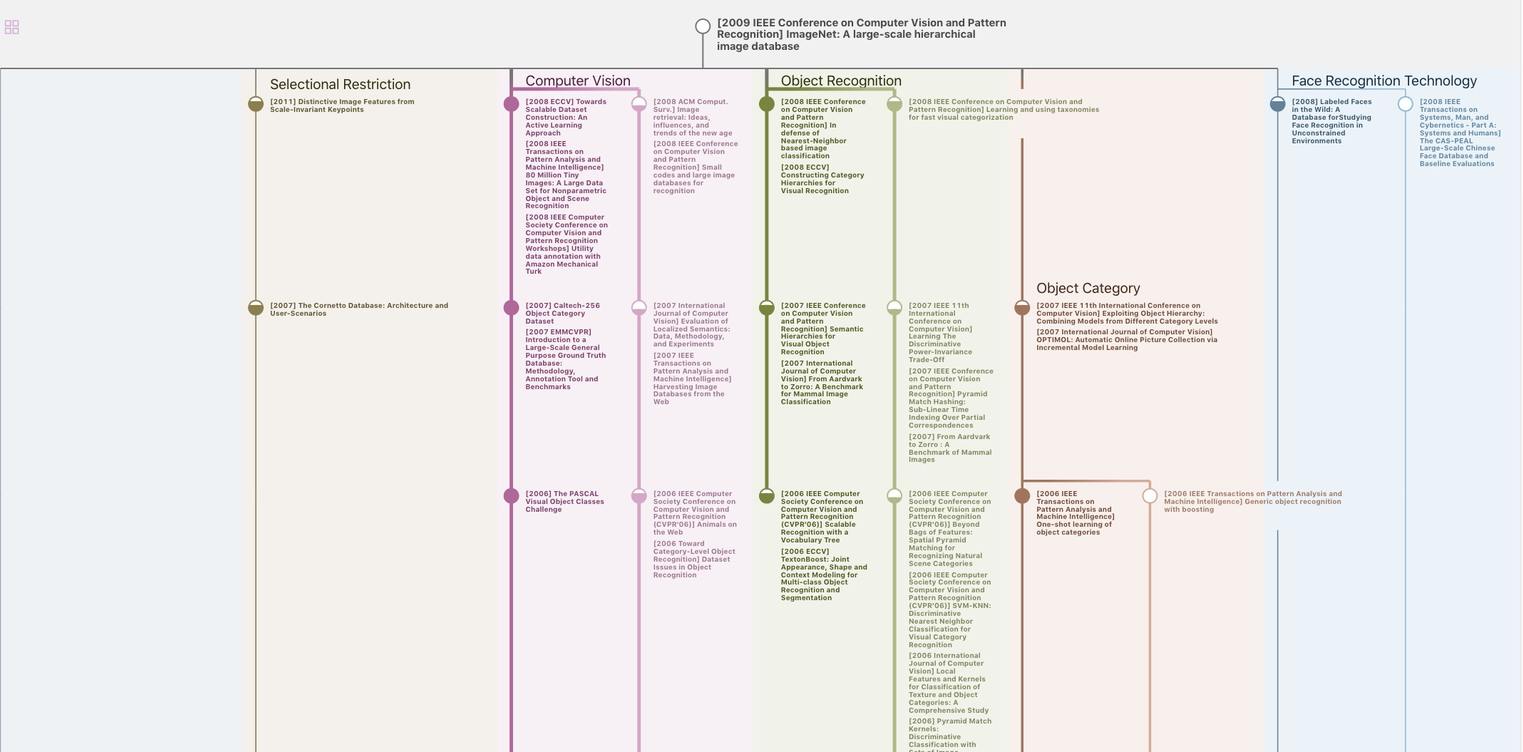
生成溯源树,研究论文发展脉络
Chat Paper
正在生成论文摘要