A progressive reconstruction modeling method for aeroengine performance space based on sequence correlation
MEASUREMENT(2024)
摘要
Due to the complex internal structure of aeroengine and the constraints of sensor installation, it is often impractical to deploy many sensors inside the aeroengine to monitor the key internal parameters. As a result, it is impossible to realize the goal of module performance evaluation. Relevant learning strategies for parameter reconstruction using limited measurement parameters have been studied to solve this problem. Firstly, because the measurable data is time series data containing noise, an attention mechanism about sequence correlation is adopted. This mechanism can gradually decompose complex time series. At the subsequence level, sequence information aggregation is carried out to improve information utilization. Due to the inclusion of attention in this mechanism, it can adaptively identify and reduce the impact of noise while retaining true signals related to aeroengine performance. Secondly, measurable parameter and reconstructed parameter as inputs to the model helps the model learn and consider the relationship between the two simultaneously, thereby better capturing the details and integrity of the aeroengine system, which can provide more comprehensive and rich information. Thirdly, due to the trained parameters already containing some level of knowledge, the parameters of the previously trained model are used as the initial parameters for the next to accelerate the convergence speed. Finally, three datasets are used for experimental verification to assess the effectiveness of the proposed method. The experimental results demonstrate that the proposed method exhibits good reconstruction performance. The relative error mean on aeroengine dataset is below 1.10%. The maximum relative error is below 5.44%.
更多查看译文
关键词
Aeroengine,Performance space,Sequence correlation,Reconstruction model,Unsupervised learning
AI 理解论文
溯源树
样例
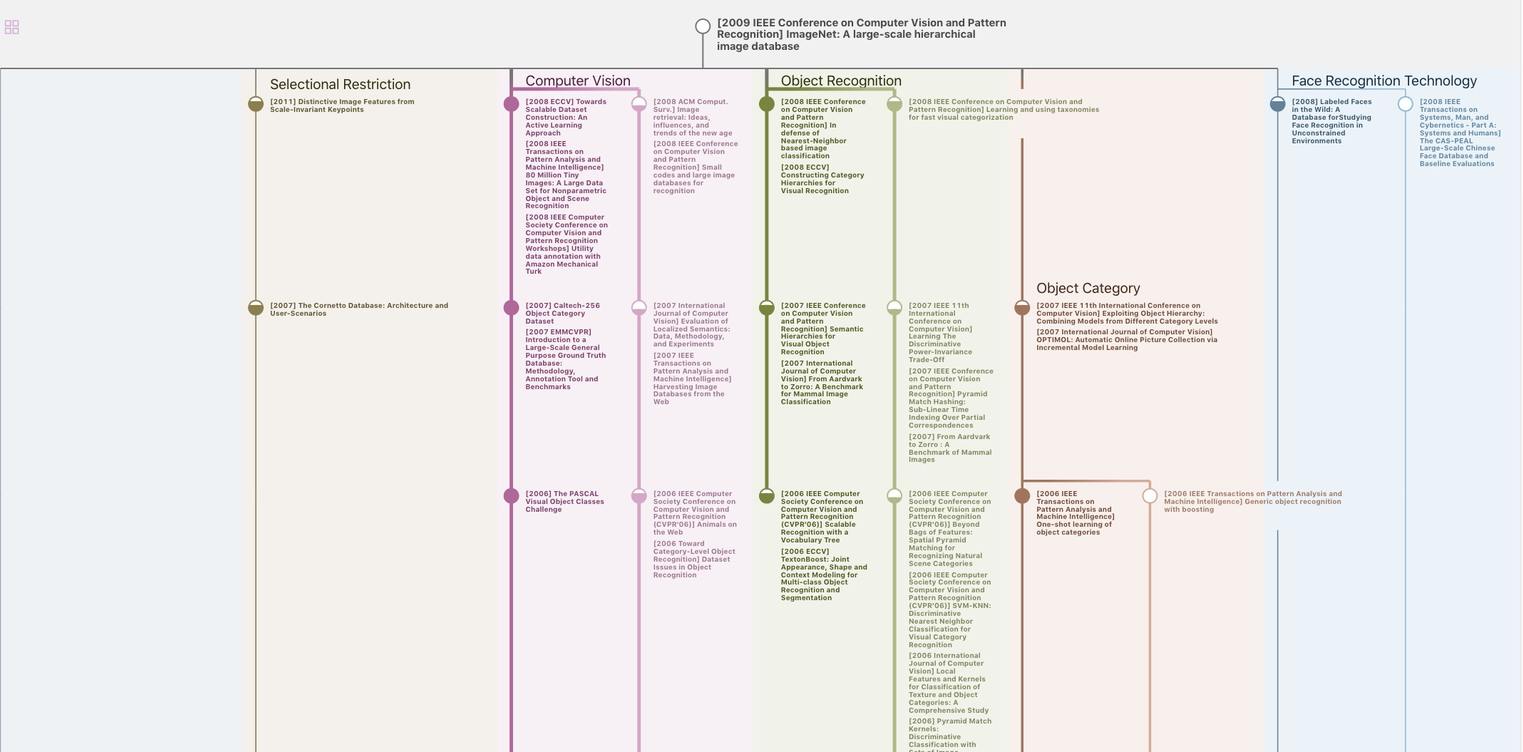
生成溯源树,研究论文发展脉络
Chat Paper
正在生成论文摘要