A time series anomaly detection method based on series-parallel transformers with spatial and temporal association discrepancies
INFORMATION SCIENCES(2024)
摘要
Multivariate time series anomaly detection methods can discover malfunctions in a complex system by detecting anomalies in the monitoring data. Multivariate time series have complex temporal and spatial correlations. Existing methods that explicitly model both correlations only extract semantic information sequentially in a single temporal-spatial or spatial-temporal order. However, different orders have a significant influence on the method's performance. In addition, a static graph structure trained on normal data has difficulties capturing the change of correlations between features when anomalies occur, limiting the method's performance. This paper proposes the Spatial-Temporal Anomaly Transformer (STAT), which extracts semantic information comprehensively by combining both spatial-temporal and temporal-spatial orders. STAT uses the Temporal Anomaly Transformer and the Spatial Anomaly Transformer (SAT) to explicitly compute temporal and spatial association discrepancies to conduct anomaly detection. Since the topological structure is usually unknown, SAT adopts a trainable hypersphere to learn the prior-association between features on normal data and computes the distance between the attention weights and the center of the hypersphere as the spatial association discrepancy. AUC and two recently proposed evaluation metrics are used to compare STAT with various typical methods on public data sets, proving that our method can achieve state-of-the-art performance.
更多查看译文
关键词
Multivariate time series,Anomaly detection,Transformer,Temporal association discrepancy,Spatial association discrepancy
AI 理解论文
溯源树
样例
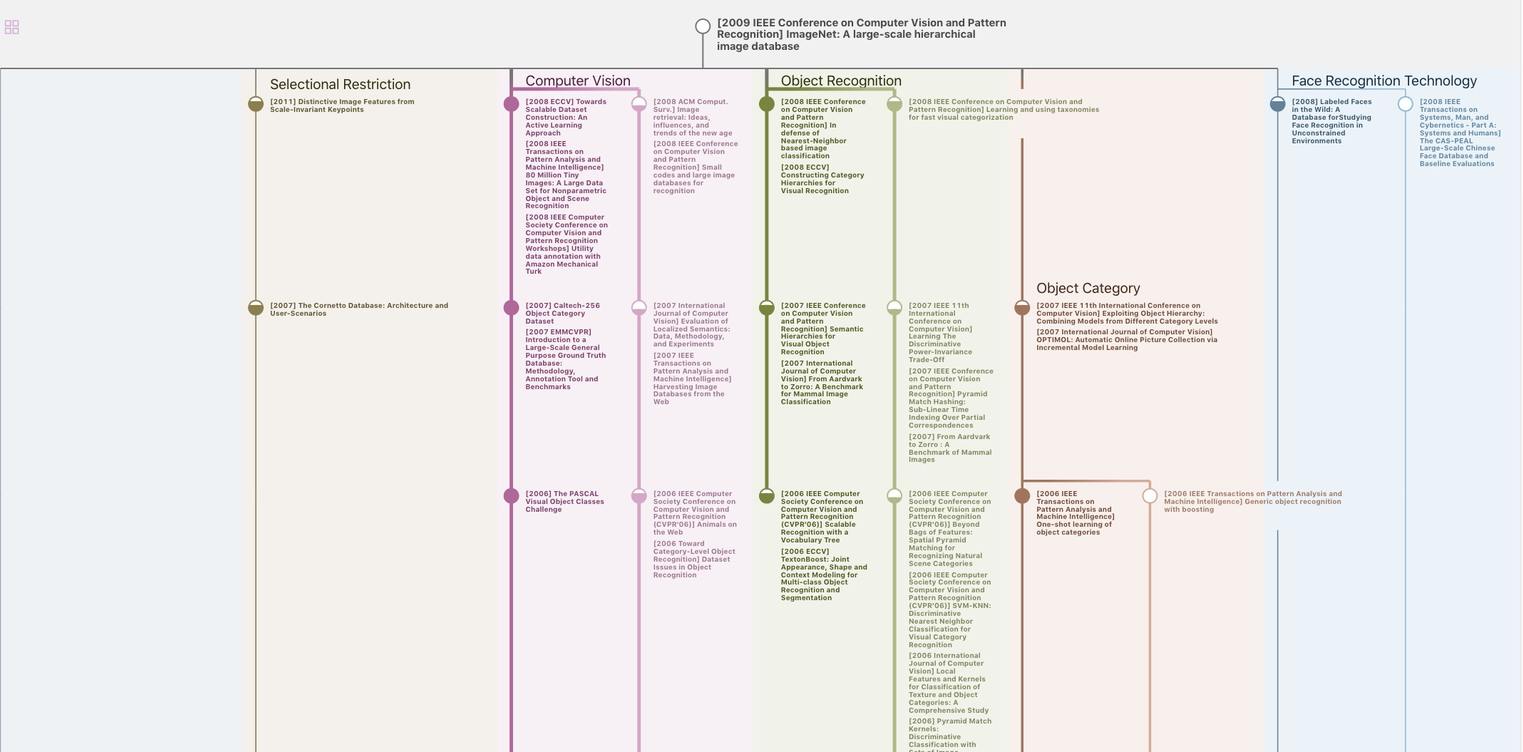
生成溯源树,研究论文发展脉络
Chat Paper
正在生成论文摘要