Enhancing Low-Resource NLP by Consistency Training With Data and Model Permutations
IEEE/ACM transactions on audio, speech, and language processing(2024)
摘要
Natural language processing (NLP) has recently shown significant progress in rich-resource scenarios. However, it is much less effective for low-resource scenarios due to the model easily overfitting to limited training data and generalizing poorly on testing data. In recent years, consistency training has been widely adopted and shown great promise in deep learning, but still remains unexplored in low-resource settings. In this work, we propose DM-CT, a framework that incorporates both data-level and model-level consistency training as well as advanced data augmentation techniques for low-resource scenarios. Concretely, the input data is first augmented, and the output distributions of different sub-models generated by model variance are forced to be consistent (model-level consistency). Meanwhile, the predictions of the original input and the augmented one are also constrained to be consistent (data-level consistency). Experiments on different low-resource NLP tasks, including neural machine translation (4 IWSLT14 translation tasks, multilingual translation task, and WMT16 Romanian $\rightarrow$ English translation), natural language understanding tasks (GLUE benchmark), and named entity recognition (Conll2003 and WikiGold), well demonstrate the superiority of DM-CT by obtaining significant and consistent performance improvements.
更多查看译文
关键词
consistency training,model permutations,low-resource
AI 理解论文
溯源树
样例
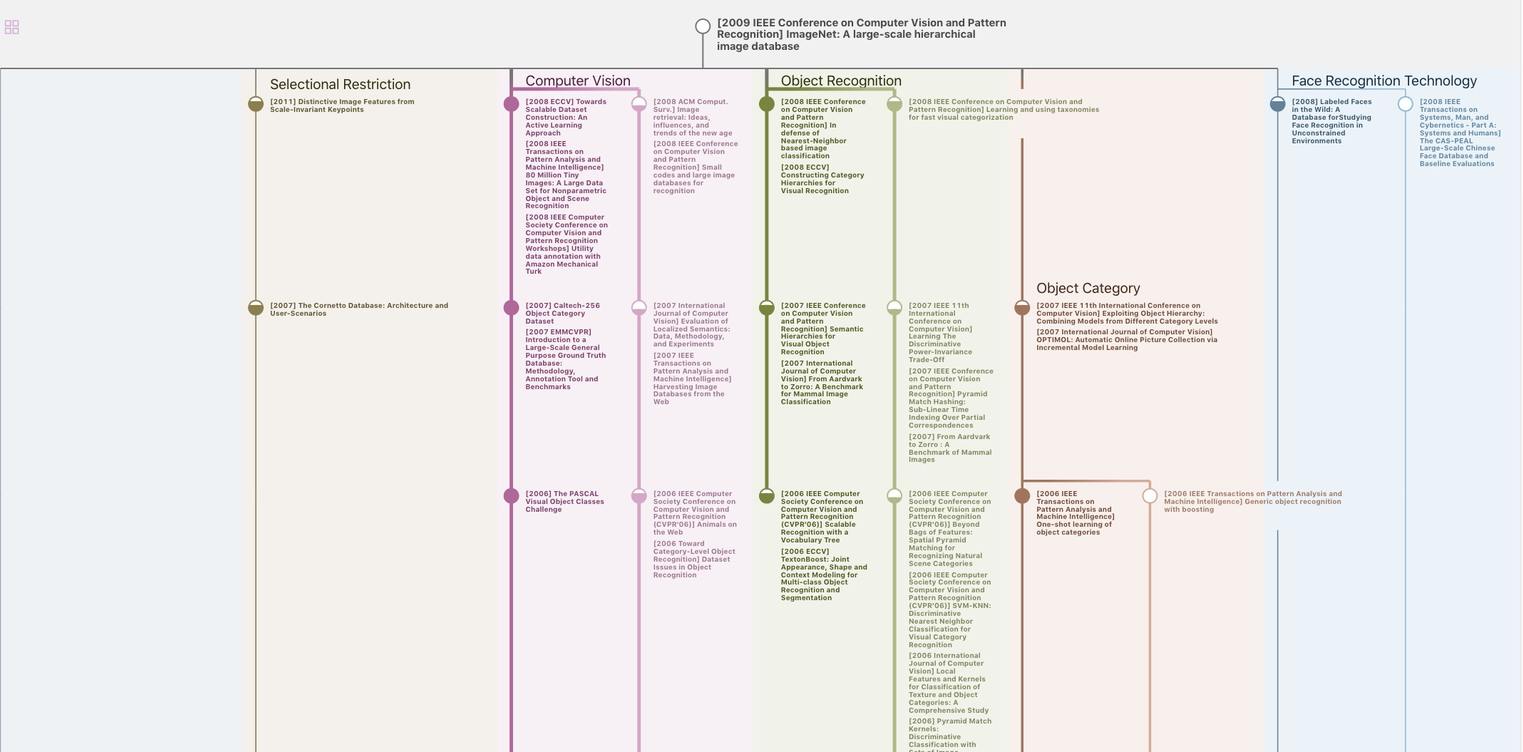
生成溯源树,研究论文发展脉络
Chat Paper
正在生成论文摘要