SSL^2: Self-Supervised Learning meets Semi-Supervised Learning: Multiple Sclerosis Segmentation in 7T-MRI from large-scale 3T-MRI
arXiv (Cornell University)(2023)
摘要
Automated segmentation of multiple sclerosis (MS) lesions from MRI scans is important to quantify disease progression. In recent years, convolutional neural networks (CNNs) have shown top performance for this task when a large amount of labeled data is available. However, the accuracy of CNNs suffers when dealing with few and/or sparsely labeled datasets. A potential solution is to leverage the information available in large public datasets in conjunction with a target dataset which only has limited labeled data. In this paper, we propose a training framework, SSL2 (self-supervised-semi-supervised), for multi-modality MS lesion segmentation with limited supervision. We adopt self-supervised learning to leverage the knowledge from large public 3T datasets to tackle the limitations of a small 7T target dataset. To leverage the information from unlabeled 7T data, we also evaluate state-of-the-art semi-supervised methods for other limited annotation settings, such as small labeled training size and sparse annotations. We use the shifted-window (Swin) transformer1 as our backbone network. The effectiveness of self-supervised and semi-supervised training strategies is evaluated in our in-house 7T MRI dataset. The results indicate that each strategy improves lesion segmentation for both limited training data size and for sparse labeling scenarios. The combined overall framework further improves the performance substantially compared to either of its components alone. Our proposed framework thus provides a promising solution for future data/label-hungry 7T MS studies.
更多查看译文
关键词
sclerosis segmentation,self-supervised,semi-supervised,t-mri,large-scale
AI 理解论文
溯源树
样例
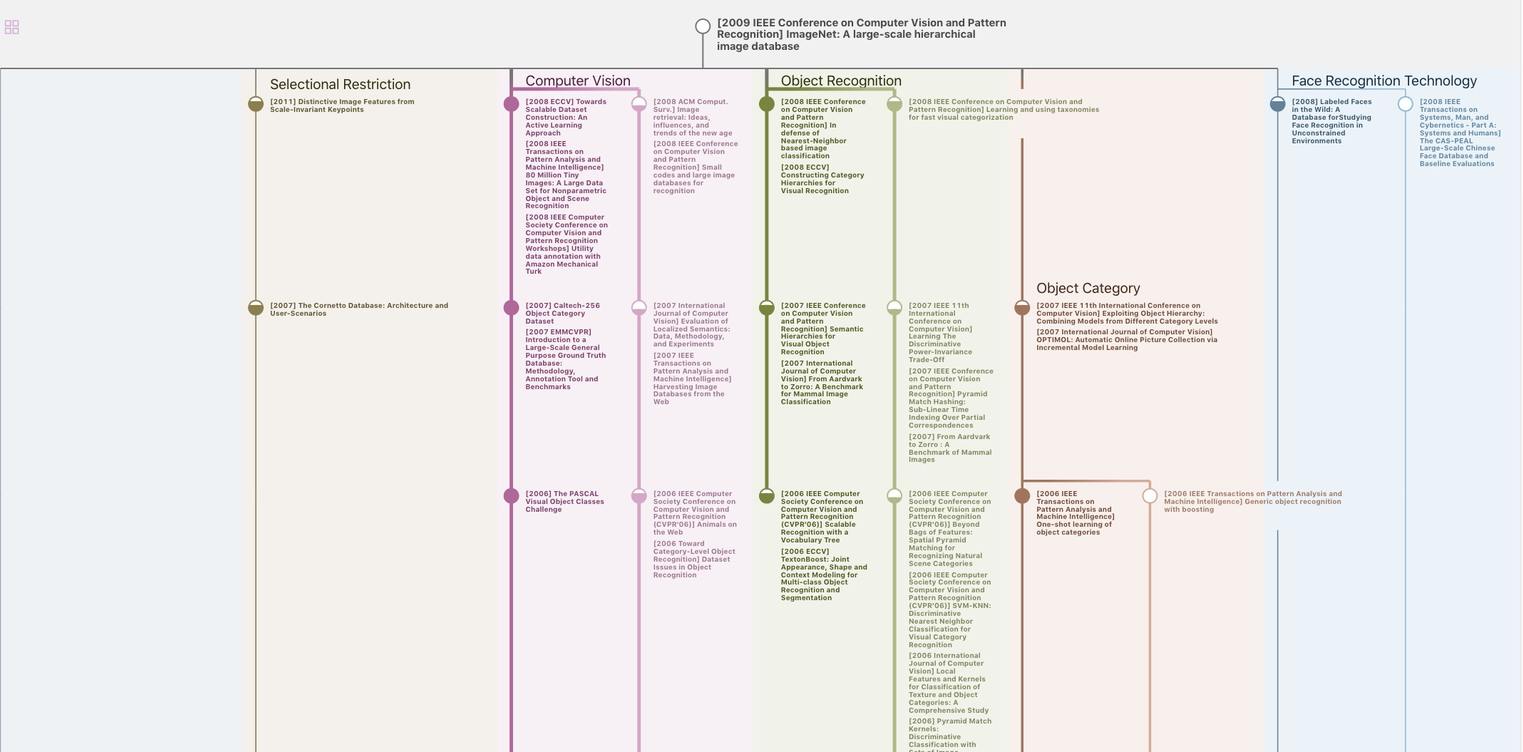
生成溯源树,研究论文发展脉络
Chat Paper
正在生成论文摘要