Enabling ImageNet-Scale Deep Learning on MCUs for Accurate and Efficient Inference
IEEE Internet of Things Journal(2023)
摘要
Conventional approaches to TinyML achieve high accuracy by deploying the largest deep learning model with highest input resolutions that fit within the size constraints imposed by the microcontroller’s (MCUs) fast internal storage and memory. In this paper, we perform an in-depth analysis of prior works to show that models derived within these constraints suffer from low accuracy and, surprisingly, high latency. We propose an alternative approach that enables the deployment of efficient models with low inference latency, but free from the constraints of internal memory. We take a holistic view of typical MCU architectures, and utilise plentiful but slower external memories to relax internal storage and memory constraints. To avoid the lower speed of external memory impacting inference latency, we build on the TinyOps inference framework, which performs operation partitioning and uses overlays via DMA, to accelerate the latency. Using insights from our study, we deploy efficient models from the TinyOps design space onto a range of embedded MCUs achieving record performance on TinyML ImageNet classification with up to 6.7% higher accuracy and 1.4x faster latency compared to state-of-the-art internal memory approaches.
更多查看译文
关键词
Edge AI,Internet of Things (IoT),Artificial Intelligence of Things (AIoT),TinyML,Microcontrollers,Embedded Systems,Machine Learning,Deep Learning,Neural Networks (NNs),Convolutional Neural Networks (CNNs)
AI 理解论文
溯源树
样例
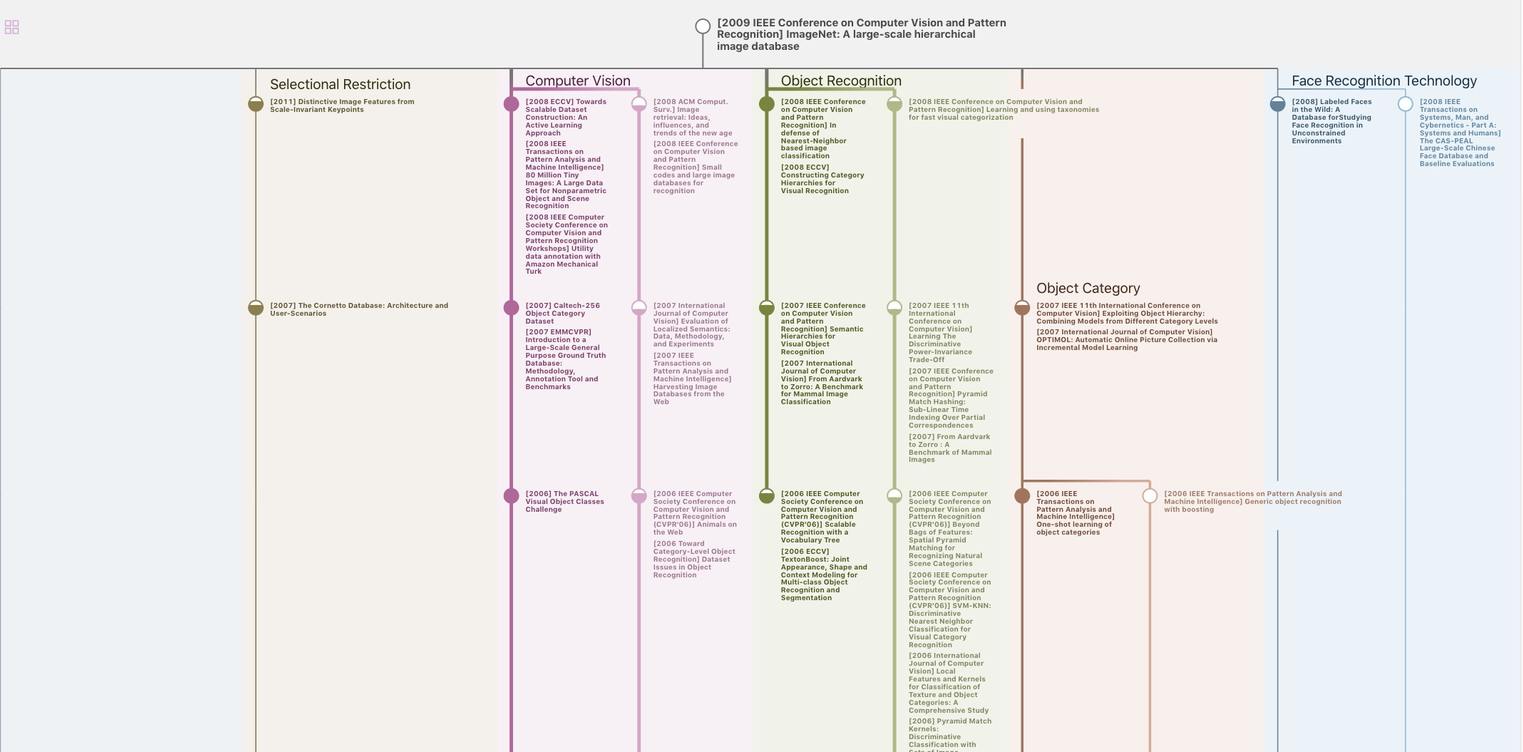
生成溯源树,研究论文发展脉络
Chat Paper
正在生成论文摘要