A Novel Data-driven Adaptive Robust Filter based on TCN-ACKF Method for Autonomous Train Localization System
Proceedings of the Satellite Division's International Technical Meeting(2023)
摘要
Satellite positioning technology plays a significant role in the next-generation train operation control system, which contributes to the establishment of an autonomous railway transportation system with reduced operation and maintenance costs. The quality of GNSS (Global Navigation Satellite System) satellite signal is not always sufficient for accurate and reliable positioning in complex railway environments. Uncertain measurement noise and faults may result in significant performance degradation. To address these issues, an adaptive robust Cubature Kalman filter (ACKF) method is proposed based on the Temporal Convolutional Network (TCN). An adaptive measurement noise covariance update module and a fault detection module are designed under the novel TCN-ACKF scheme. The adaptive measurement noise covariance is calculated with the pseudo-range error predicted by the TCN. The fault detection module is employed to determine whether there is a fault and carry out the specific measurement compensation when fault occurrence is identified. Simulations are conducted to evaluate the proposed method under a challenging GNSS environment via field data collected on the Lhasa-Rikaze Railway. Different scenarios, with both the step fault and time-varying noise, are investigated to illustrate the performance of the proposed filter. Experimental results demonstrate that the proposed method achieves robust state estimation against uncertain measurement noise and fault, significantly enhancing the positioning accuracy and robustness of the autonomous train positioning system.
更多查看译文
关键词
adaptive robust filter,data-driven,tcn-ackf
AI 理解论文
溯源树
样例
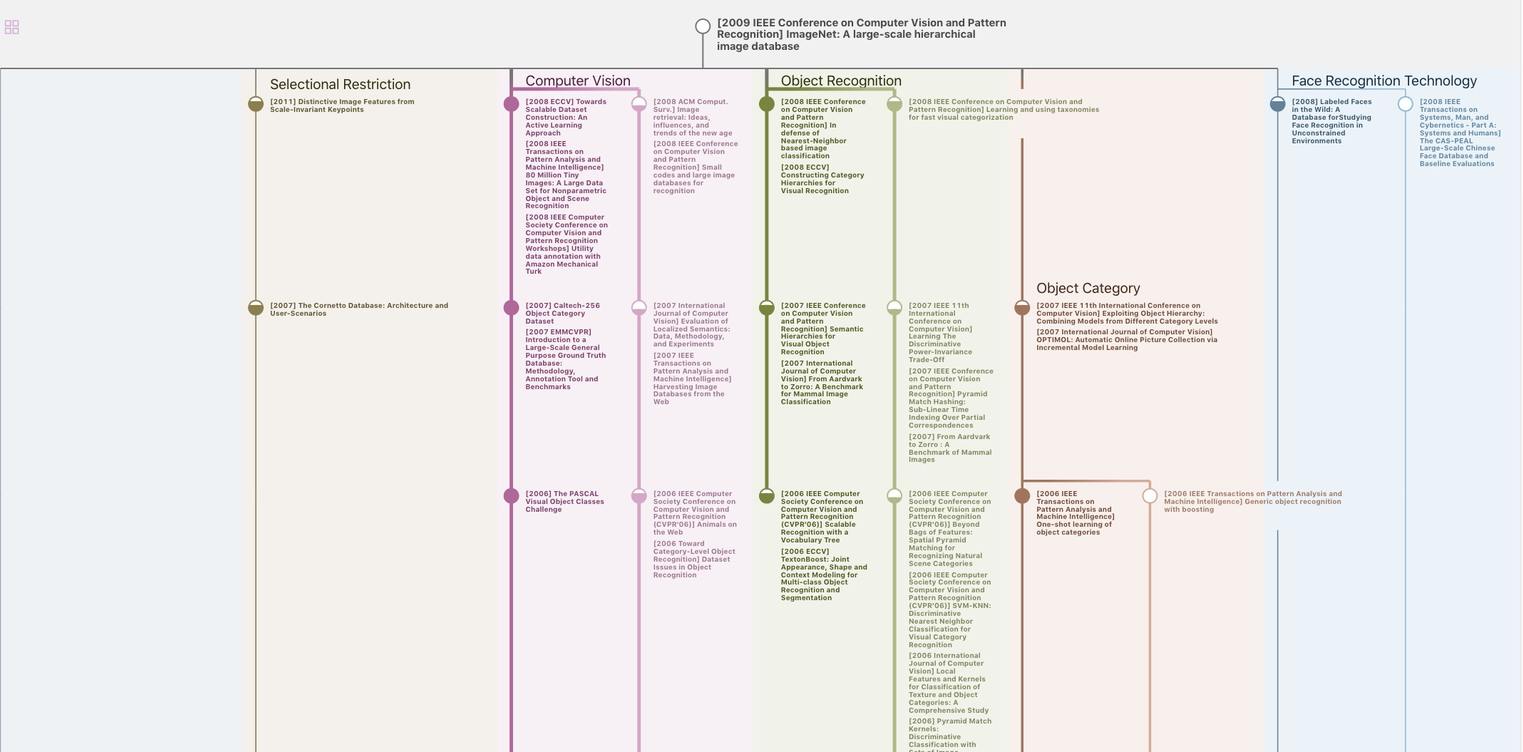
生成溯源树,研究论文发展脉络
Chat Paper
正在生成论文摘要