The Effect of Temporal Correlations on State Estimation Through Variational Bayesian Inference
Conference proceedings of the Society for Experimental Mechanics(2023)
摘要
Effective health monitoring in dynamic systems hinges on the proper estimation of the system’s state. As one of the most powerful methods of state estimation, variational Bayesian inference provides a flexible framework for making probabilistic inference about the state of a system. This method approximates the latent variables’ posteriors by minimizing the evidence lower bound (ELBO) loss function, which is an estimate of the information lost by approximating the true posterior with the selected variational family. This chapter focuses on the flexibility of the variational family and investigates the effect of considering dependency between the latent variables on the quality of posteriors, with respect to applications in structural health monitoring. The most common assumption made for the variational family is mean-field Gaussian, restricting the posterior’s space by independent Gaussian distributions. As this space does not realistically represent the relation between the dynamic system’s parameters, in this study, temporal correlations are added to the family’s covariance matrix structure. To evaluate the effect of the temporal off-diagonal covariance matrix on the performance of the inference, a numerical simulation of a linear single-degree-of-freedom system is utilized. The results of employing this covariance structure in the variational family are discussed from the accuracy, robustness, and computational cost standpoints. The findings show that for this linear system, the estimates of the parameters would not be meaningfully enhanced by adding the temporal dependency to the mean-field structure of the variational family.
更多查看译文
关键词
variational bayesian inference,state estimation,temporal correlations,bayesian inference
AI 理解论文
溯源树
样例
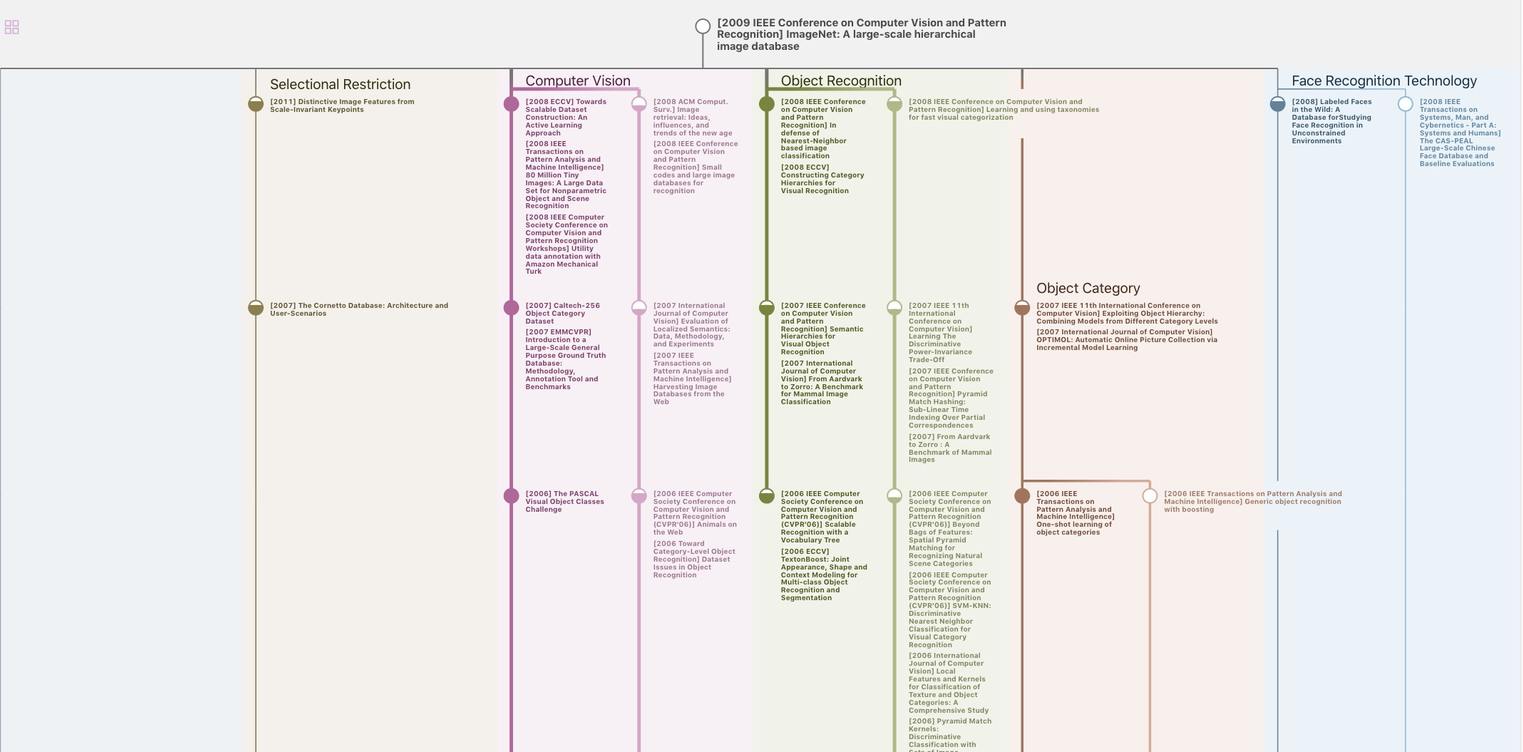
生成溯源树,研究论文发展脉络
Chat Paper
正在生成论文摘要