Spatio-Temporal Deep Graph Network for Event Detection, Localization and Classification in Cyber-Physical Electric Distribution System
IEEE TRANSACTIONS ON INDUSTRIAL INFORMATICS(2023)
摘要
This work proposes a deep graph learning framework to identify, locate, and classify power, cyber, and cyber power events at the distribution system level. The proposed algorithm jointly exploits spatial, temporal, and node-level cyber and physical data features. The developed graph neural network, together with a deep autoencoder, utilizes physical measurements from distribution level phasor measurement units and cyber data from communication network logs. The spatial structure of the synchrophasor measurements and network is incorporated through a weighted adjacency matrix. The temporal structure is incorporated by defining a spatial operation in the gated recurrent unit. This spatio-temporal learning element resides inside a power event detection, localization and classification module that provides degree of confidence for an event label. To accurately pinpoint the location of an event to the nearest bus equipped with a measurement unit, a combination of squared error and proximity score is utilized. Also included is a cyber event detection module that employs heteroskedasticity to analyze the significance of various cyber features during different types of attacks. Lastly, a dual-bit cyber-power decision table determines the nature of the event. The proposed method is validated on two distribution systems modeled in OPAL-RT/Hypersim with limited phasor measurement units for different possible physical and cyber events. Further analyses include comparison with other state-of-the-art methods and validation in the presence of measurement noise. Our method outperforms existing approaches and achieves an average detection accuracy of 97.97%, F1-score of 96.88%, precision of 96.53% and recall of 98.57%.
更多查看译文
关键词
autoencoder,cyber-physical attack,deep learning,distribution phasor measurement unit,graph neural network
AI 理解论文
溯源树
样例
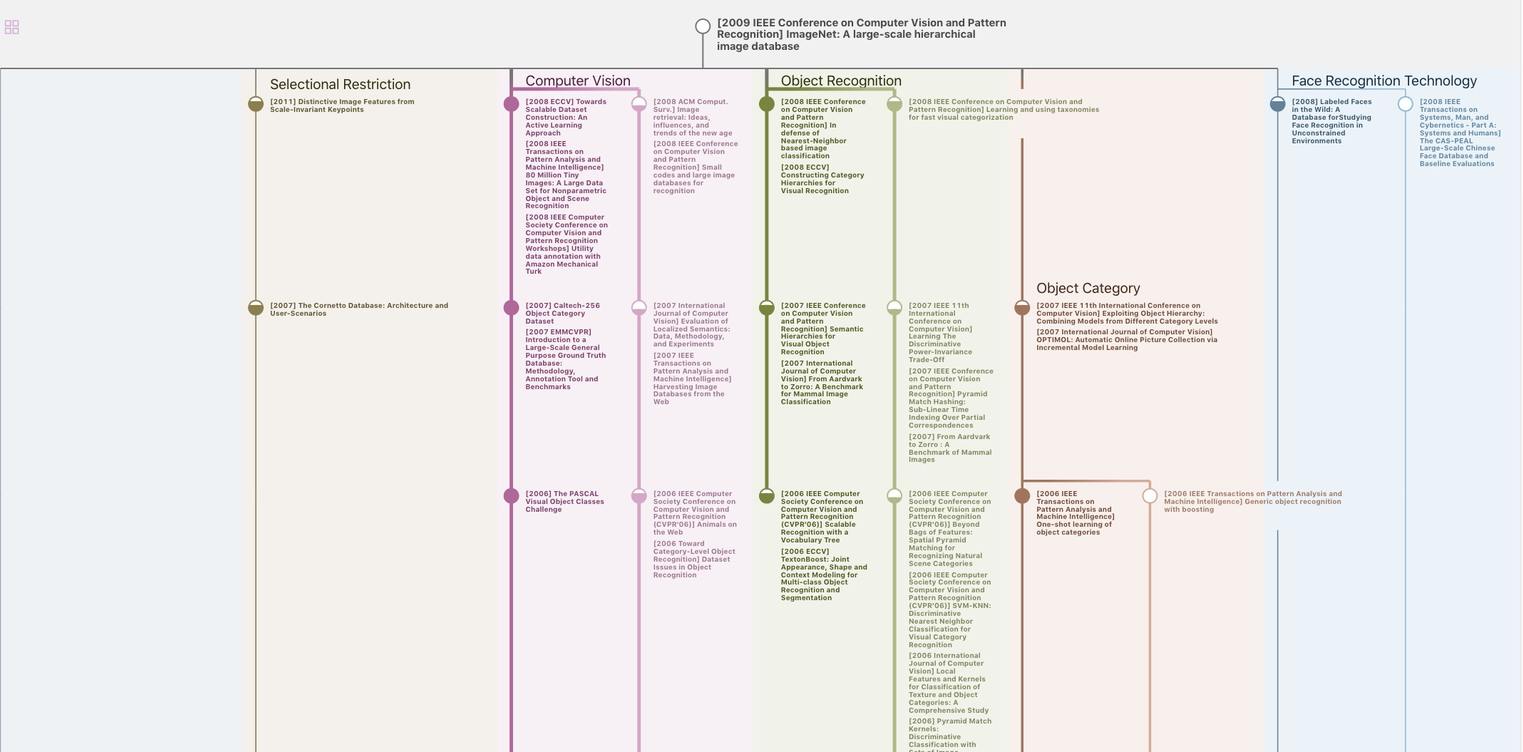
生成溯源树,研究论文发展脉络
Chat Paper
正在生成论文摘要