Evidence Reasoning and Curriculum Learning for Document-Level Relation Extraction
IEEE TRANSACTIONS ON KNOWLEDGE AND DATA ENGINEERING(2024)
摘要
Document-level Relation Extraction (RE) is a promising task aiming at identifying relations of multiple entity pairs in a document. Compared with the sentence-level counterpart, it has raised two significant challenges: a) In most cases, a relational fact can be adequately expressed via a small subset of sentences from the document, namely evidence. But the traditional method cannot model such strong semantic correlations between evidence sentences that collaborate to describe a specific relation; b) The data of this task is extremely long-tail in terms of too many NA instances and imbalanced relational types. Such data can mislead the tail prediction bias to the head categories in the RE model. In this paper, we present a novel E vidence reasoning and C urriculum learning method for D ocRE (DRE-EC) to address these challenges. Particularly, we first formulate evidence extraction as a sequential decision problem through a crafted reinforcement learning mechanism with an efficient path searching strategy to reduce the action space. Providing the evidence for each entity pair as a customized-filtered document in advance helps infer the relations better. To address the long-tail issue, we further develop a hybrid curriculum learning method at the NA-level (NC) and relation-level (RC) with our customized difficulty measure score. In NC, the NA samples are scheduled in an easy-to-hard scheme and gradually added, resulting in the data distribution from ideal and balanced to real and unbalanced. In RC, the scheme is switched into hard-to-easy to enhance the hard and tail samples. In addition, we propose a new Equalization adaptive Focal Loss(EFLoss) that can adjust to the changing data distribution and focus more on the tail categories. We conduct various experiments on two document-level RE benchmarks and achieve a remarkable improvement over previous competitive baselines. Furthermore, we provide detailed analyses of the advantages and effectiveness of our method.
更多查看译文
关键词
Curriculum learning,document-level relation extraction,evidence extraction,long-tail problem
AI 理解论文
溯源树
样例
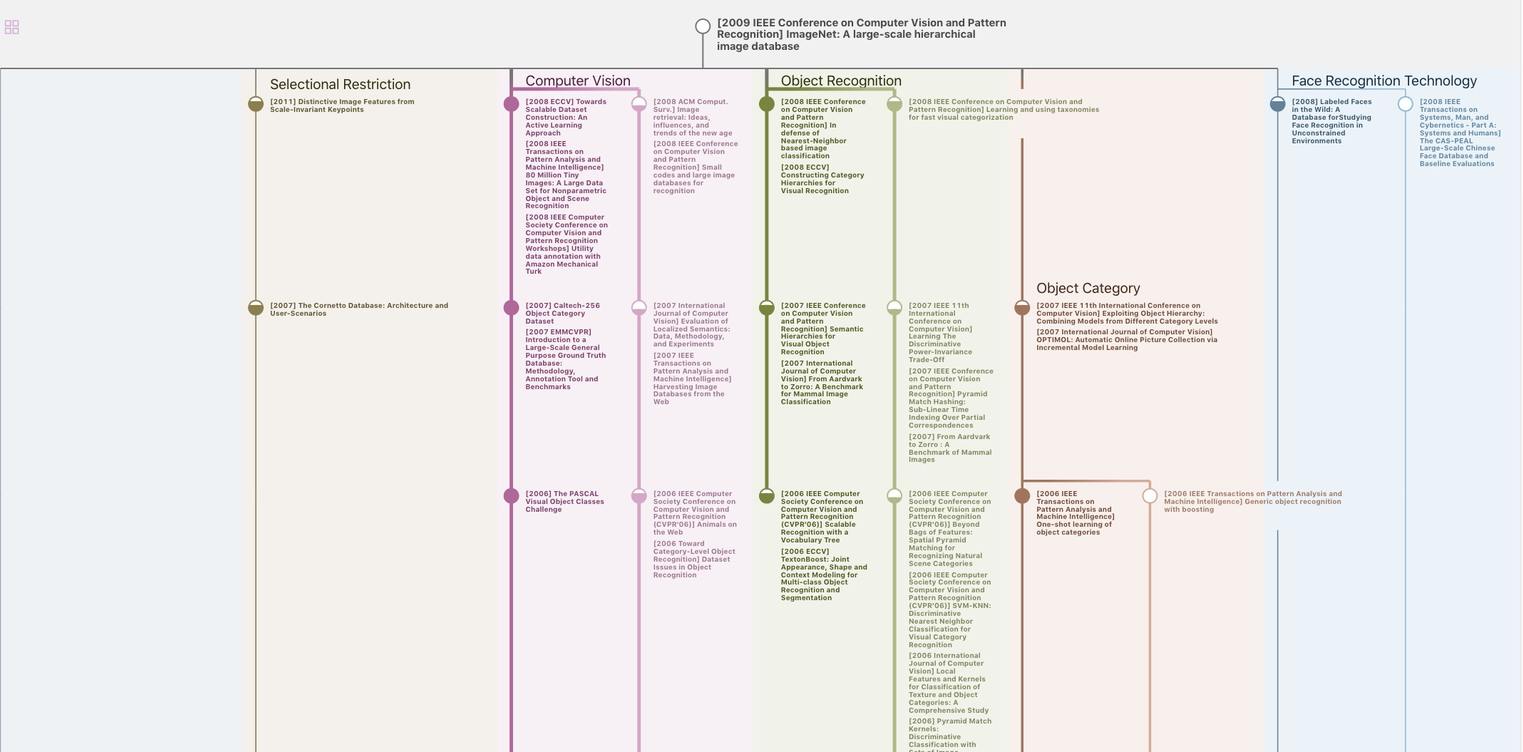
生成溯源树,研究论文发展脉络
Chat Paper
正在生成论文摘要