A Mini-batch Stochastic Recursive Gradient Method with Barzilai-Borwein Step-Size for Machine Learning
Research Square (Research Square)(2023)
摘要
Abstract As a mini-batch version of the SARAH algorithm, the MB-SARAH algorithm has received extensive attention due to its simple recursive scheme for updating stochastic gradient estimates. In this paper, we give a modification of the MB-SARAH method via cooperating with the BB step-size, shorted to MB-SARAH-BB. The MB-SARAH-BB combines some advantages of both MB-SARAH and BB methods, providing robustness in selecting initial step size during the optimization process. In the framework of MB-SARAH-BB, we propose a novel implementable method, Ada-MB-SARAH-BB, which utilizes adaptive probability for sampling in the mini-batch stochastic recursive gradient computation during the inner loop iteration. We establish the linear convergence of the MB-SARAH-BB and Ada-MB-SARAH-BB methods under some mild assumptions. Numerical experiments on standard machine learning datasets demonstrate that, the MB-SARAH-BB is effective and more competitive than the recent successful stochastic gradient methods. In addition, numerical experiments also demonstrate that the performance of Ada-MB-SARAH-BB is generally better than or comparable to MB-SARAH-BB method. MSC Classification: 90C15 · 90C25 · 90C30
更多查看译文
关键词
machine learning,mini-batch,barzilai-borwein,step-size
AI 理解论文
溯源树
样例
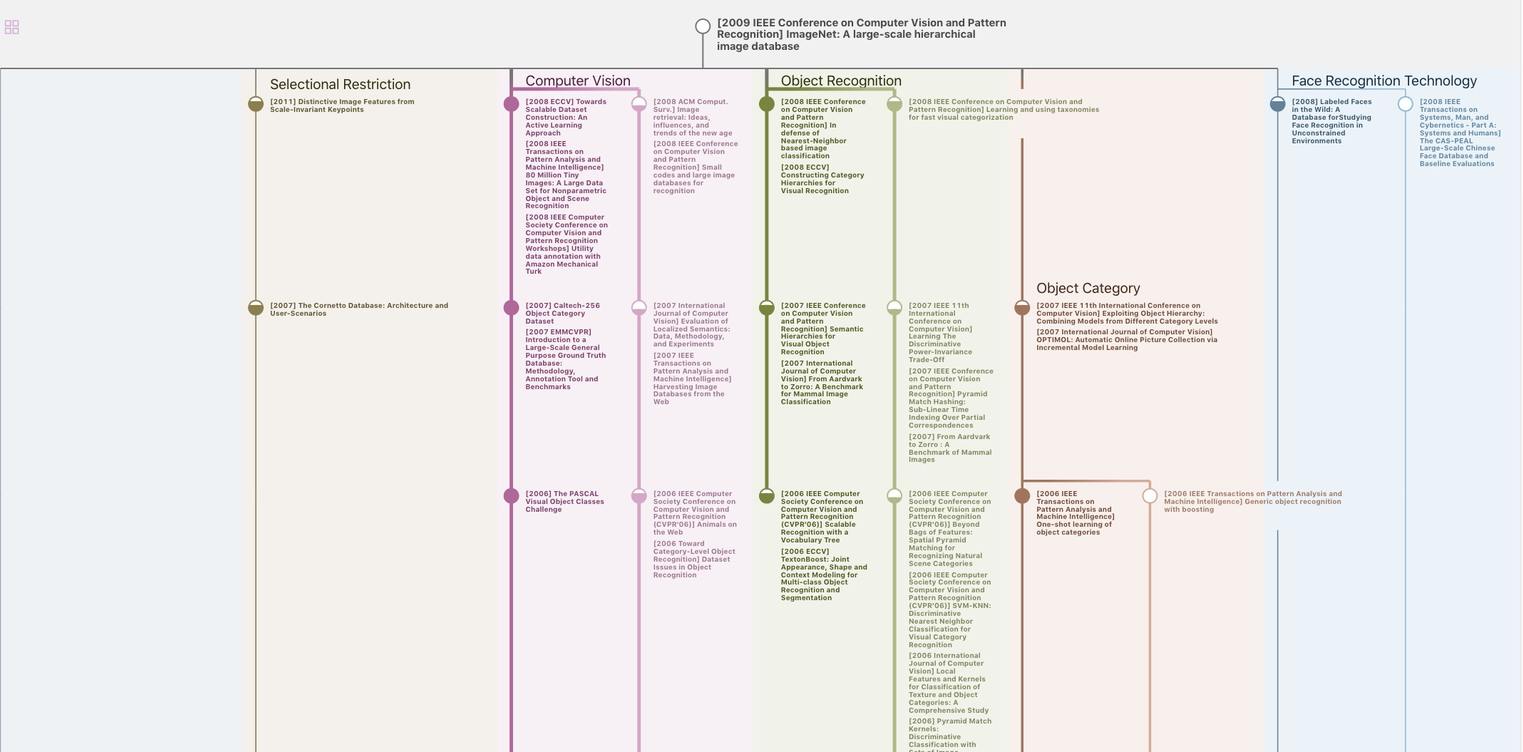
生成溯源树,研究论文发展脉络
Chat Paper
正在生成论文摘要