Semi-Supervised Domain Adaptation for Major Depressive Disorder Detection.
IEEE Trans. Multim.(2024)
摘要
Major Depressive Disorder (MDD) detection with cross-domain datasets is a crucial yet challenging application due to the
data scarcity
and
isolated data island
issues in multimedia computing research. Given the domain shift issue in MDD datasets and a continuous stream of incoming data in clinical settings, Semi-supervised Domain Adaptation (SDA) is suitable for addressing these challenges in MDD detection. However, existing mainstream Domain Adaptation (DA) methods have the following limitations that still need to be addressed, such as semantic misalignment, challenges in extending to various DA paradigms, and difficulty in addressing the classifier bias caused by class imbalance issues. To relieve the above issues, we propose a flexible
G
raph
N
eural
N
etwork-based
S
emi-supervised
D
omain
A
daptation (GNN-SDA) for MDD detection. The proposed framework comprises a feature extraction backbone along with two essential modules: a GNN-based domain alignment module and an uncertainty-guided optimization module. The GNN-based domain alignment module is designed to reduce the domain gap in a flexible manner, which is able to align multiple domains through the information propagation mechanism instead of the explicit alignment operation. The uncertainty-guided optimization module discusses the uncertainty of pseudo-labels, mitigating the adverse impact of noisy predictions and taking into account the class distribution of unlabeled data. Finally, we evaluate the proposed GNN-SDA framework for MDD detection under different domain adaptation paradigms on four benchmark datasets, i.e., DAIC-WOZ, EATD, CMDC, and MODMA. The promising results indicate the flexibility and effectiveness of the proposed framework for MDD detection.
更多查看译文
关键词
Semi-supervised domain adaptation,MDD detection,graph neural network,pseudo-label optimization,class imbalance,uncertainty estimation
AI 理解论文
溯源树
样例
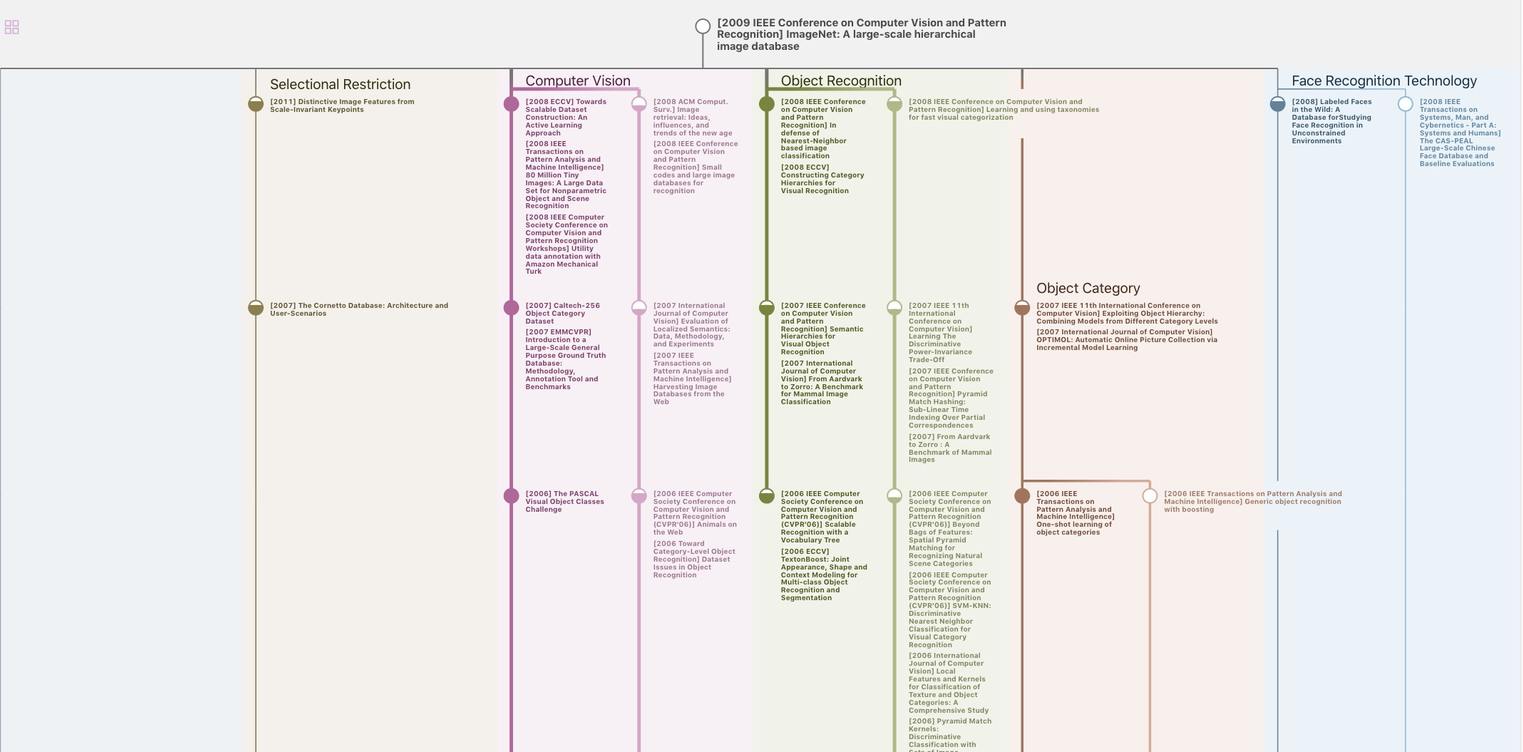
生成溯源树,研究论文发展脉络
Chat Paper
正在生成论文摘要