Self-Sampling Meta SAM: Enhancing Few-shot Medical Image Segmentation with Meta-Learning
arXiv (Cornell University)(2023)
摘要
While the Segment Anything Model (SAM) excels in semantic segmentation for general-purpose images, its performance significantly deteriorates when applied to medical images, primarily attributable to insufficient representation of medical images in its training dataset. Nonetheless, gathering comprehensive datasets and training models that are universally applicable is particularly challenging due to the long-tail problem common in medical images. To address this gap, here we present a Self-Sampling Meta SAM (SSM-SAM) framework for few-shot medical image segmentation. Our innovation lies in the design of three key modules: 1) An online fast gradient descent optimizer, further optimized by a meta-learner, which ensures swift and robust adaptation to new tasks. 2) A Self-Sampling module designed to provide well-aligned visual prompts for improved attention allocation; and 3) A robust attention-based decoder specifically designed for medical few-shot learning to capture relationship between different slices. Extensive experiments on a popular abdominal CT dataset and an MRI dataset demonstrate that the proposed method achieves significant improvements over state-of-the-art methods in few-shot segmentation, with an average improvements of 10.21% and 1.80% in terms of DSC, respectively. In conclusion, we present a novel approach for rapid online adaptation in interactive image segmentation, adapting to a new organ in just 0.83 minutes. Code is publicly available on GitHub upon acceptance.
更多查看译文
关键词
segmentation
AI 理解论文
溯源树
样例
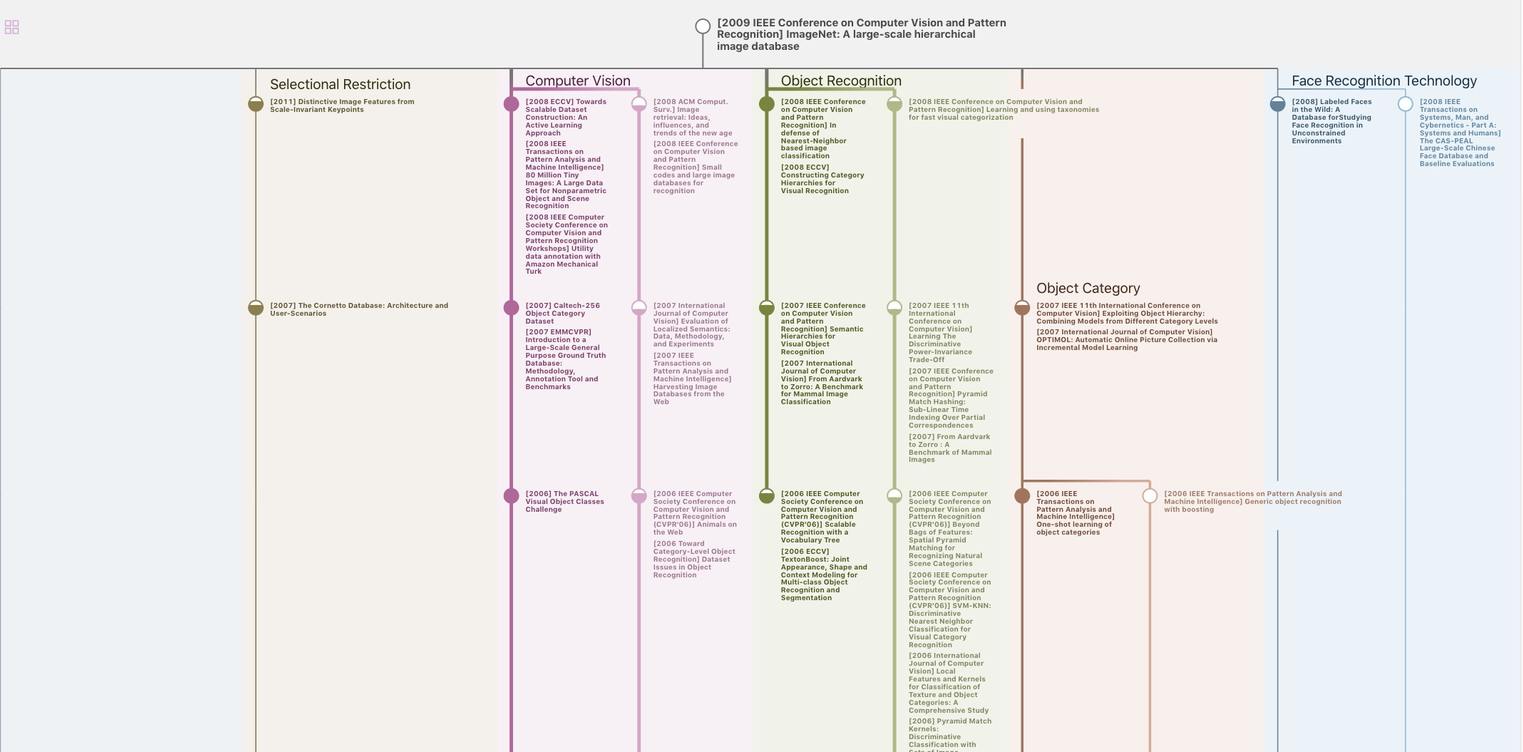
生成溯源树,研究论文发展脉络
Chat Paper
正在生成论文摘要