Understanding the high-dimensional nature of visual cortex representations
Journal of Vision(2023)
摘要
Most of the variance in cortical image representations is concentrated on a small set of latent dimensions that typically correspond to interpretable image properties like animacy, aspect ratio, and curvature. This has led to speculation that the cortex uses a low-dimensional code to represent natural images. However, this perspective neglects the long tail of low-variance dimensions that may be critical to human vision. We set out to determine if these dimensions contain meaningful visual information and whether deep neural networks (DNNs) can explain them. Using inter-participant comparisons, we quantified the reliable signal present in each dimension of the large-scale Natural Scenes fMRI dataset. Specifically, we used cross-validated ridge regression to predict the principal components (PCs) of one participant's neural responses using another participant's responses. We reasoned that if a dimension in one brain can be explained using independent neural responses in another brain, it must contain stimulus-related information. Our analysis revealed a strong power-law relationship between stimulus-related signal and PC rank that held across nearly four orders of magnitude, emphasizing that even extremely low-variance dimensions contain meaningful visual information. We also found that while DNNs successfully explained the first ten PCs of cortical responses, they diverged from human brain representations when examining higher-rank PCs. This suggests that current DNNs only match human brain representations in a coarse, low-dimensional subspace, perhaps explaining the longstanding gap between human and artificial vision. Our findings demonstrate the high-dimensional nature of the representational code in human visual cortex and reveal a long tail of stimulus-related information in cortical responses that DNNs have yet to explain.
更多查看译文
关键词
visual cortex,representations,high-dimensional
AI 理解论文
溯源树
样例
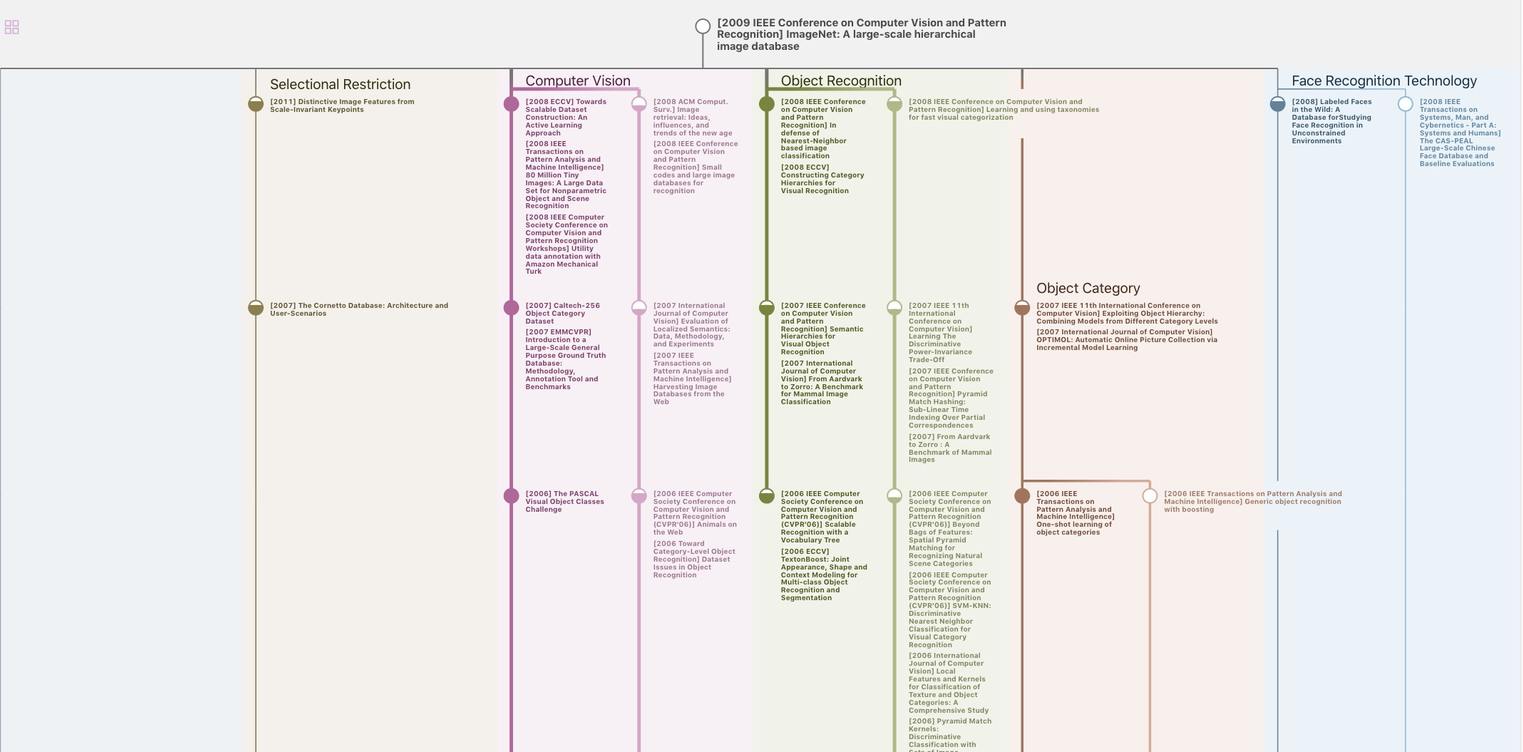
生成溯源树,研究论文发展脉络
Chat Paper
正在生成论文摘要