Embedding-Based First-Order Rule Learning in Large Knowledge Graphs
Frontiers in Artificial Intelligence and Applications(2023)
摘要
Numerous large knowledge graphs, such as DBpedia, Wikidata, Yago and Freebase, have been developed in the last decade, which contain millions of facts about various entities in the world. These knowledge graphs have proven to be incredibly useful for intelligent Web search, question understanding, in-context advertising, social media mining, and biomedicine. As some researchers have pointed out, a knowledge graph is not just a graph database, but it should have a layer of conceptual knowledge, which is usually represented as a set of first-order rules. However, it is challenging to automatically extract first-order rules from large knowledge graphs. In particular, traditional models are usually unable to handle rule learning in large knowledge graphs. This chapter aims to present state-of-the-art techniques and models on learning first-order rules using representation learning. By first recalling some basics of rule learning in knowledge graphs, we will introduce useful techniques of embedding-based rule learning through major models such as RLvLR and TyRuLe, which embed paths in knowledge graphs into latent spaces for candidate rule search. Then we evaluate the rule learning efficiency and the quality of automatically learned rules by applying them in link prediction. Before the chapter is concluded, we will also discuss some future research problems in the area.
更多查看译文
关键词
knowledge,rule,graphs,embedding-based,first-order
AI 理解论文
溯源树
样例
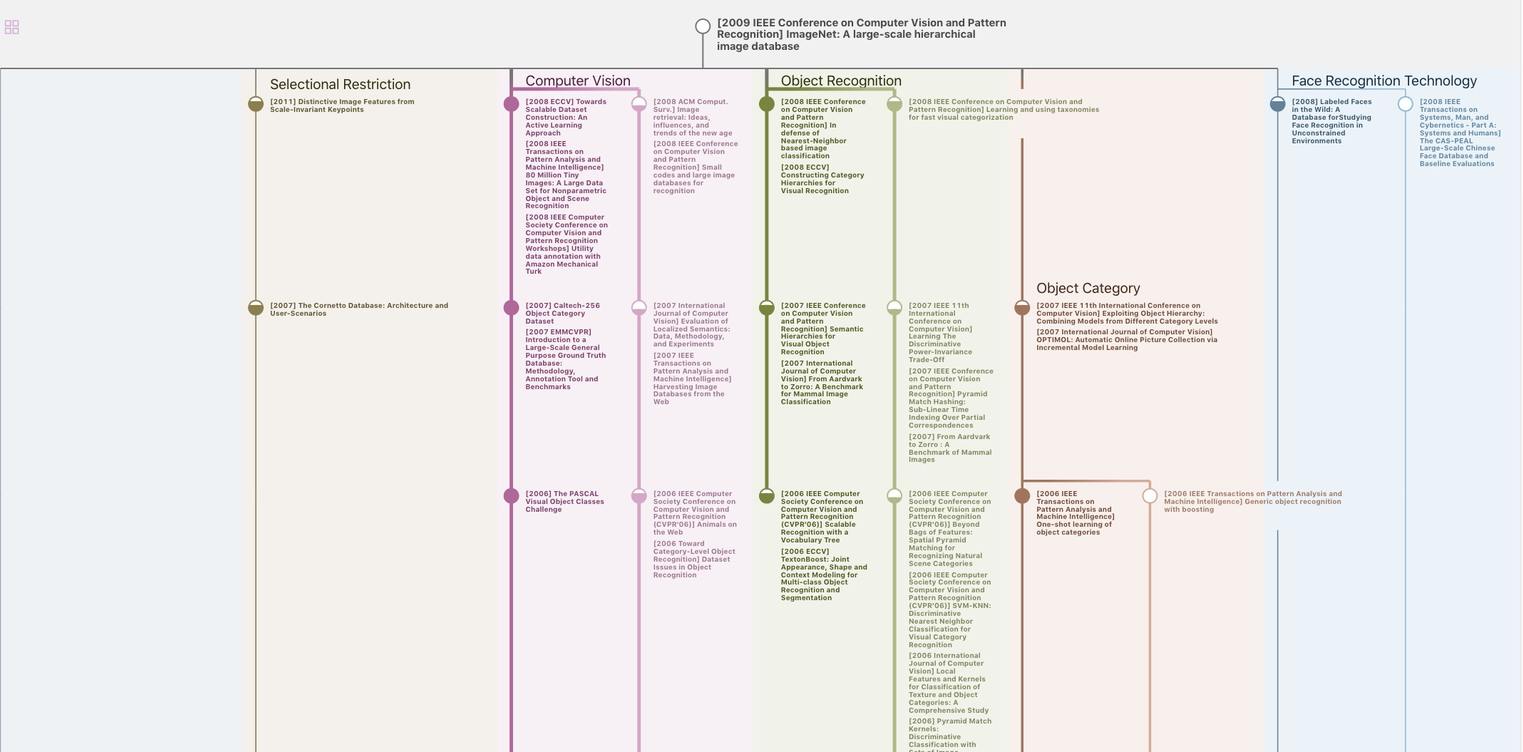
生成溯源树,研究论文发展脉络
Chat Paper
正在生成论文摘要