Transferability of Machine Learning Algorithm for IoT Device Profiling and Identification
IEEE INTERNET OF THINGS JOURNAL(2024)
摘要
The lack of appropriate cyber security measures deployed on Internet of Things (IoT) makes these devices prone to security issues. Consequently, the timely identification and detection of these compromised devices become crucial. Machine learning (ML) models which are used to monitor devices in a network have made tremendous strides. However, most of the research in profiling and identification uses the same data for training and testing. Hence, a slight change in the data renders most learning algorithms to work poorly. In this article, we study a transferability approach based on the concept of transductive transfer learning for IoT device profiling and identification. Notably, this type of transfer learning works by explicitly assigning labels to the test data in the target domain by using the test feature space in the target domain, with training data from the source domain. Specifically, we propose a three-component system comprising: 1) the device type identification; 2) the vulnerability assessment; and 3) the visualization module. The device type identification component uses the underlying concept of transductive transfer learning where the trained model is transferred to a remote lab for testing. A variety of ML models are evaluated with respect to accuracy, precision, recall, and F1-score in order to determine which are the most suitable for the proposed transferability profiling. Furthermore, the vulnerability of the predicted device type is also assessed by using three vulnerability databases: 1) Vulners; 2) National Vulnerability Database (NVD); and 3) IBM X-Force. Finally, the results from the vulnerability assessment are visualized and displayed on a dashboard.
更多查看译文
关键词
Internet of Things (IoT),machine learning (ML),security,transferability,visualization,vulnerability assessment
AI 理解论文
溯源树
样例
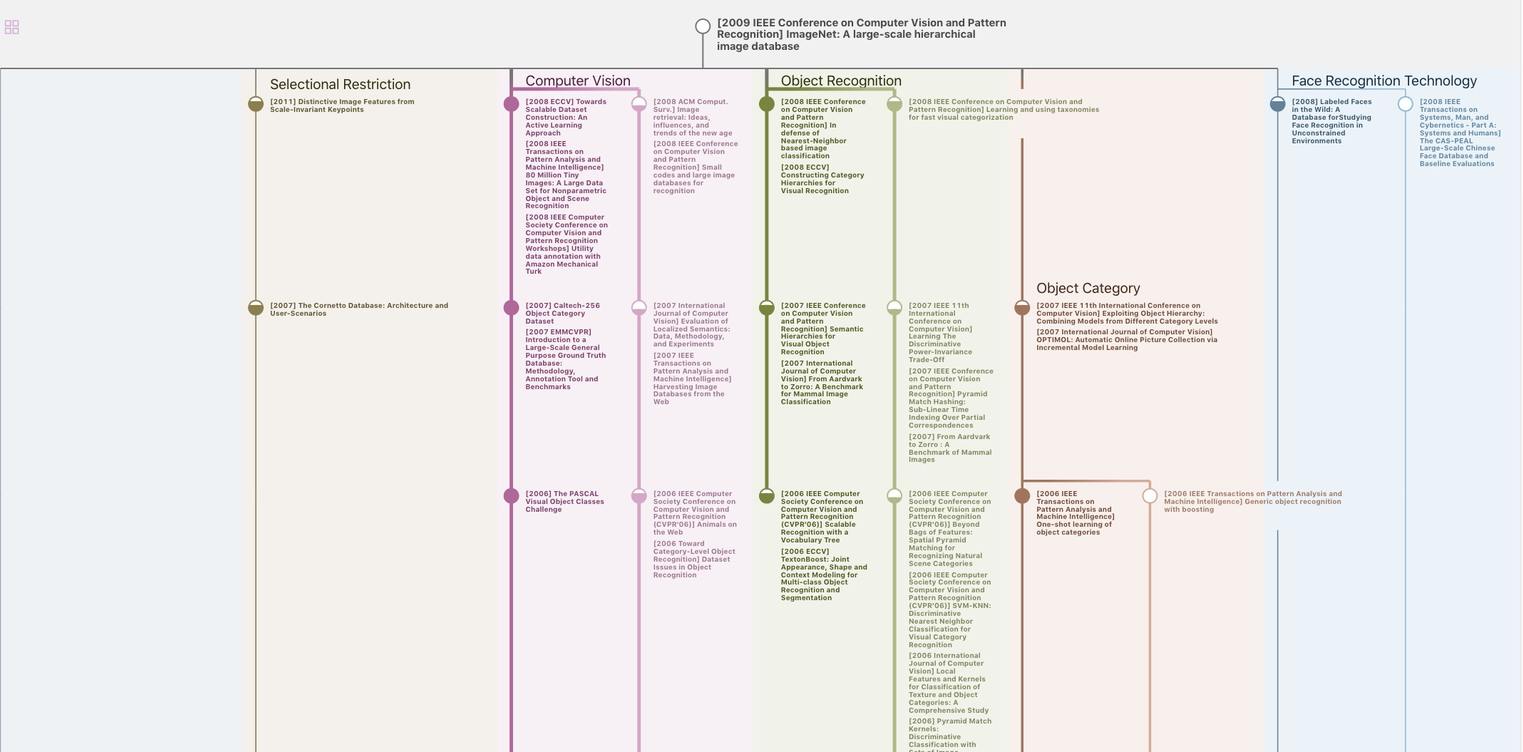
生成溯源树,研究论文发展脉络
Chat Paper
正在生成论文摘要