Generation of 2D-QSAR Models for Designing Potent Atovaquone Derivatives
Journal of Advance Research in Science and Social Science(2023)
摘要
Atovaquone belongs to the class of naphthalenes and is mainly an anti parasite drug which selectively targets malarial parasite.It is structurally hydrophobic in nature therefore having poor water solubility and oral bioavailability.Chemically it is composed of a napthoquinonyl head, a cyclohexanyl linker and a hydrophobic 4-chlorophenyl tail, which is responsible for the hydrophobic nature of this drug.Due to the presence of naphthoquinonyl head moiety O O OH Atovaquone shows antitumor activity.The 4-chlorophenyl tail and cyclohexanyl linker provides potential sites for structural optimization.Drug designing is a crucial step for obtaining novel drugs and requires potent methodologies.In this research work, QSAR analysis of Atovaquone derivatives was performed using a number of structural parameters.The values of different physicochemical parameters are evaluated and indicator parameter I1 for 4-F-3-Cl at R1,I2 for H at R3 position and I3 for CH groups at X position were used in present QSAR studies.In this work, the ratio PRESS/SSY for the equations 2, 17, 18, 41 and 42 are 0.190, 0.174, 0.190, 0.180, 0.179 respectively, which are less than 0.400, indicating that these models are reliable QSAR models.The PSE as well as SPRESS are good parameters to be used for discussing the uncertainty in prediction.The lower the value of these parameters, the better will be the predictive ability of the model.Also, the highest R 2 CV (0.826) has been found for the QSAR model.no.17 indicating that it has excellent overall predictive power.Thus from the results obtained it is clear that for getting the potent and efficient drug less Bulky groups having lesser vertices should be used, more polar, less electronegative group, more hydrophobic group is beneficial for the activity.Group H at R3 position and CH at position X should be retained at R2 position.The results are
更多查看译文
关键词
d-qsar
AI 理解论文
溯源树
样例
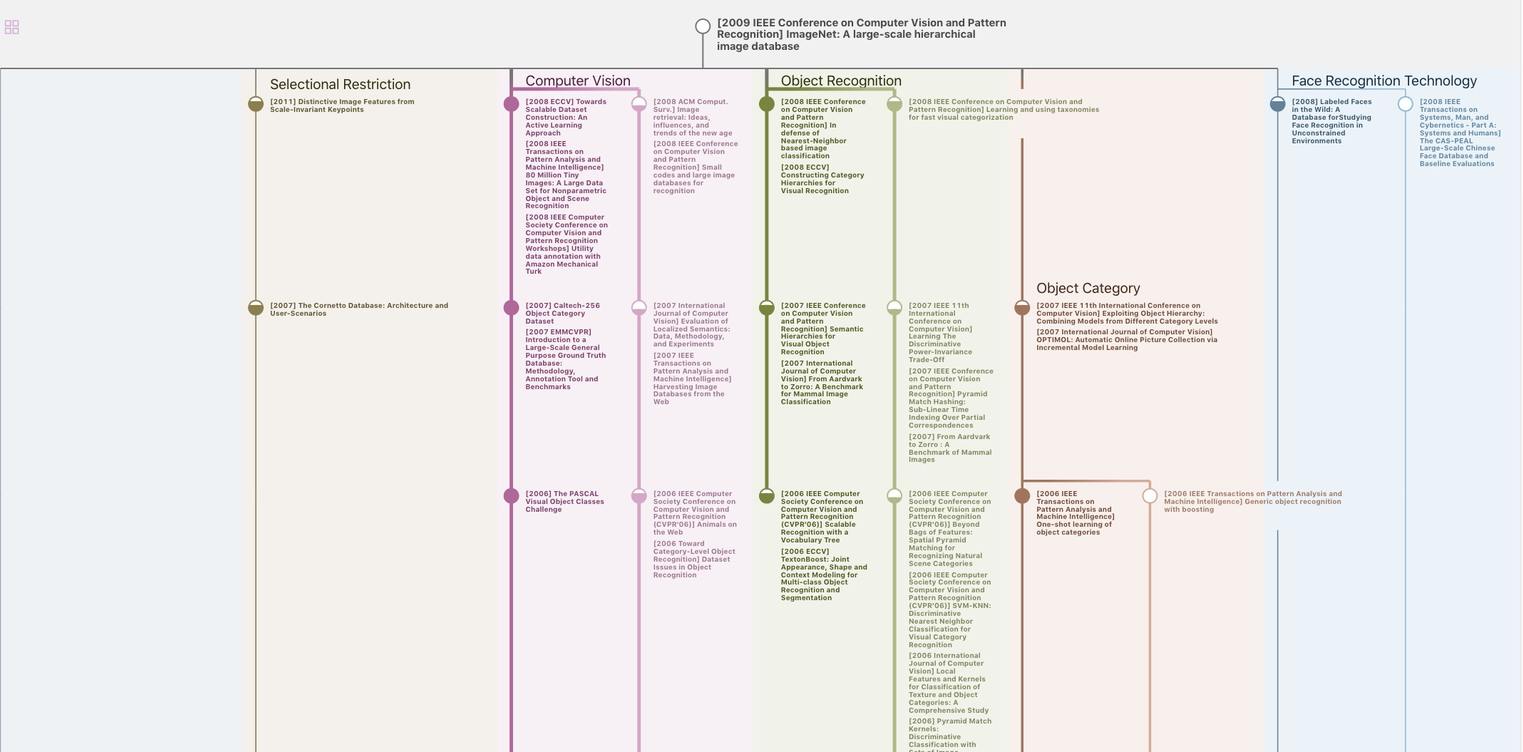
生成溯源树,研究论文发展脉络
Chat Paper
正在生成论文摘要