Probabilistic Graph Networks for Learning Physics Simulations
Research Square (Research Square)(2023)
摘要
Abstract Inductive biases play a critical role in enabling Graph Networks (GN) to learn particle and mesh-based physics simulations. In this paper, we propose two generalizable inductive biases that minimize rollout error and energy accumulation. Conditioned on the input states, GNs currently assume Gaussian distributed targets. As a consequence, GNs either assign probability densities to infeasible regions in the state space of the physics problem or fails to assign densities to feasible regions. Instead, we replace the existing assumption with a stronger assumption by estimating the true conditional target densities using our proposed conditional normalizing flows (cNF) decoder. We discover that this inductive bias enables GN to significantly improve its next state prediction accuracy. Existing sequential GNs encode temporal dependencies by autoregressively processing the latent representations of the input data. In our work, we discover that inducing the Arrow-of-Time inductive bias through an auto-regressive encoding step before autoregressively processing the resulting latent vectors enables GNs to better minimize rollout error. We critically analyze the impact of existing inductive biases on rollout error and energy accumulation and discover that the choice of biases to be encoded in a GN as opposed to the number of inductive biases has a substantial impact on forward simulation prediction.
更多查看译文
关键词
networks,simulations,graph,learning,physics
AI 理解论文
溯源树
样例
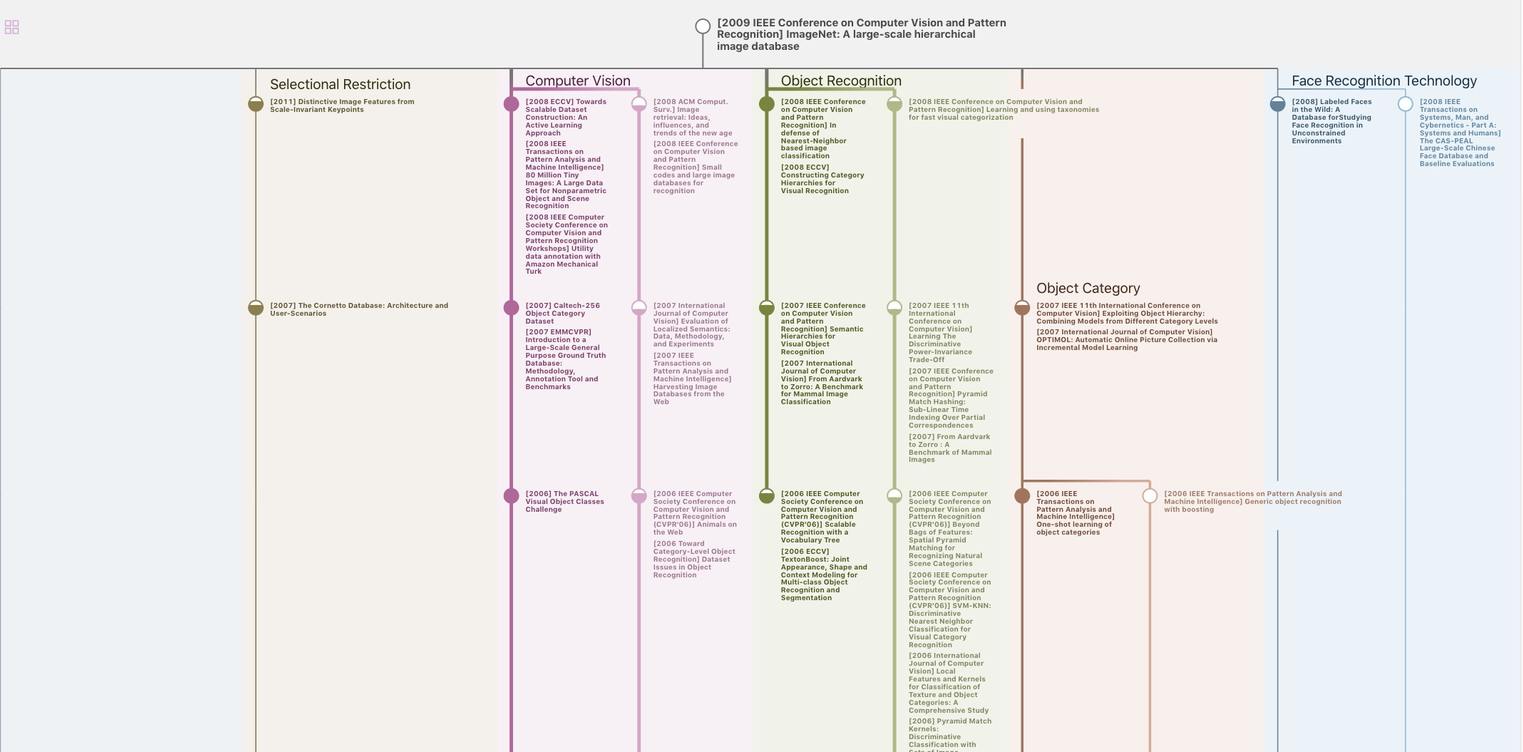
生成溯源树,研究论文发展脉络
Chat Paper
正在生成论文摘要