Unlocking the Potential of Spiking Neural Networks: Understanding the What, Why, and Where
IEEE Transactions on Cognitive and Developmental Systems(2023)
摘要
Spiking neural networks (SNNs) are a promising avenue for machine learning with superior energy efficiency compared to traditional artificial neural networks (ANNs). Recent advances in training and input encoding have put SNNs on par with state-of-the-art ANNs in image classification. However, such tasks do not utilize the internal dynamics of SNNs fully. Notably, a spiking neuron’s membrane potential acts as an internal memory, merging incoming inputs sequentially. This recurrent dynamic enables the networks to learn temporal correlations, making SNNs suitable for sequential learning. Such problems can also be tackled using ANNs. However, to capture the temporal dependencies, either the inputs have to be lumped over time (e.g. Transformers); or explicit recurrence needs to be introduced (e.g. recurrent neural networks (RNNs), long-short-term memory (LSTM) networks), which incurs considerable complexity. To that end, we explore the capabilities of SNNs in providing lightweight solutions to four sequential tasks involving text, speech and vision. Our results demonstrate that SNNs, by leveraging their intrinsic memory, can be an efficient alternative to RNNs and LSTMs for sequence processing, especially for certain edge applications. Furthermore, SNNs can be combined with ANNs (hybrid networks) synergistically to obtain the best of both worlds in terms of accuracy and efficiency.
更多查看译文
关键词
Neuromorphic computing,Spiking neural networks,Automatic speech recognition,Optical flow estimation
AI 理解论文
溯源树
样例
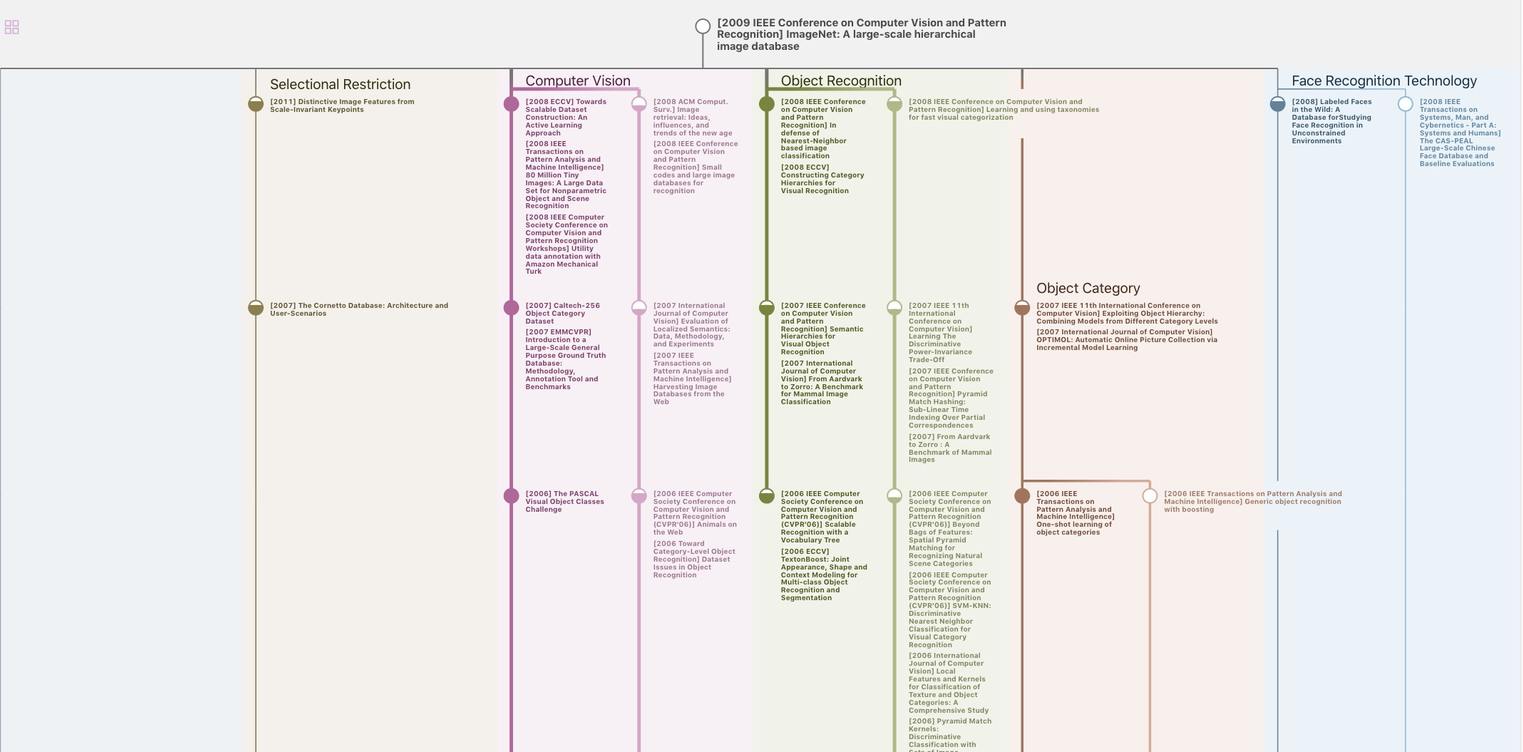
生成溯源树,研究论文发展脉络
Chat Paper
正在生成论文摘要