Benchmarking Compound Activity Prediction for Real-World Drug Discovery Applications
Research Square (Research Square)(2023)
摘要
Abstract Identifying active compounds for target proteins is one of the most fundamental objectives in early drug discovery. Recently, a growing number of computational methods, especially data-driven methods, have demonstrated promising potential in predicting compound activities. However, there lacks a well-designed benchmark to objectively evaluate these methods from the aspects of practical applications, which can consider the biased distribution of current real-world compound activity data and adopt appropriate evaluation metrics to avoid overestimation of model performances. In this paper, we propose a novel benchmark, named CARA, to systematically evaluate the performances of compound activity prediction models from a practical perspective. To eliminate the biases in current compound activity data, two types of assays, i.e., virtual screening (VS) and lead optimization (LO), were distinguished according to their drug discovery stages. New train-test splitting schemes and evaluation metrics including the assay-based evaluation and success rates were adopted to comprehensively measure the performances of different methods. Evaluation of several state-of-the-art models on our CARA benchmark demonstrated a more accurate, informative, and direct understanding of model performances than the commonly-used bulk evaluation. In addition, another popular application scenario, in which there were a few already measured task-related samples, was also considered by CARA. Evaluation of a number of few-shot training strategies demonstrated their different preferences for VS and LO tasks, further suggesting the necessity of distinguishing the two task types. A useful indicator was also discovered to estimate the confidence of model predictions on the experimentally unmeasured data. Overall, our CARA benchmark can provide a high-quality dataset for developing and evaluating compound activity prediction models, and the analysis results and new findings in this work can also act as useful guidance for drug discovery applications. New challenges and opportunities were also revealed and discussed for improving current data-driven methods.
更多查看译文
关键词
compound activity prediction,drug,real-world
AI 理解论文
溯源树
样例
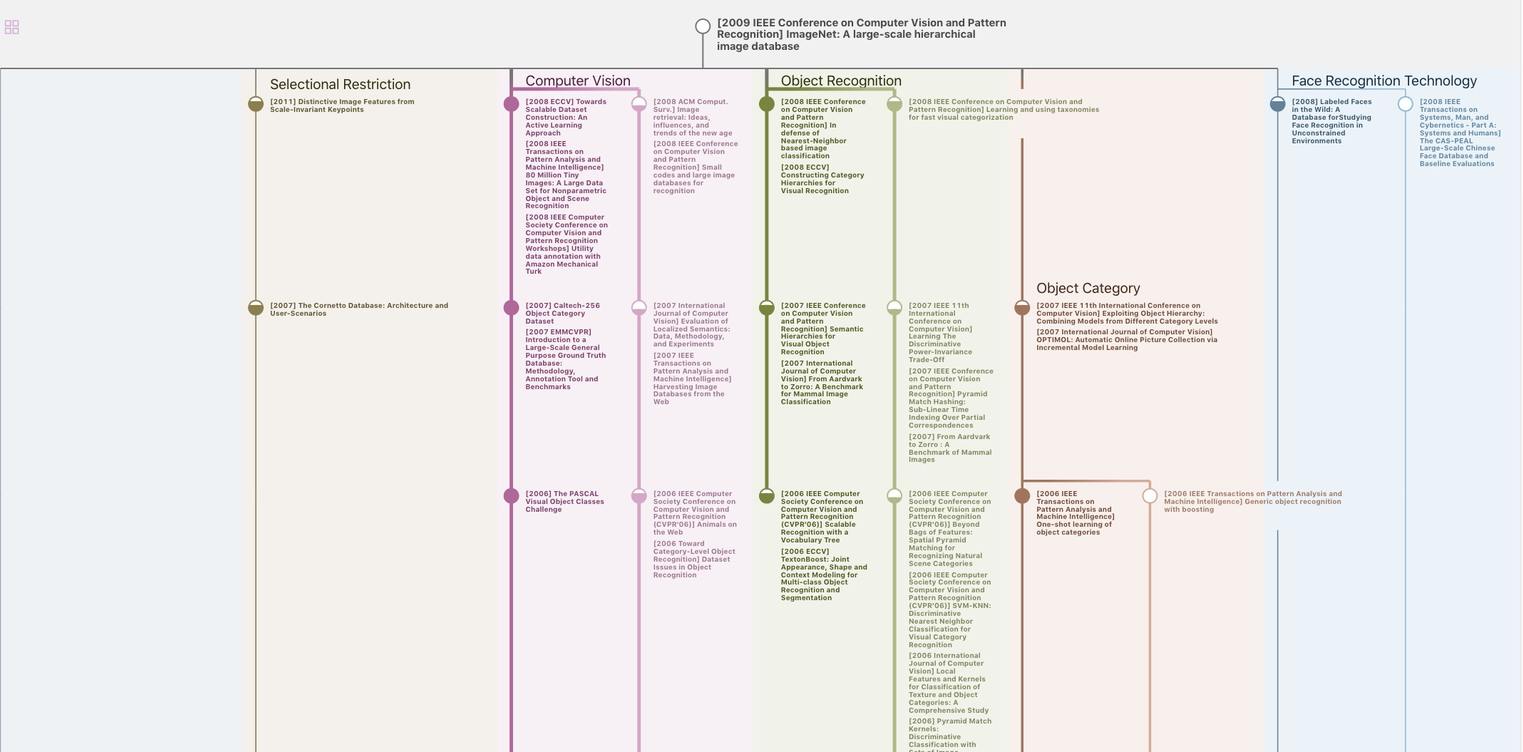
生成溯源树,研究论文发展脉络
Chat Paper
正在生成论文摘要