Dynamic Degradation Intensity Estimation for Adaptive Blind Super-Resolution: A Novel Approach and Benchmark Dataset
IEEE Transactions on Circuits and Systems for Video Technology(2023)
摘要
Blind Super-Resolution (BlindSR) aims to reconstruct high-resolution (HR) images from low-resolution (LR) images without prior knowledge of the image degradation process. This is a challenging problem in real-world applications, where the degradation can be complex and unknown. Recent unsupervised learning-based BlindSR methods can estimate the image degradation in an unsupervised manner, but they suffer from limited adaptability to different types and intensities of degradation. They tend to capture the average level of degradation across all training samples, resulting in over-smoothing or over-sharpening effects for some images. As a result, the final reconstruction may exhibit the mean effect. Moreover, existing synthetic datasets do not reflect the real-world degradation scenarios, making it difficult to evaluate the performance of BlindSR methods. To address these issues, we propose a novel Degradation Intensity Estimation Module (DIEM) method, which can estimate the pixel-level degradation information of the input image more specifically and use it to guide image reconstruction. Furthermore, we construct a benchmark dataset under real scenarios, which is closer to the real-world BlindSR problem than existing synthetic datasets, and can provide a more reasonable evaluation of BlindSR methods. Extensive experimental results demonstrate that our DIEM-guided BlindSR method can achieve state-of-the-art image reconstruction results. Our code and pre-trained models have been uploaded to GitHub† for validation.
更多查看译文
关键词
Blind Super-Resolution,Degradation Intensity Estimation,Image Reconstruction,Benchmark Dataset
AI 理解论文
溯源树
样例
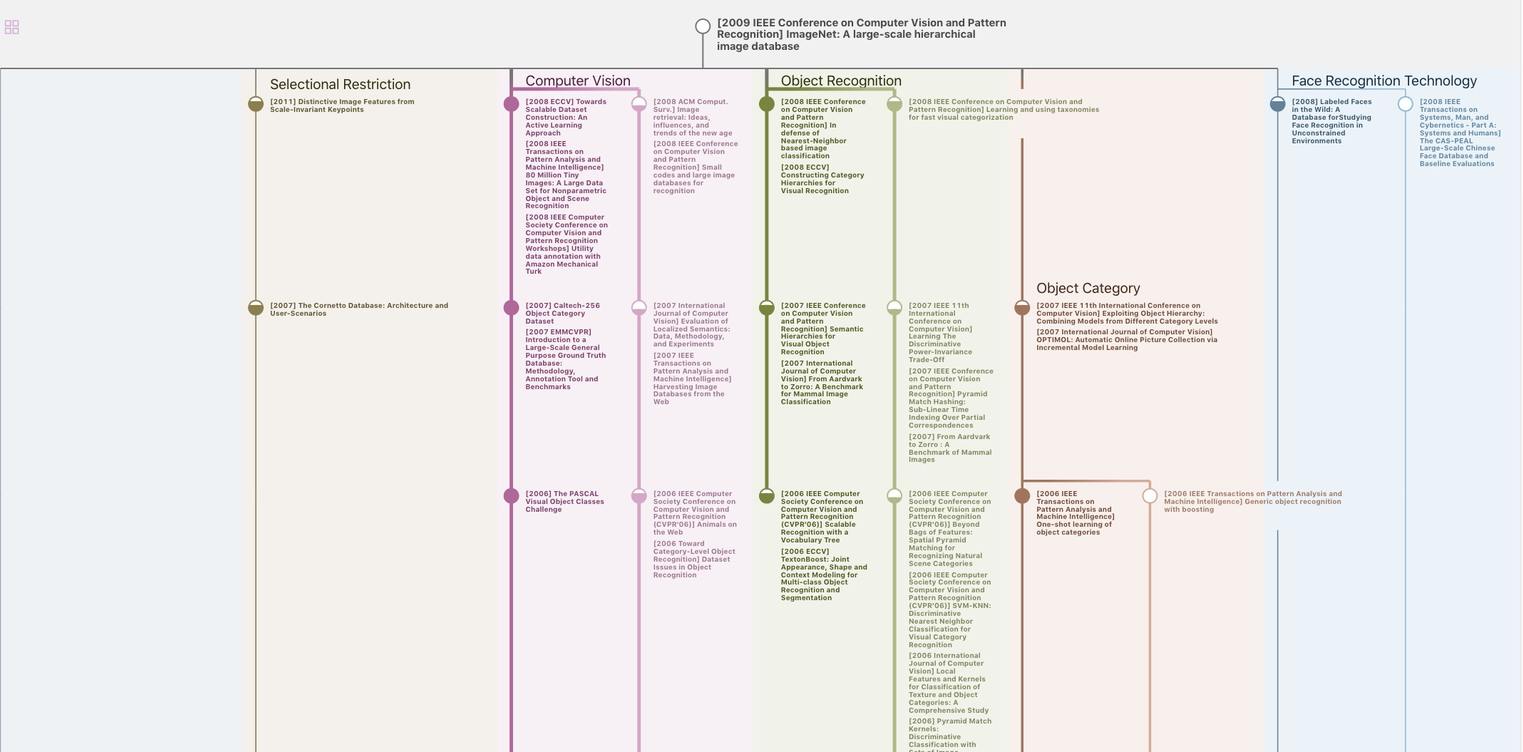
生成溯源树,研究论文发展脉络
Chat Paper
正在生成论文摘要