Learning to Predict Diverse Stable Placements for Extrinsic Manipulation on a Support Plane
IEEE Transactions on Cognitive and Developmental Systems(2023)
摘要
Extrinsic manipulation, a technique that enables robots to leverage extrinsic resources for object manipulation, presents practical yet challenging scenarios. Particularly in the context of extrinsic manipulation on a supporting plane, regrasping becomes essential for achieving the desired final object poses. This process involves sequential operation steps and stable placements of objects, which provide grasp space for the robot. To address this challenge, we focus on predicting diverse placements of objects on the plane using deep neural networks. A framework that comprises orientation generation, placement refinement, and placement discrimination stages is proposed, leveraging point clouds to obtain precise and diverse stable placements. To facilitate training, a large-scale dataset is constructed, encompassing stable object placements and contact information between objects. Through extensive experiments, our approach is demonstrated to outperform the start-of-the-art, achieving an accuracy rate of 90.4% and a diversity rate of 81.3% in predicted placements. Furthermore, we validate the effectiveness of our approach through real-robot experiments, demonstrating its capability to compute sequential pick-and-place steps based on the predicted placements for regrasping objects to goal poses that are not readily attainable within a single step. Videos and dataset are available at
https://sites.google.com/view/pmvlr2022/
.
更多查看译文
关键词
Regrasping,Deep learning,Extrinsic manipulation
AI 理解论文
溯源树
样例
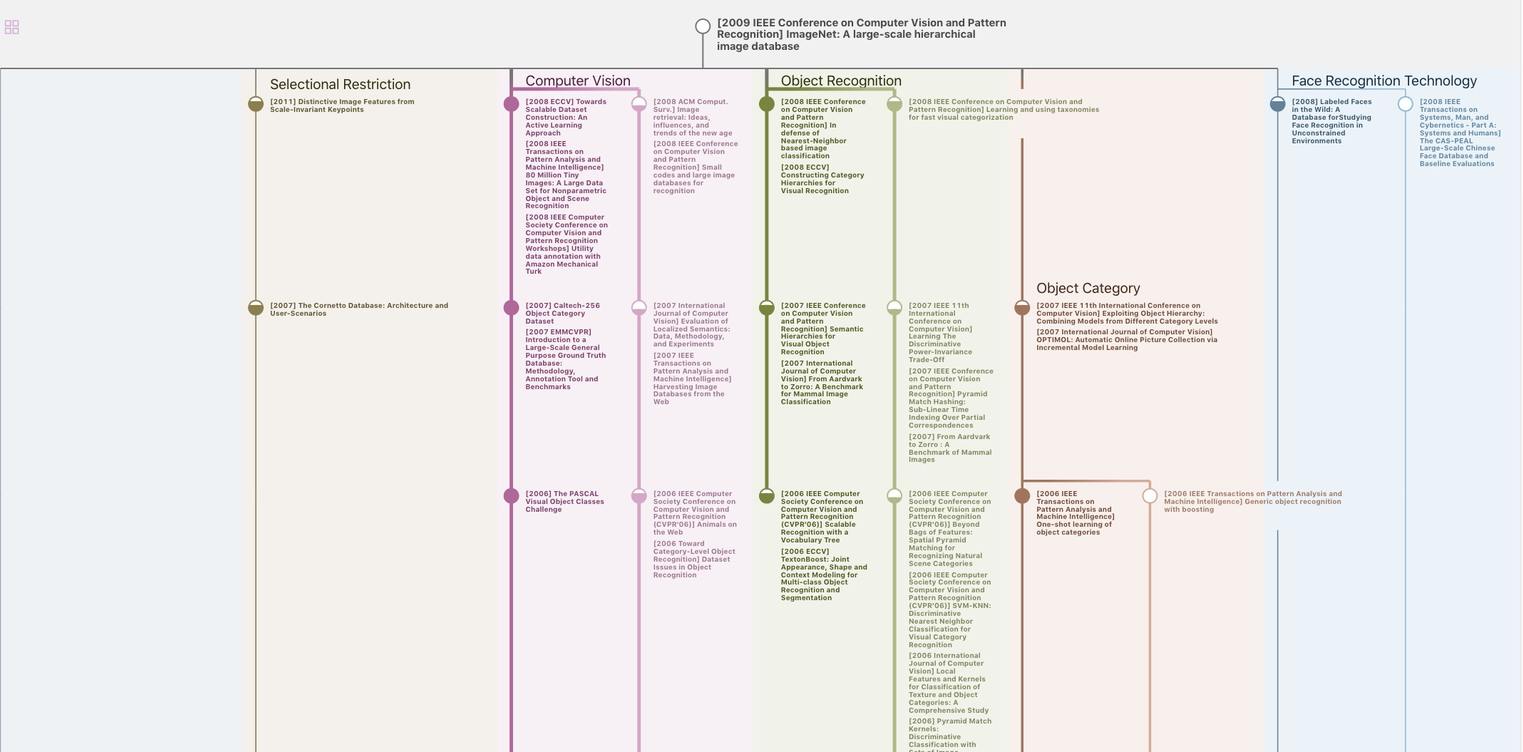
生成溯源树,研究论文发展脉络
Chat Paper
正在生成论文摘要