Identifying GNSS NLOS Using Visual Label and Ensemble Tree Under Complex City Environment
Lecture notes in electrical engineering(2023)
摘要
GNSS NLOS signals in complex urban canyons can produce large ranging errors, and the detection and classification of LOS and NLOS signals is a key factor in improving the accuracy of vehicle navigation and positioning. Vision sensors can reflect scene occlusion information in real time, and machine learning is high speed and accurate in processing multiple types of features. In this paper, the advantages of both methods are combined, and the NLOS signals detected by INS and fisheye images are used as visual labels. Six feature values of signal-to-noise ratio and pseudorange consistency, altitude angle and azimuth angle of two frequency points are selected, and NLOS signals based on gradient boosted decision tree (GBDT) and random forest (RF) are designed respectively. The models were trained using urban in-vehicle dynamic data collected by low-cost UBLOX receivers, and compared with three single-learner models: logistic regression (LR), support vector machine (SVM) and naive Bayesian (NB). The experimental results show that the proposed model can be used for real-time dynamic scenes in complex urban environments, is not dependent on additional hardware devices and is suitable for general users. The LOS/NLOS signal classification performance of the GBDT and RF algorithms were comparable, with precision, recall and F1_score reaching over 86%, significantly outperforming the LR, SVM and NB algorithms. The feature importance ranking based on both GBDT and RF algorithms showed that the importance of altitude angle and azimuth angle was greater than the importance of pseudorange consistency and signal-to-noise ratio at both frequencies.
更多查看译文
关键词
gnss nlos,ensemble tree,complex city environment,visual label
AI 理解论文
溯源树
样例
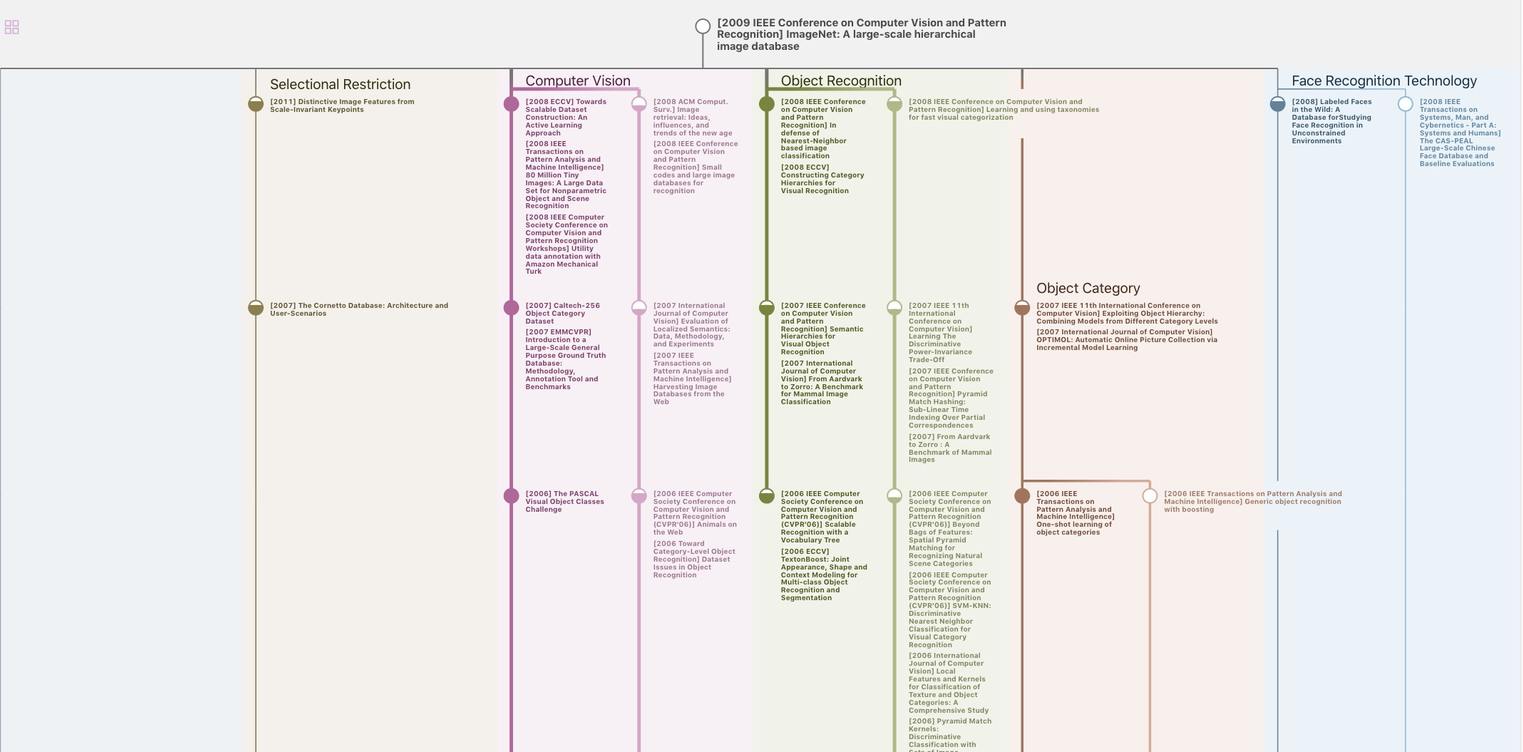
生成溯源树,研究论文发展脉络
Chat Paper
正在生成论文摘要