Refinement of structural theories for composite shells through convolutional neural networks
Materials research proceedings(2023)
摘要
Abstract. This study examines the use of Convolutional Neural Networks (CNN) to determine the optimal structural theories to adopt for the modeling of composite shells, to combine accuracy and computational efficiency. The use of the Axiomatic/Asymptotic Method (AAM) on higher-order theories (HOT) based on polynomial expansions can be cumbersome due to the amount of Finite Element Models (FEM) virtually available and the problem-dependency of a theory’s performance. Adopting the Carrera Unified Formulation (CUF) can mitigate this obstacle through its procedural and lean derivation of the required structural results. At the same time, the CNN can act as a surrogate model to guide the selection process. The network can inform on the convenience of a specific set of generalized variables after being trained with just a small percentage of the results typically required by the AAM. The CNN capabilities are compared to the AAM through the Best Theory Diagram (BTD) obtained using different selection criteria: errors over natural frequencies or failure indexes.
更多查看译文
关键词
composite shells,structural theories
AI 理解论文
溯源树
样例
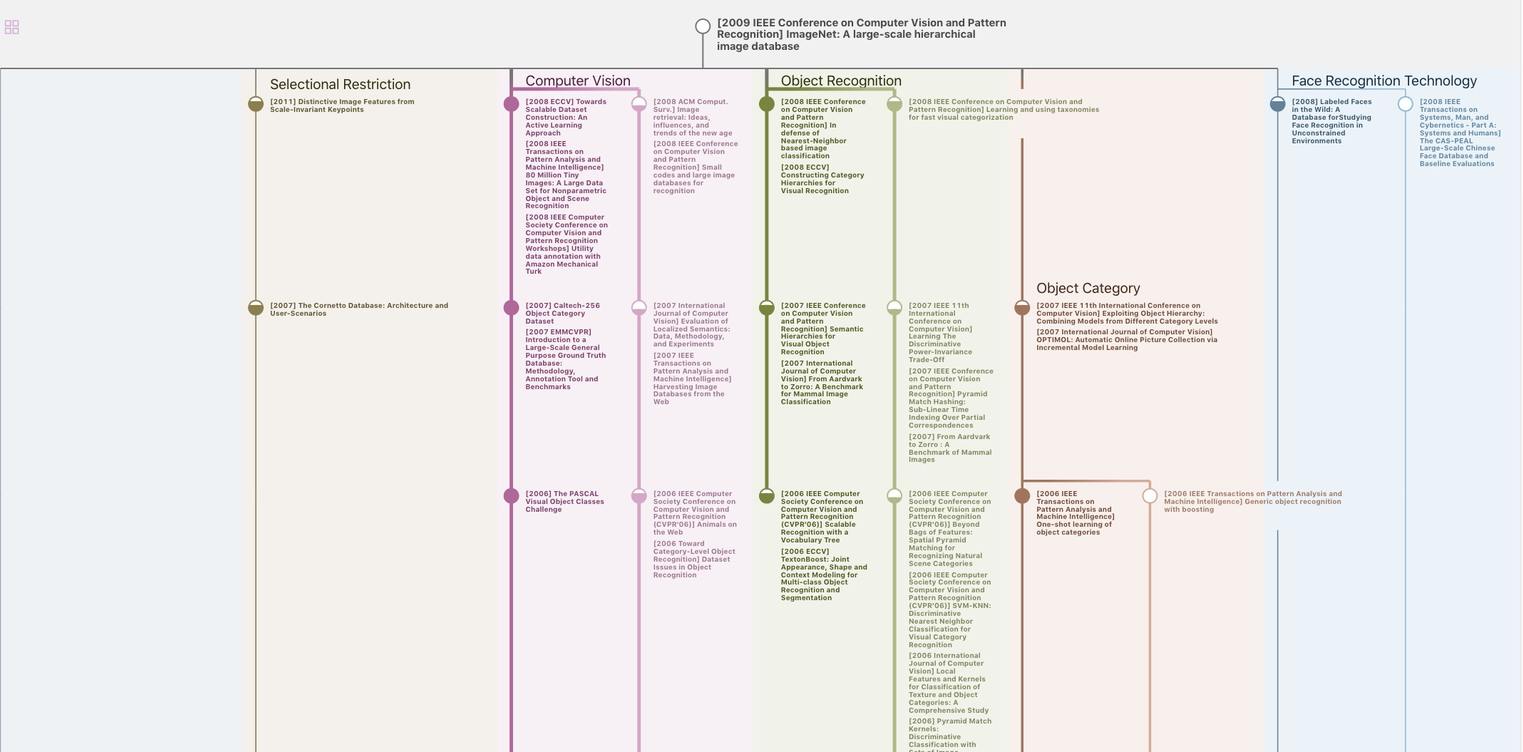
生成溯源树,研究论文发展脉络
Chat Paper
正在生成论文摘要