Skin the sheep not only once: Reusing Various Depth Datasets to Drive the Learning of Optical Flow
arXiv (Cornell University)(2023)
摘要
Optical flow estimation is crucial for various applications in vision and robotics. As the difficulty of collecting ground truth optical flow in real-world scenarios, most of the existing methods of learning optical flow still adopt synthetic dataset for supervised training or utilize photometric consistency across temporally adjacent video frames to drive the unsupervised learning, where the former typically has issues of generalizability while the latter usually performs worse than the supervised ones. To tackle such challenges, we propose to leverage the geometric connection between optical flow estimation and stereo matching (based on the similarity upon finding pixel correspondences across images) to unify various real-world depth estimation datasets for generating supervised training data upon optical flow. Specifically, we turn the monocular depth datasets into stereo ones via synthesizing virtual disparity, thus leading to the flows along the horizontal direction; moreover, we introduce virtual camera motion into stereo data to produce additional flows along the vertical direction. Furthermore, we propose applying geometric augmentations on one image of an optical flow pair, encouraging the optical flow estimator to learn from more challenging cases. Lastly, as the optical flow maps under different geometric augmentations actually exhibit distinct characteristics, an auxiliary classifier which trains to identify the type of augmentation from the appearance of the flow map is utilized to further enhance the learning of the optical flow estimator. Our proposed method is general and is not tied to any particular flow estimator, where extensive experiments based on various datasets and optical flow estimation models verify its efficacy and superiority.
更多查看译文
关键词
various depth datasets,flow,learning,sheep
AI 理解论文
溯源树
样例
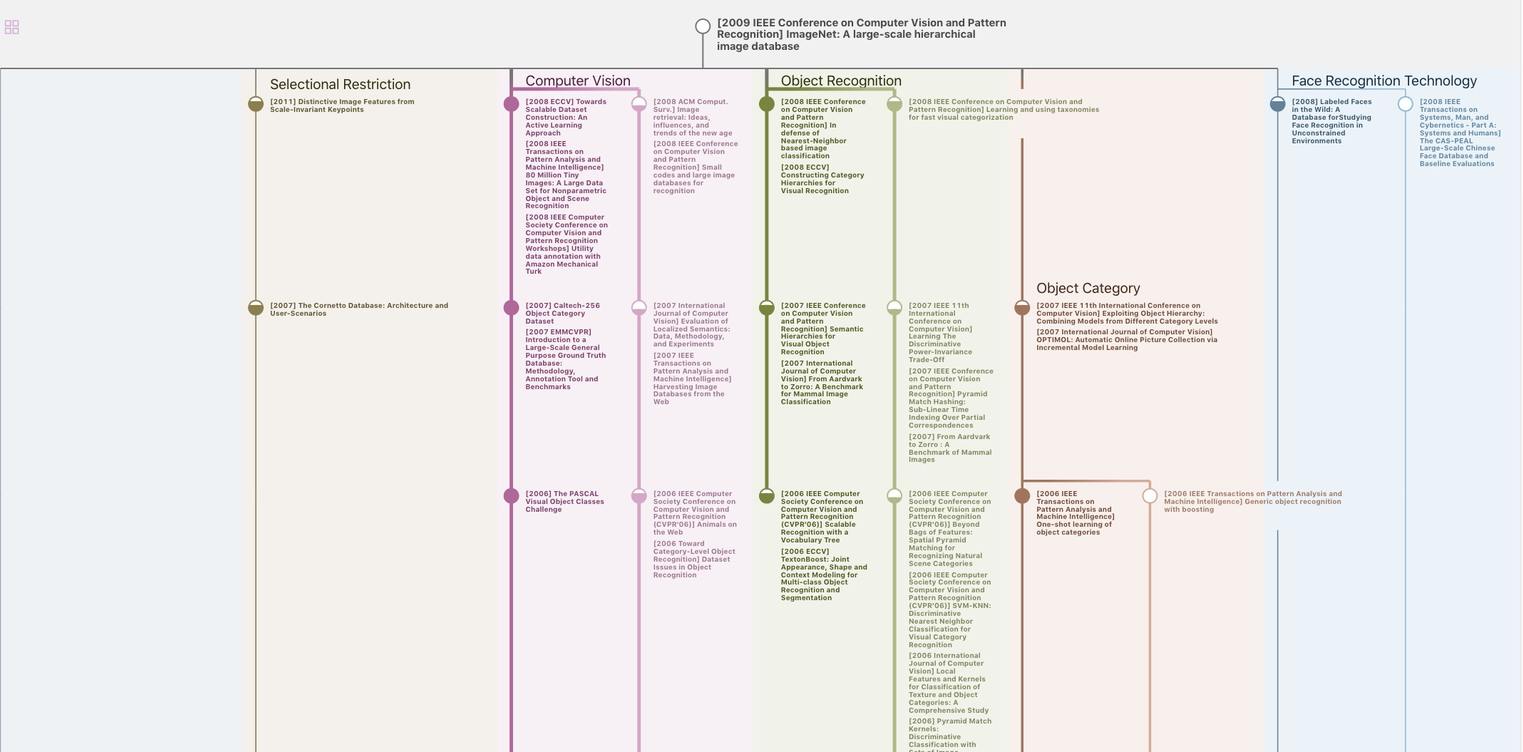
生成溯源树,研究论文发展脉络
Chat Paper
正在生成论文摘要