Rapid detection of fetal compromise using input length invariant deep learning on fetal heart rate signals
Research Square (Research Square)(2023)
摘要
Abstract Standard clinical practice to assess fetal well-being during labour utilises monitoring of the fetal heart rate (FHR) using cardiotocography. However, visual evaluation of FHR signals can result in subjective interpretations leading to inter and intra-observer disagreement. Therefore, recent studies have proposed deep-learning-based methods to interpret FHR signals and detect fetal compromise. These methods have typically focused on evaluating fixed-length FHR segments at the conclusion of labour, leaving little time for clinicians to intervene. In this study, we propose a novel FHR evaluation method using an input length invariant deep learning model to progressively evaluate FHR as labour progresses and achieve rapid detection of fetal compromise. Using a new performance metric called time to predict (TTP), we achieve a 25% reduction in the time taken to detect fetal compromise compared to the state-of-the-art multimodal convolutional neural network (MCNN), with equivalent sensitivity at a 20% false positive rate. A diagnostic system based on our approach could potentially enable earlier intervention for fetal compromise and improve clinical outcomes.
更多查看译文
关键词
fetal compromise,invariant deep learning,deep learning,heart
AI 理解论文
溯源树
样例
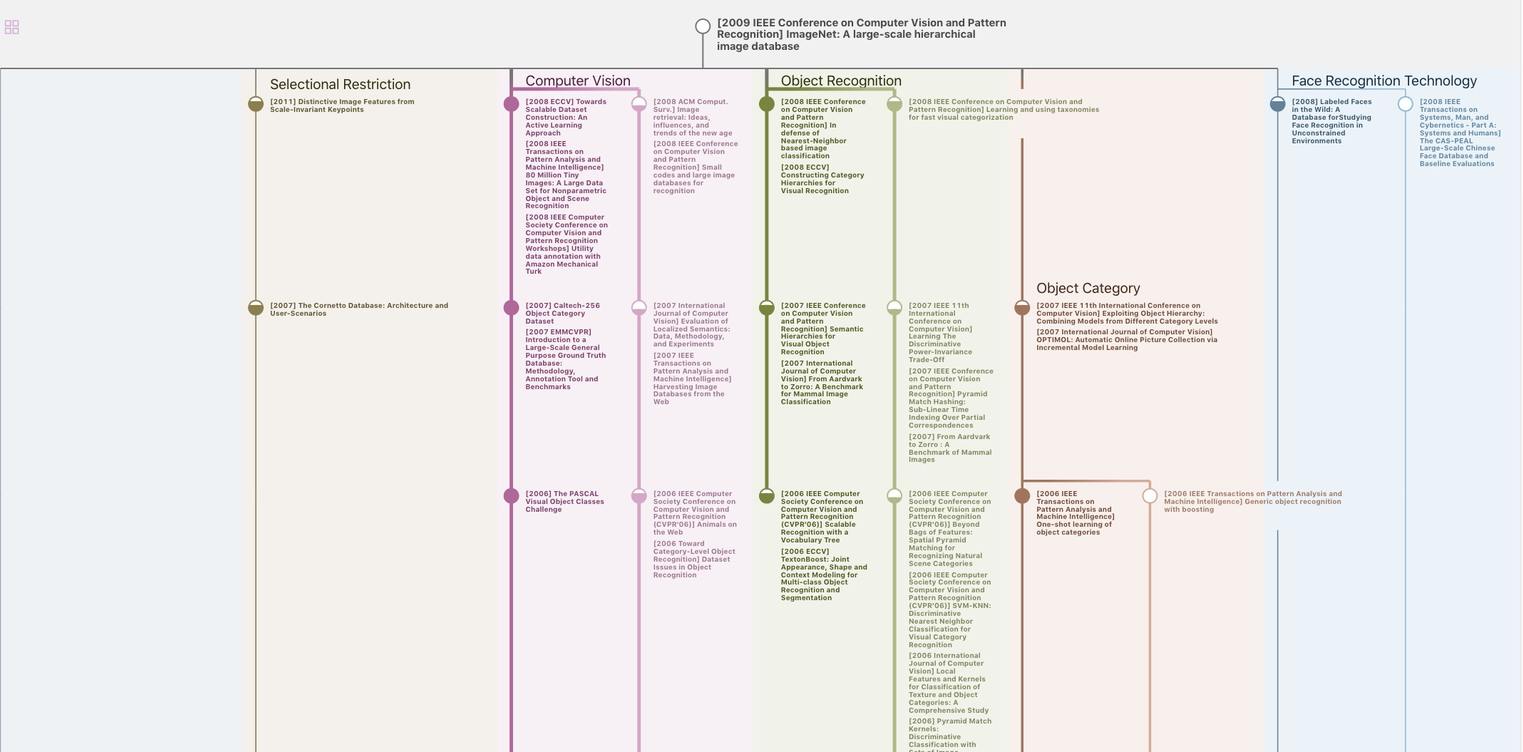
生成溯源树,研究论文发展脉络
Chat Paper
正在生成论文摘要