Efficient Byzantine-Robust and Privacy-Preserving Federated Learning on Compressive Domain.
IEEE Internet Things J.(2024)
摘要
Data privacy and resistance against poisoning attack (Byzantine-robustness) are two critical concerns of Federated Learning (FL). Addressing the two issues simultaneously is challenging, since privacy-preserving mechanism tends to make the data be indistinguishable, whereas Byzantine-robustness methods require access for the data to make comprehensive analysis. To solve this problem, in this paper, we propose a novel defender for privacy-ensured Byzantine-robust FL on compressive domain. Unlike existing works that mainly using computation-intensive techniques, our method leverages Compressive Sensing (CS) as a lightweight encryption to protect the data privacy, while maintaining the possibility of Byzantine-robustness analysis on the encrypted (compressive) model update (i.e., gradient). Our key insight is that the cosine similarity can be approximately measured on the compressive measurements of any two normalized vectors, thus makes it be feasible to identify the malicious gradients on the CS compressive domain. We theoretically prove the correctness of our method. Notably, due to the dimensionality reduction of CS, the computation and communication overhead of our system can be significantly reduced. This makes our scheme be fit for applying in the applications with resource-constrained devices, such as Internet of Things (IoT). Experimental results demonstrate the effectiveness and efficiency of our method.
更多查看译文
关键词
Federated Learning,Byzantine-robust,Compressive Sensing,Confidentiality,Low Overhead
AI 理解论文
溯源树
样例
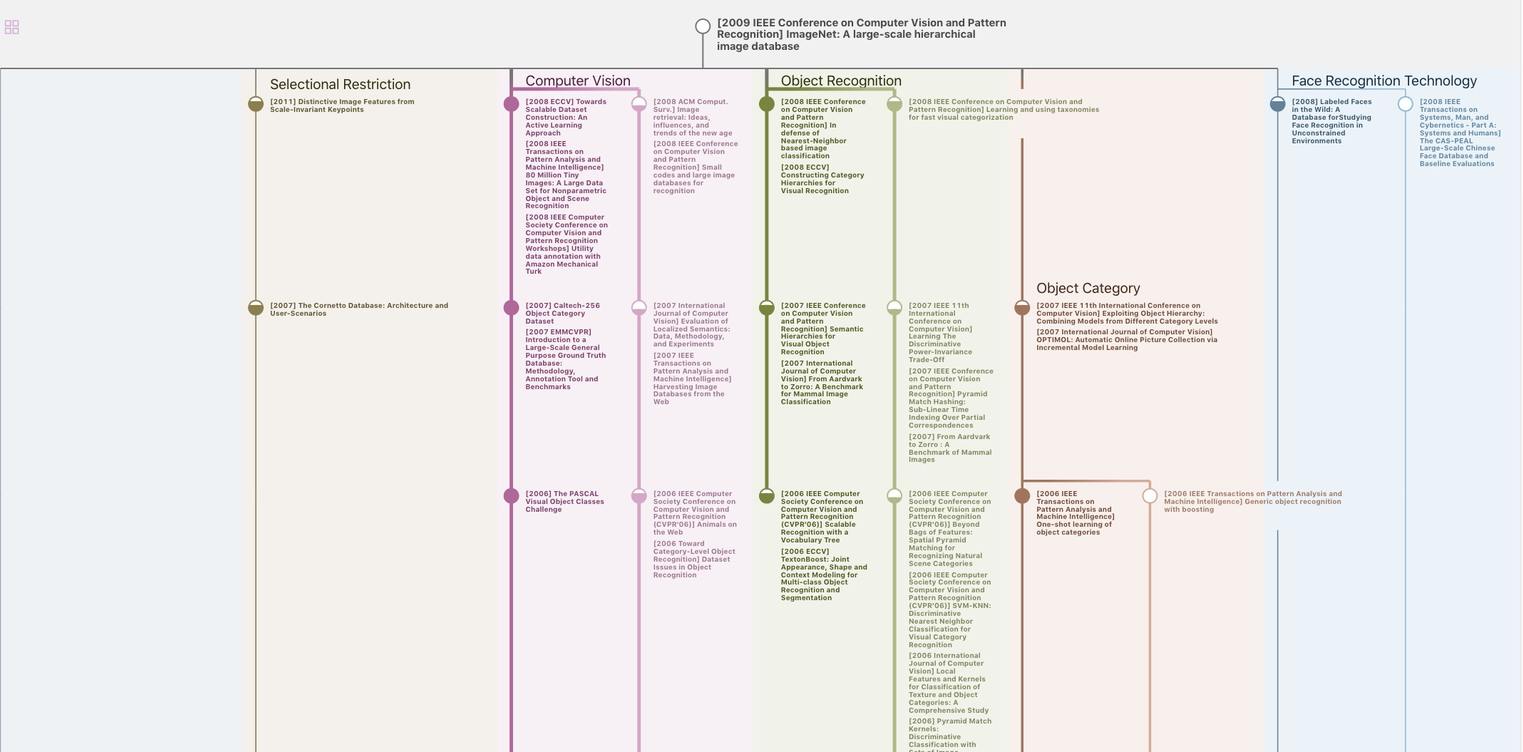
生成溯源树,研究论文发展脉络
Chat Paper
正在生成论文摘要