Clustering and Predicting Multiple Time Series Count Data via Mixture of Bayesian Predictive Syntheses: Analysis of COVID-19 Hospitalisation in Japan and Korea
arXiv (Cornell University)(2023)
摘要
Since the outbreak of COVID-19, governments and academia have made tremendous efforts to predict the course of the pandemic and implement prevention measures by monitoring various indicators. These indicators are obtained daily or weekly, and typically constitute time series count data over multiple sub-regions of a country, where groups of sub-regions often exhibit similar dynamics. This paper proposes a novel methodology called the mixture of Bayesian predictive syntheses (MBPS) to improve the predictive performance for such data by combining a set of predictive models and clustering the time series based on the contribution of those predictive models. MBPS takes the form of a finite mixture of dynamic factor models and is particularly useful when the multiple time series are discrete, as MBPS does not require multivariate count models, which are generally cumbersome to develop and implement. The clustering through MBPS provides valuable insights into the data and improves predictive performance by leveraging the share information within each cluster. Grouping multiple series into clusters also reduces the number of parameters needed compared to employing a multivariate model. To validate its efficacy, we apply the model to analyse the data on the numbers of COVID-19 inpatients and isolated individuals in Japan and Korea. The results demonstrate that our proposed approach outperforms the agent methods in predictive accuracy. Moreover, the insights gained from our model provide valuable information for interpreting and diagnosing the analysis, contributing to the formulation of preventive measures for infectious diseases in the future.
更多查看译文
关键词
bayesian predictive syntheses,time series
AI 理解论文
溯源树
样例
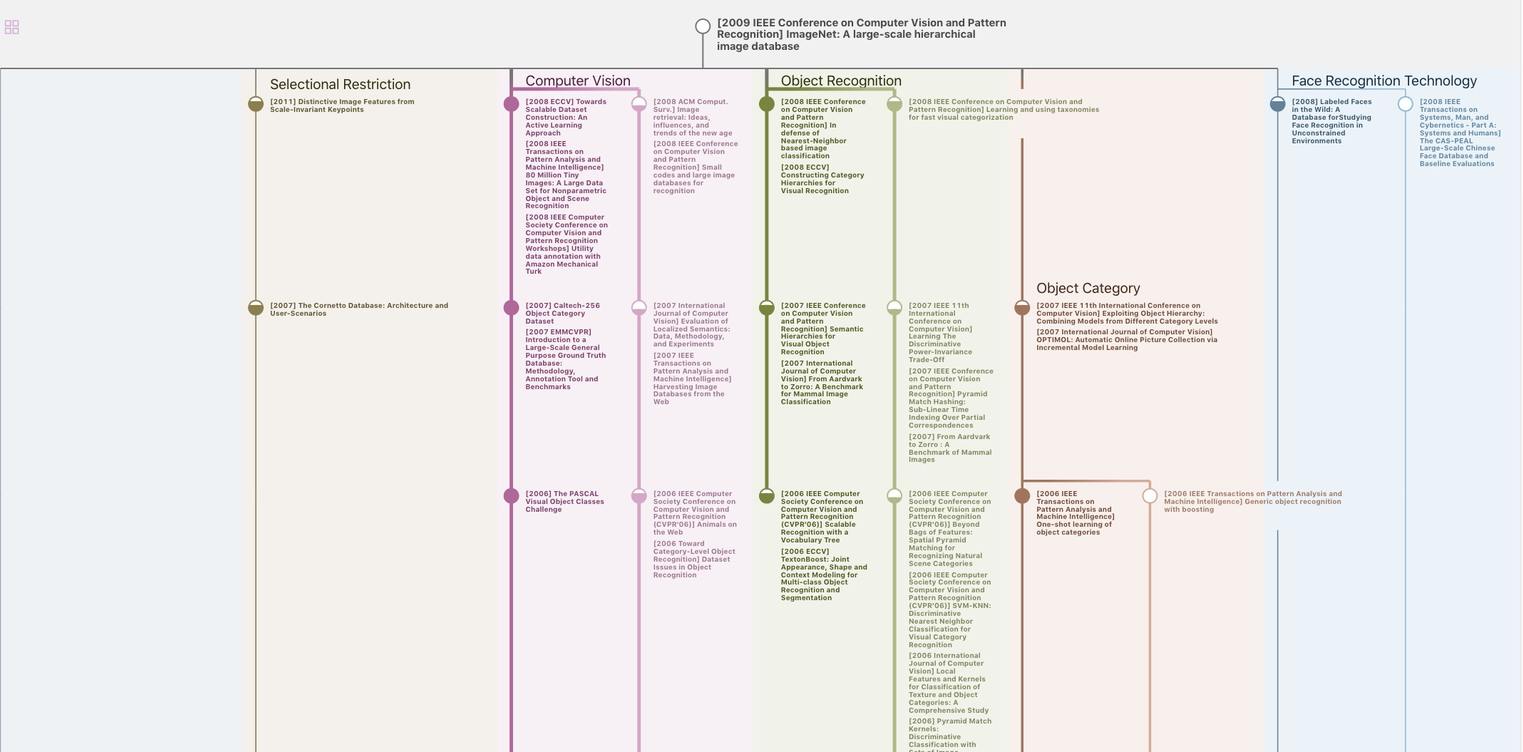
生成溯源树,研究论文发展脉络
Chat Paper
正在生成论文摘要