Learning high-dimensional reaction coordinates of fast-folding proteins using State Predictive information bottleneck and Bias Exchange Metadynamics
bioRxiv (Cold Spring Harbor Laboratory)(2023)
摘要
ABSTRACT Biological events occurring on long timescales, such as protein folding, remain hard to capture with conventional molecular dynamics (MD) simulation. To overcome these limitations, enhanced sampling techniques can be used to sample regions of the free energy landscape separated by high energy barriers, thereby allowing to observe these rare events. However, many of these techniques require a priori knowledge of the appropriate reaction coordinates (RCs) that describe the process of interest. In recent years, Artificial Intelligence (AI) models have emerged as promising approaches to accelerate rare event sampling. However, integration of these AI methods with MD for automated learning of improved RCs is not trivial, particularly when working with undersampled trajectories and highly complex systems. In this study, we employed the State Predictive Information Bottleneck (SPIB) neural network, coupled with bias exchange metadynamics simulations (BE-metaD), to investigate the unfolding process of two proteins, chignolin and villin. By utilizing the high-dimensional RCs learned from SPIB even with poor training data, BE-metaD simulations dramatically accelerate the sampling of the unfolding process for both proteins. In addition, we compare different RCs and find that the careful selection of RCs is crucial to substantially speed up the sampling of rare events. Thus, this approach, leveraging the power of AI and enhanced sampling techniques, holds great promise for advancing our understanding of complex biological processes occurring on long timescales. Abstract Figure TABLE OF CONTENT GRAPHIC
更多查看译文
关键词
state predictive information bottleneck,proteins,bias exchange metadynamics,learning,high-dimensional,fast-folding
AI 理解论文
溯源树
样例
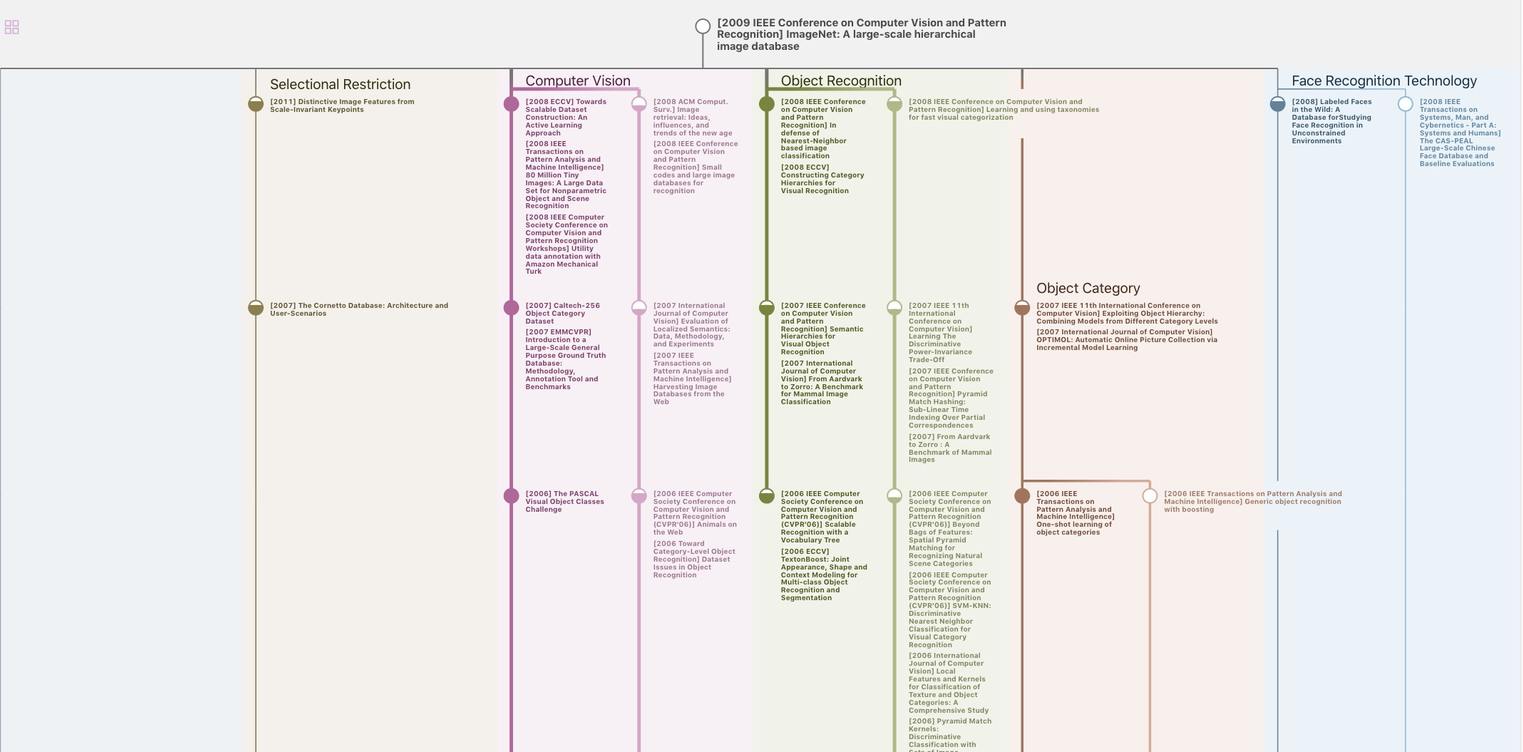
生成溯源树,研究论文发展脉络
Chat Paper
正在生成论文摘要