Performance Comparative of Surrogate Models as Fitness Functions for Metaheuristic Algorithms
Studies in computational intelligence(2023)
摘要
Real-world optimization problems can use a high number of computational resources to be solved because of the complexity of the fitness function or objective function. This situation negatively impacts the performance of any Heuristic or deterministic solver since most of the computational time is invested into processing the fitness function. Surrogate models are used to reduce the computational time of processing complex functions by replacing extensive numerical simulations with approximate functions that are faster to evaluate. Surrogate models are constructed by evaluating the original model at a set of points (training), and then using the corresponding evaluations to construct an approximate model based on given mathematical functions. The main idea is to have a faster function that can guide an optimization solver with a minimum error or difference against the original fitness function. In this work, several surrogated models are constructed and compared in the context of a heuristic optimization solver to analyze their performance as fitness functions.
更多查看译文
关键词
metaheuristic algorithms,surrogate models,fitness functions
AI 理解论文
溯源树
样例
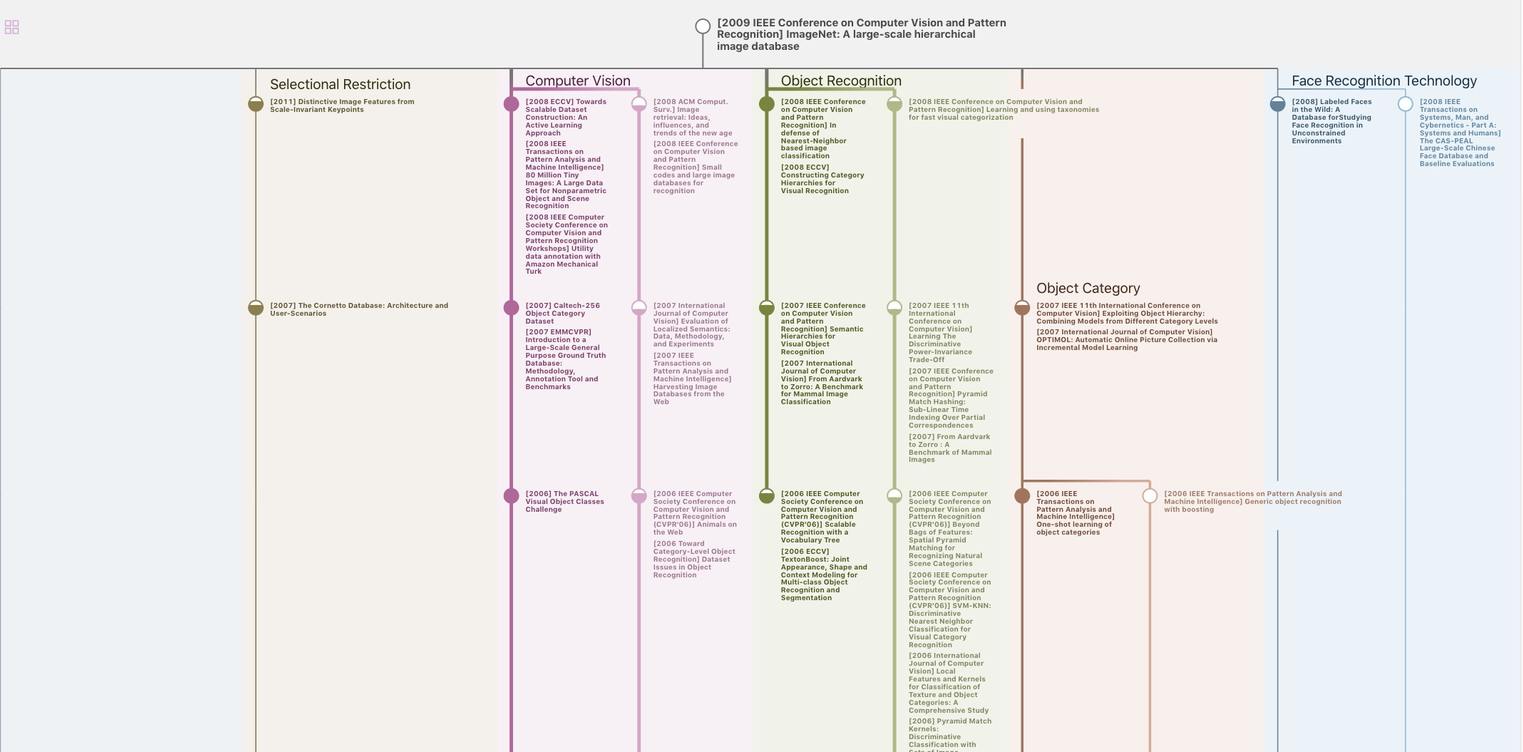
生成溯源树,研究论文发展脉络
Chat Paper
正在生成论文摘要