Lithography Hotspot Detection Based on Heterogeneous Federated Learning with Local Adaptation and Feature Selection
IEEE Transactions on Computer-Aided Design of Integrated Circuits and Systems(2023)
摘要
Since the scaling of advanced technology nodes is pushing to its physical limit, lithography hotspot detection has become more significant than ever in design for manufacturability. Recently, machine learning techniques have been deployed to greatly reduce simulation time for hotspot detection, but high-quality data are required to build a model. Many design companies do not have enough high-quality data and are hesitant to share it for fear of intellectual property theft or model ineffectiveness. Furthermore, using locally trained models with limited and similar data can lead to overfitting and lack of generalization and robustness when applied to new designs. In this paper, we propose a heterogeneous federated learning framework for lithography hotspot detection that can address the aforementioned issues. Our framework can overcome the challenges of non-independent and identically distributed data and heterogeneous communication, ensuring high performance and good convergence in various scenarios. The proposed framework creates a more robust centralized global sub-model through heterogeneous knowledge sharing while keeping local data private. Then, it combines the global sub-model with a local sub-model for better adaptation to local data heterogeneity. Our experimental results show that the proposed framework outperforms other state-of-the-art methods.
更多查看译文
AI 理解论文
溯源树
样例
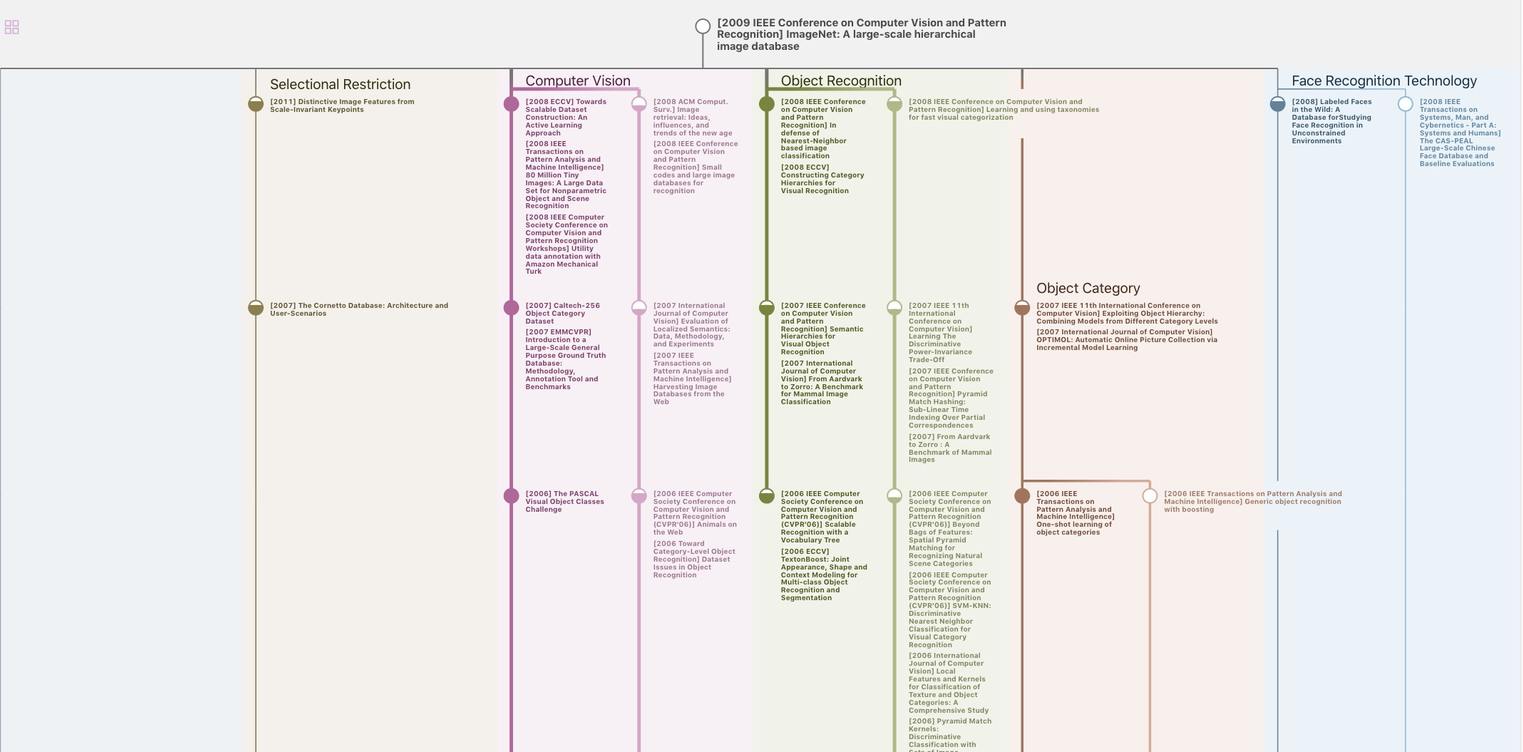
生成溯源树,研究论文发展脉络
Chat Paper
正在生成论文摘要