Land Subsidence Susceptibility Mapping Using Machine Learning in the Google Earth Engine Platform
Lecture notes on data engineering and communications technologies(2023)
摘要
This study aims to compare the effectiveness of two predictive models, CART regression and Random Forest in mapping land subsidence susceptibility. The analysis is supported by the Google Earth Engine cloud computing platform. The study focuses on Camau province, located in the Mekong Delta, where significant land subsidence occurs annually. Eight variables were considered in the models, including elevation, slope, aspect, land cover, NDVI, soil map, geology, and groundwater level. Land subsidence points, obtained through the PSInSAR method, were used in the study, comprising a total of 989 points. These points were divided into a 70% training dataset and a 30% testing dataset for both models. The results produced a land subsidence sensitivity map categorized into five levels: very low, low, moderate, high, and very high. The performance of the models was evaluated using ROC curve and the area under the curve (AUC). The AUC values for the Random Forest (RF) model are 0.86 and 0.87 for the training and validation datasets, respectively. In comparison, the CART model achieves AUC values of 0.79 and 0.73 for the training and validation datasets, respectively. The research findings demonstrate an 7% superior performance of the RF model compared to the CART method. Therefore, the RF model is chosen as the final model for land subsidence susceptibility mapping in Camau.
更多查看译文
关键词
land,earth,machine learning,mapping
AI 理解论文
溯源树
样例
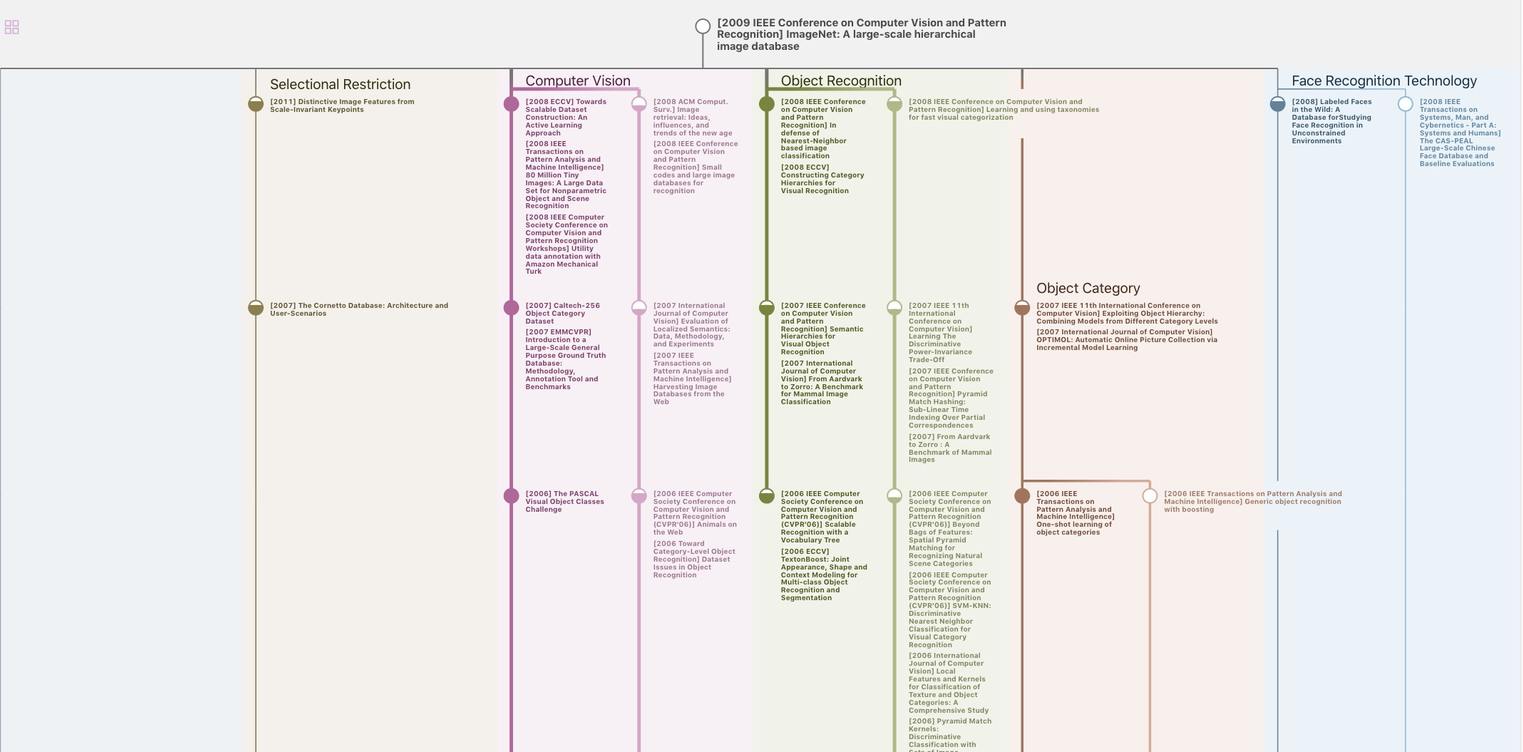
生成溯源树,研究论文发展脉络
Chat Paper
正在生成论文摘要