Two-Dimensional Following Lane-Changing (2DF-LC): A Framework for Dynamic Decision-Making and Rapid Behavior Planning
IEEE transactions on intelligent vehicles(2023)
摘要
Lane changes require dynamic decision-making and rapid behavior planning, which are challenging for traffic modeling. We propose a two-dimensional following lane-changing framework (2DF-LC) that exploits the benefits of car-following (CF) models for computational efficiency, collision avoidance, and human-like behavior. This framework uses a sigmoid-based intelligent driver model (SIDM) with both longitudinal and lateral following. To avoid excessive acceleration at start-up, we develop an SIDM that ensures a smooth start-up. In the longitudinal plane, we introduce a transition function to create a double-target car-following model (DT-SIDM) that can handle sudden acceleration changes due to target switching, thereby guaranteeing stable longitudinal motion and dynamic collision avoidance. In the lateral plane, we develop a lateral movement car-following model (LM-SIDM) inspired by a social force model. The LM-SIDM defines both lane and gap forces, resulting in effective lateral motion and collision avoidance during lane changes. Simulations and tests in three typical scenarios show that 2DF-LC has high computational efficiency: it completes calculations within milliseconds. Compared with the widely used hierarchical motion planning system (HMPS) and integrated model and learning combined algorithm (IMLC) methods, 2DF-LC based on real trajectories reduces the errors by 49.5% and 16.1%, respectively, and achieves a 28.63% lower time-integrated anticipated collision time (TI-ACT) than the original trajectories, indicating improved safety. Moreover, 2DF-LC produces a smooth acceleration curve, with an average jerk value of 0.358 m/s
3
. The lane-change trajectory generated by 2DF-LC can also be followed and executed effectively in CarSim tests.
更多查看译文
关键词
Small-scale traffic models,Lane change,Two-dimensional car-following,Social forces,Human-like driving
AI 理解论文
溯源树
样例
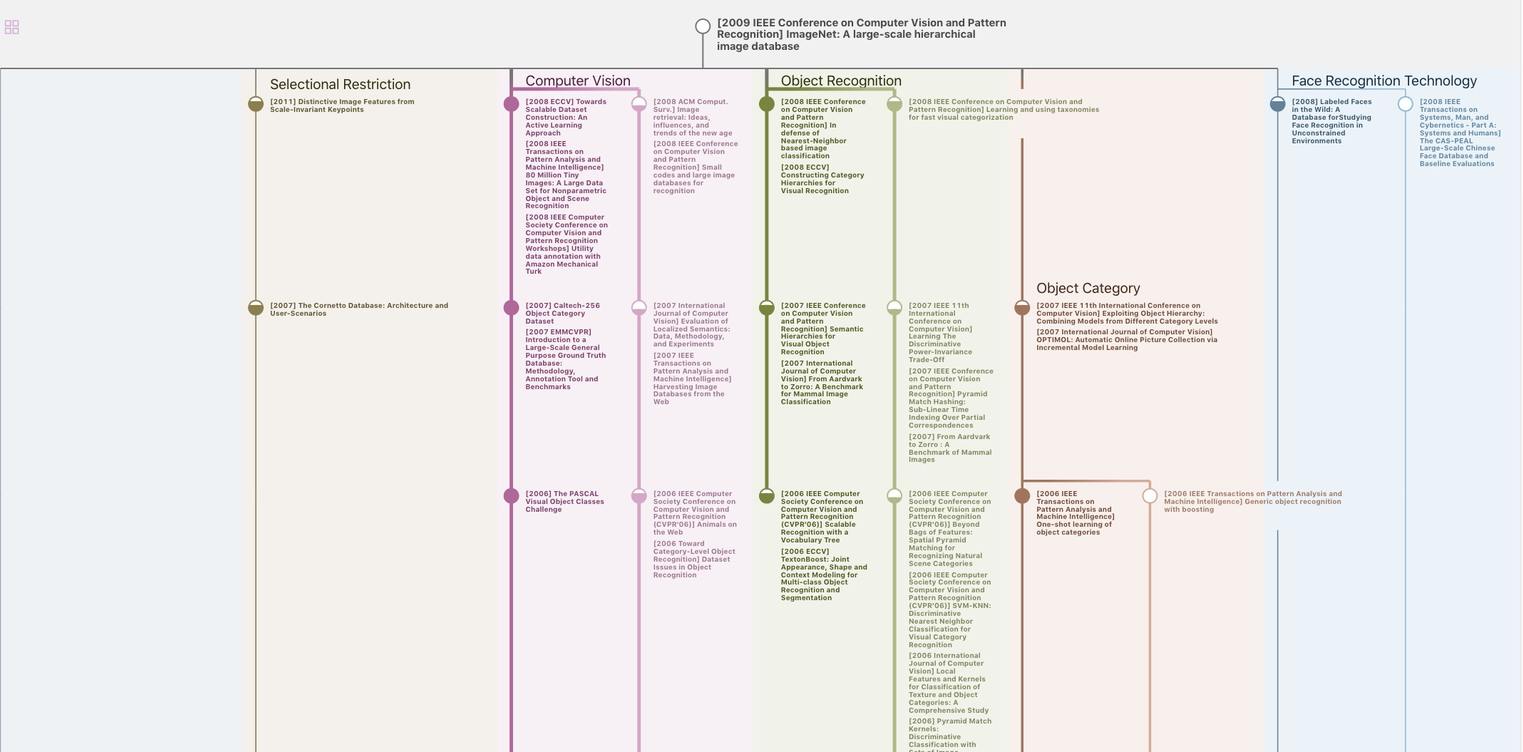
生成溯源树,研究论文发展脉络
Chat Paper
正在生成论文摘要