Zero-shot prediction of mutation effects on protein function with multimodal deep representation learning
Research Square (Research Square)(2023)
摘要
Abstract Mutations in amino acid sequences can disrupt protein structures and functions. Accurate and unsupervised prediction of mutation effects is critical in biotechnology and biomedicine, but remains a fundamental challenge. To resolve this challenge, we first presented a multimodal deep representation learning model that comprehensively learns both sequence context and structural constraints from ~160 million proteins with reliable structures. Based on the proposed model, we developed ProMEP (Protein Mutational Effect Predictor) to predict mutation effects in a zero-shot manner. ProMEP can capture multi-scale signatures of proteins at atomic-resolution and achieves state-of-the-art (SOTA) performance on mutational effects prediction. ProMEP showed an average accuracy of 91.67% during the engineering of the TnpB protein. Remarkably, ProMEP identified a TnpB variant of triple-mutations with approximately 2.4-fold editing efficiency than the wild-type. ProMEP enables efficient exploration of gigantic protein space and will greatly facilitate studies in protein structures and functions.
更多查看译文
关键词
mutation effects,representation learning,protein function,zero-shot
AI 理解论文
溯源树
样例
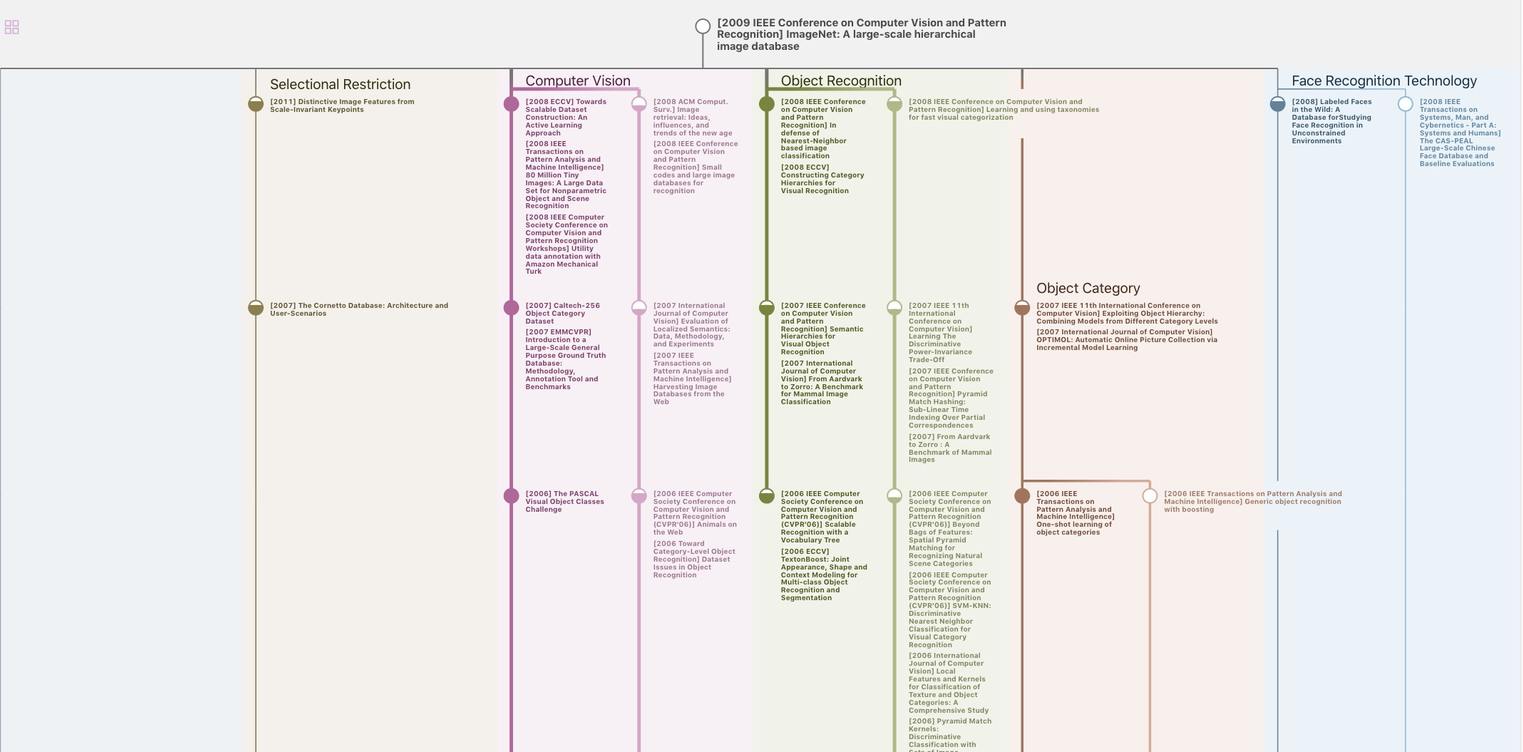
生成溯源树,研究论文发展脉络
Chat Paper
正在生成论文摘要