Radar Waveform Gaming Configurable Framework Based on Deep Reinforcement Learning
Smart innovation, systems and technologies(2023)
摘要
Radar faces a variety of different jamming techniques, among which mainlobe jamming is difficult to deal with. Traditional experience-based passive anti-jamming methods are usually less effective when facing flexible mainlobe jamming, while frequency agile (FA) radars can actively adopt various anti-jamming strategies to avoid being jammed. To enable FA radar to obtain better performance, a radar waveform gaming configurable framework based on deep reinforcement learning (RL) is proposed. This framework unifies the interfaces of three modules: anti-jamming methods, reward functions and the deep RL algorithms. The basic framework can be used for future expansion of algorithms and improvement of anti-jamming performance. The detecting rate is used as the reward and the frequency hopping of the radar signal is used as the anti-jamming method. The effectiveness of this framework is proved by accessing four RL algorithms. Meanwhile, the effects of different reward functions on the convergence and stability of the algorithms are analyzed for the four algorithms, which provide guidance for extending the reward function module. The simulation results show that under this framework system, all four RL algorithms can learn better anti-jamming strategies, which make the FA radar less affected by jamming and obtain a higher detecting rate of countermeasures.
更多查看译文
AI 理解论文
溯源树
样例
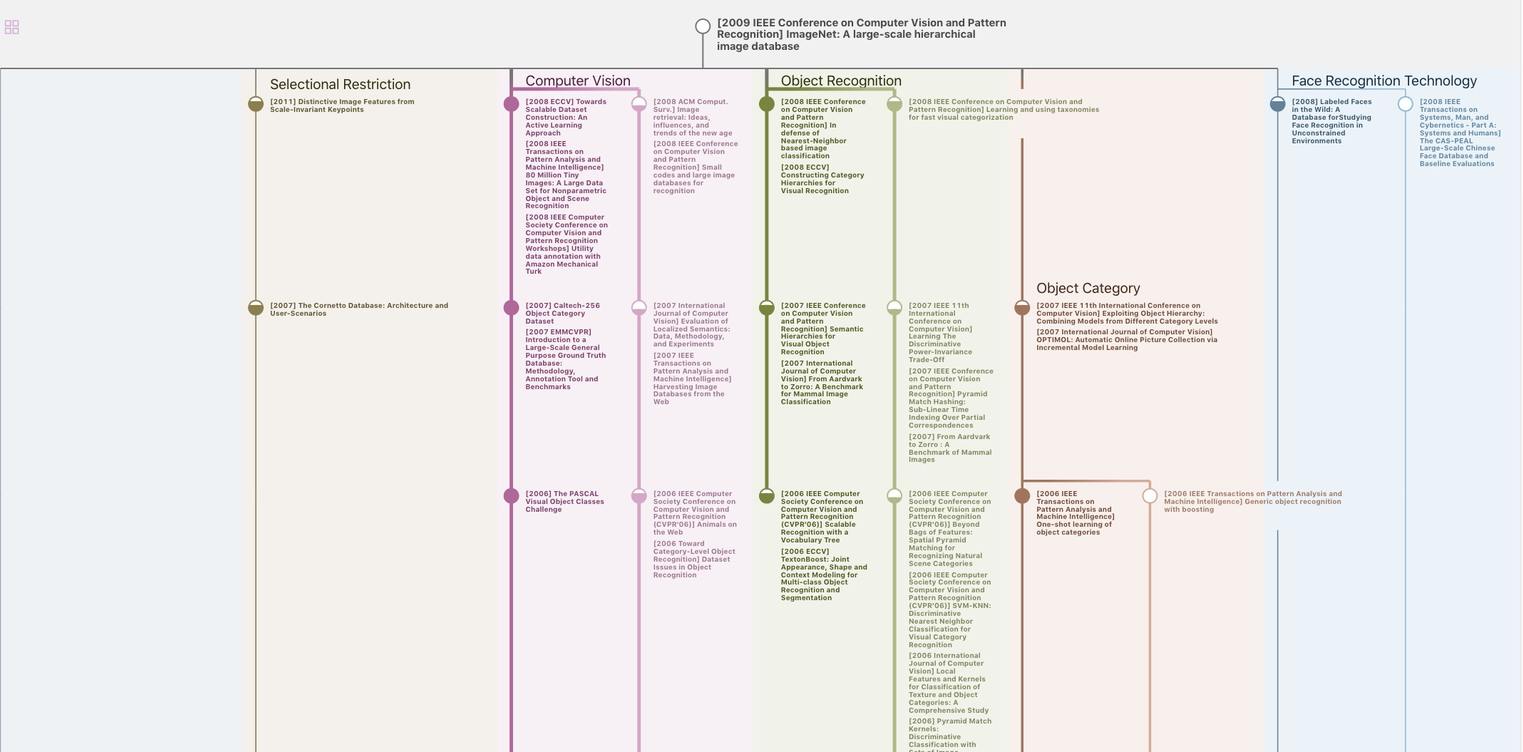
生成溯源树,研究论文发展脉络
Chat Paper
正在生成论文摘要