Clinical validation of an artificial intelligence algorithm offering cross-platform detection of atrial fibrillation using smart device electrocardiograms
Archives of Cardiovascular Diseases(2023)
摘要
Several smart devices are able to detect atrial fibrillation automatically by recording a single-lead electrocardiogram, and have created a work overload at the hospital level as a result of the need for over-reads by physicians. To compare the atrial fibrillation detection performances of the manufacturers’ algorithms of five smart devices and a novel deep neural network-based algorithm. We compared the rate of inconclusive tracings and the diagnostic accuracy for the detection of atrial fibrillation between the manufacturers’ algorithms and the deep neural network-based algorithm on five smart devices, using a physician-interpreted 12-lead electrocardiogram as the reference standard. Of the 117 patients (27% female, median age 65 years, atrial fibrillation present at time of recording in 30%) included in the final analysis (resulting in 585 analyzed single-lead electrocardiogram tracings), the deep neural network-based algorithm exhibited a higher conclusive rate relative to the manufacturer algorithm for all five models: 98% vs. 84% for Apple; 99% vs. 81% for Fitbit; 96% vs. 77% for AliveCor; 99% vs. 85% for Samsung; and 97% vs. 74% for Withings (P < 0.01, for each model). When applying our deep neural network-based algorithm, sensitivity and specificity to correctly identify atrial fibrillation were not significantly different for all assessed smart devices. In this clinical validation, the deep neural network-based algorithm significantly reduced the number of tracings labeled inconclusive, while demonstrating similarly high diagnostic accuracy for the detection of atrial fibrillation, thereby providing a possible solution to the data surge created by these smart devices.
更多查看译文
关键词
atrial fibrillation,artificial intelligence algorithm,artificial intelligence,clinical validation,cross-platform
AI 理解论文
溯源树
样例
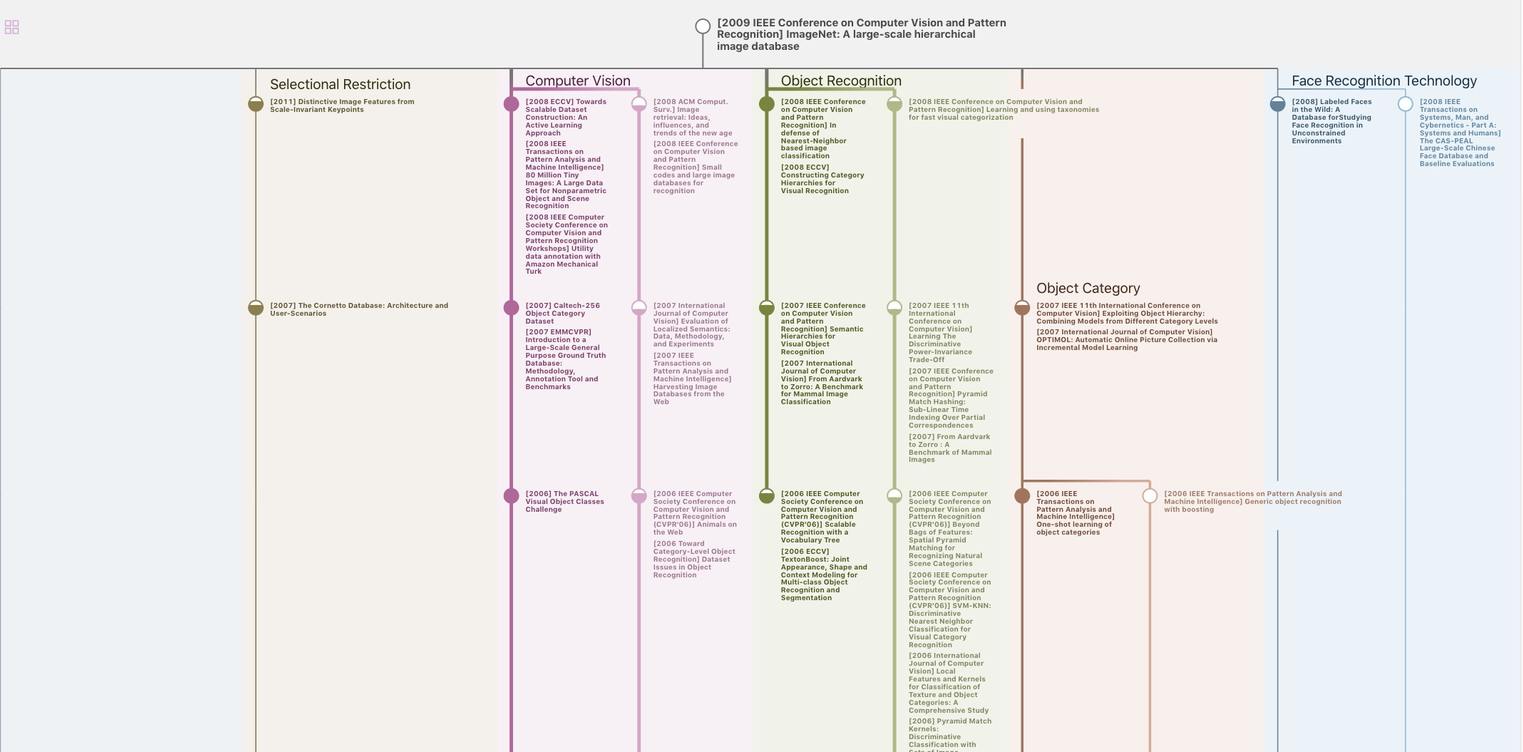
生成溯源树,研究论文发展脉络
Chat Paper
正在生成论文摘要