From Proper Scoring Rules to Max-Min Optimal Forecast Aggregation
Operations Research(2023)
摘要
There are many ways to elicit honest probabilistic forecasts from experts. Once those forecasts are elicited, there are many ways to aggregate them into a single forecast. Should the choice of elicitation method inform the choice of aggregation method? In “From Proper Scoring Rules to Max-Min Optimal Forecast Aggregation,” Neyman and Roughgarden establish a connection between these two problems. To every elicitation method they associate the aggregation method that improves as much as possible upon the forecast of a randomly chosen expert, in the worst case. This association maps the two most widely used elicitation methods (Brier and logarithmic scoring) to the two most well-known aggregation methods (linear and logarithmic pooling). The authors show a number of interesting properties of this connection, including a natural axiomatization of aggregation methods obtained through the connection, as well as an algorithm for efficient no-regret learning of expert weights. This paper forges a strong connection between two seemingly unrelated forecasting problems: incentive-compatible forecast elicitation and forecast aggregation. Proper scoring rules are the well-known solution to the former problem. To each such rule s , we associate a corresponding method of aggregation, mapping expert forecasts and expert weights to a “consensus forecast,” which we call quasi-arithmetic (QA) pooling with respect to s . We justify this correspondence in several ways: QA pooling with respect to the two most well-studied scoring rules (quadratic and logarithmic) corresponds to the two most well-studied forecast aggregation methods (linear and logarithmic); given a scoring rule s used for payment, a forecaster agent who subcontracts several experts, paying them in proportion to their weights, is best off aggregating the experts’ reports using QA pooling with respect to s , meaning this strategy maximizes its worst-case profit (over the possible outcomes); the score of an aggregator who uses QA pooling is concave in the experts’ weights (as a consequence, online gradient descent can be used to learn appropriate expert weights from repeated experiments with low regret); and the class of all QA pooling methods is characterized by a natural set of axioms (generalizing classical work by Kolmogorov on quasi-arithmetic means). Funding: This work was supported by the Division of Computing and Communication Foundations [Grant CCF-1813188], the Army Research Office [Grant W911NF1910294], and the Division of Graduate Education [Grant DGE-2036197]. Supplemental Material: The e-companion is available at https://doi.org/10.1287/opre.2022.2414 .
更多查看译文
关键词
forecast,aggregation,proper scoring rules,max-min
AI 理解论文
溯源树
样例
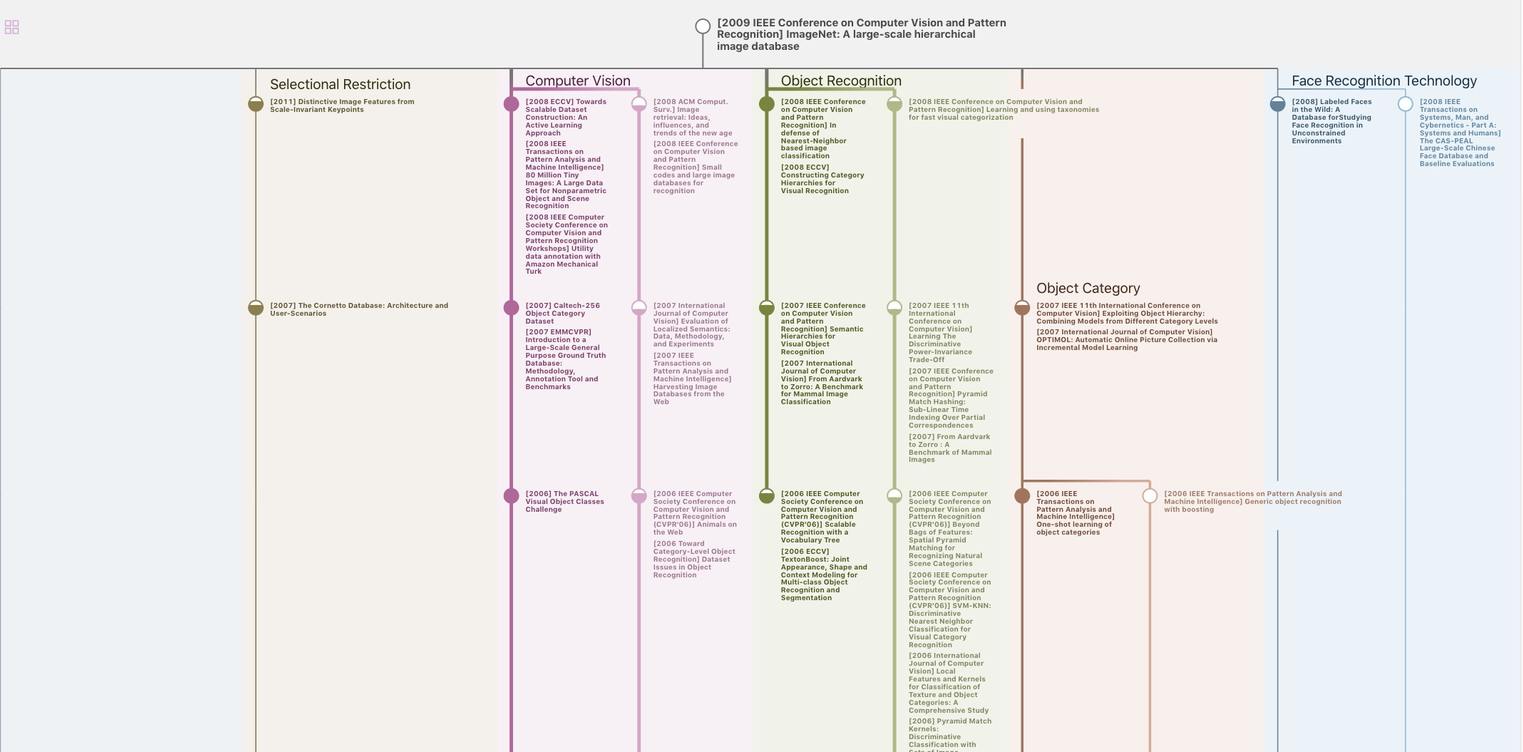
生成溯源树,研究论文发展脉络
Chat Paper
正在生成论文摘要