Predicting the risk of heatstroke: Development of a highly accurate model
Research Square (Research Square)(2023)
摘要
Abstract In Japan, a heat health warning system is in operation to reduce heat-related mortality and morbidity caused by global climate change. However, warnings are issued uniformly throughout the country under the same conditions, resulting in low accuracy in some areas. To reduce heat-related mortality and morbidity, accurate models for predicting the number of people transported by ambulance due to heatstroke, a precursor to death, must be developed. This study compared three methods for predicting heatstroke risk: multiple regression analysis, a generalised additive model, and time-stratified case-crossover analysis (TC). Heatstroke susceptibility is likely to depend on year-wise trends and sensitivity to training data. Most previous models, however, only tested a small amount of training data. In this study, we looked into the best number of years to use as training data. Error-influencing factors in the training data were identified by comparing the errors of each method. The TC errors were significantly less sensitive to the training data than the others (p <0.01). When data from three to four years after the most recent data were included, the accuracy of all three methods tended to decrease. Consequently, a highly accurate prediction model with low sensitivity to training data was developed using the odds ratios produced by TC, which was impossible with previous models. This modelling approach will help improve heat-health warning systems, reducing heatrelated mortality and morbidity and can be used to make each area safer in the future.
更多查看译文
关键词
heatstroke,predicting,risk
AI 理解论文
溯源树
样例
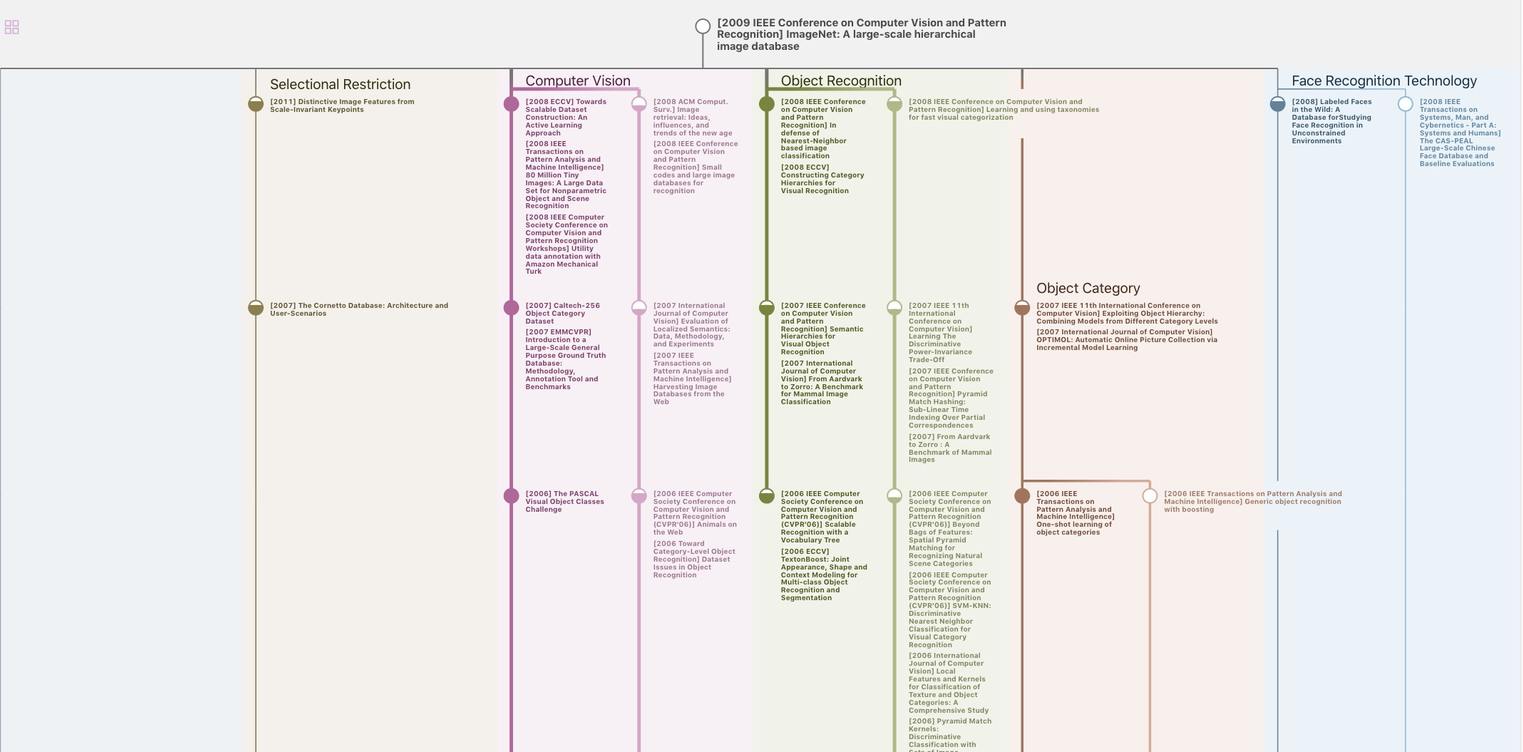
生成溯源树,研究论文发展脉络
Chat Paper
正在生成论文摘要