XCTF: A CNN-Based Interpretable Model for Multivariate Time Series Forecasting
Communications in computer and information science(2023)
摘要
Over the past decade, multivariate time series forecasting is becoming a research hotspot. Despite the emergence of several deep learning-based models achieve superior performance, the lack of credible explanations limits their usability in many fields. To bridge this gap, we propose an interpretable forecasting model called XCTF (eXplainable CNN for multivariate Time series Forecasting), which combines a Dual-Attention Module and a Saliency Detection Module. The Dual-Attention Module is leveraged to extract variable features related to prediction and extract temporal features from the input data. The Saliency Detection Module has two main parts: ConvDown and ConvUp block. The ConvDown block can filter out the small, redundant features but keep the important information. The ConvUp block can enlarge the extracted feature map to obtain more accurate prediction data. Then, by using a method based on Grad-CAM, XCTF can generate an attribution map of feature importance. The attribution map can precisely demonstrate the important information of the input data. Experimental results on three benchmark datasets (ETTh1, ETTm1, and Exchange) demonstrate that XCTF has high performance when compares with current mainstream models. Moreover, XCTF offers strong interpretability and practical relevance by providing an attribution map.
更多查看译文
关键词
multivariate time series forecasting,multivariate time series,cnn-based
AI 理解论文
溯源树
样例
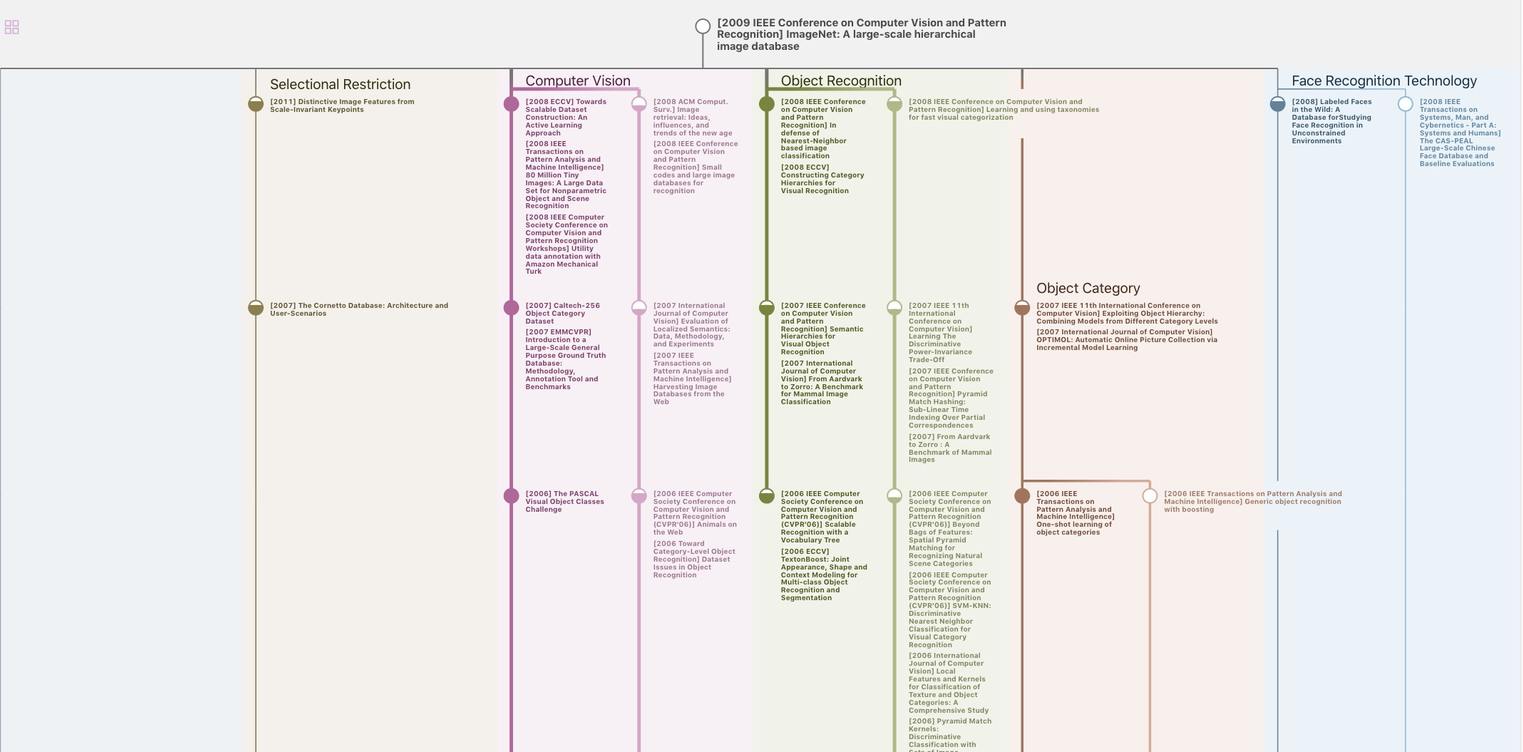
生成溯源树,研究论文发展脉络
Chat Paper
正在生成论文摘要