Prediction of Benign and Malignant Thyroid Nodules Based on Multicenter Ultrasound Images
Research Square (Research Square)(2023)
摘要
Abstract Purpose Thyroid cancer is a prevalent form of cancer, ranking ninth in terms of incidence rate worldwide. To leverage the advancements in deep learning for medical imaging, we successfully developed a dynamic integration model that combines the transformer and convolutional neural network (CNN) architectures to estimate benignity or malignancy. Methods We recruited 202 patients with thyroid nodules from Quzhou People’s Hospital and 102 patients from the public Thyroid Ultrasound Images (DDTI) dataset. We randomly divided the data into a training set (429 ultrasound images) and testing set (70 ultrasound images) at a 7:3 ratio. To address the inherent imbalance in the dataset, we employed a data augmentation strategy that adds 1 noise as a compensatory measure. A dynamic integration strategy, DiTNet, which is based on Vision Transformer, ResNet, and DenseNet, is proposed, using a CNN and self-attention mechanism to extract image features. Results To evaluate the performance of DiTNet, its relevant indicators were assessed based on the Receiver Operating Characteristic (ROC). ROC analysis revealed an area under the curve (AUC) of 0.95, accompanied by accuracy, sensitivity, and specificity values of 0.89. Conclusion DiTNet exhibited excellent performance in the face of imbalanced datasets and complex and diverse samples, verifying the effectiveness of data augmentation strategies and the ability of different basic models to learn different features.
更多查看译文
关键词
malignant thyroid nodules,thyroid nodules,ultrasound images
AI 理解论文
溯源树
样例
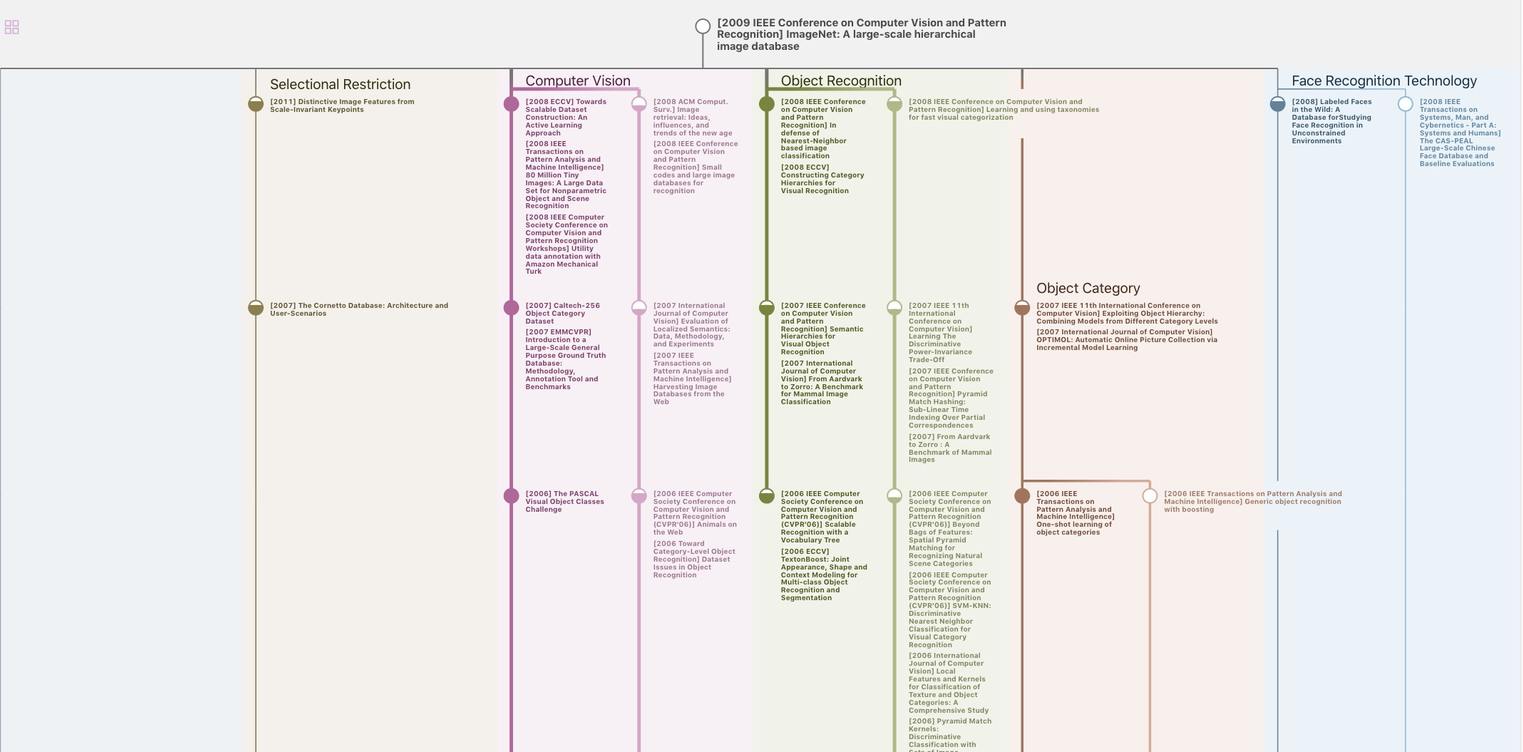
生成溯源树,研究论文发展脉络
Chat Paper
正在生成论文摘要