Inferring molecular Inhibition potency with AlphaFold predicted structures
Research Square (Research Square)(2023)
摘要
Abstract Even though in silico drug ligand-based methods have been successful in predicting interactions with known target proteins, they struggle with new, unassessed targets. To address this challenge, we propose an innovative approach that integrates structural data from AlphaFold 2-predicted protein structures into machine learning models. Our method extracts 3D structural protein fingerprints and combines them with ligand structural data to train a single machine learning model. This model captures the relationship between ligand properties and the unique structural features of various target proteins, enabling predictions for novel molecules and protein targets. To assess our model, we used a dataset of 144 Human G-protein Coupled Receptors (GPCRs) with over 140,000 measured inhibition constants (Ki) values. Our results demonstrate that our approach performs as well as state-of-the-art ligand-based methods, even for previously unencountered targets. In a test set of 15 protein targets with removed Ki information from the training data, our model predicted interactions for 73% of targets, with explained variances exceeding 0.50 in 22% of cases. In conclusion, our study presents an in silico drug development approach that bridges the gap between ligand-based methods and structural data integration for predicting protein-molecule interaction even for orphan targets.
更多查看译文
关键词
molecular inhibition potency,alphafold,structures
AI 理解论文
溯源树
样例
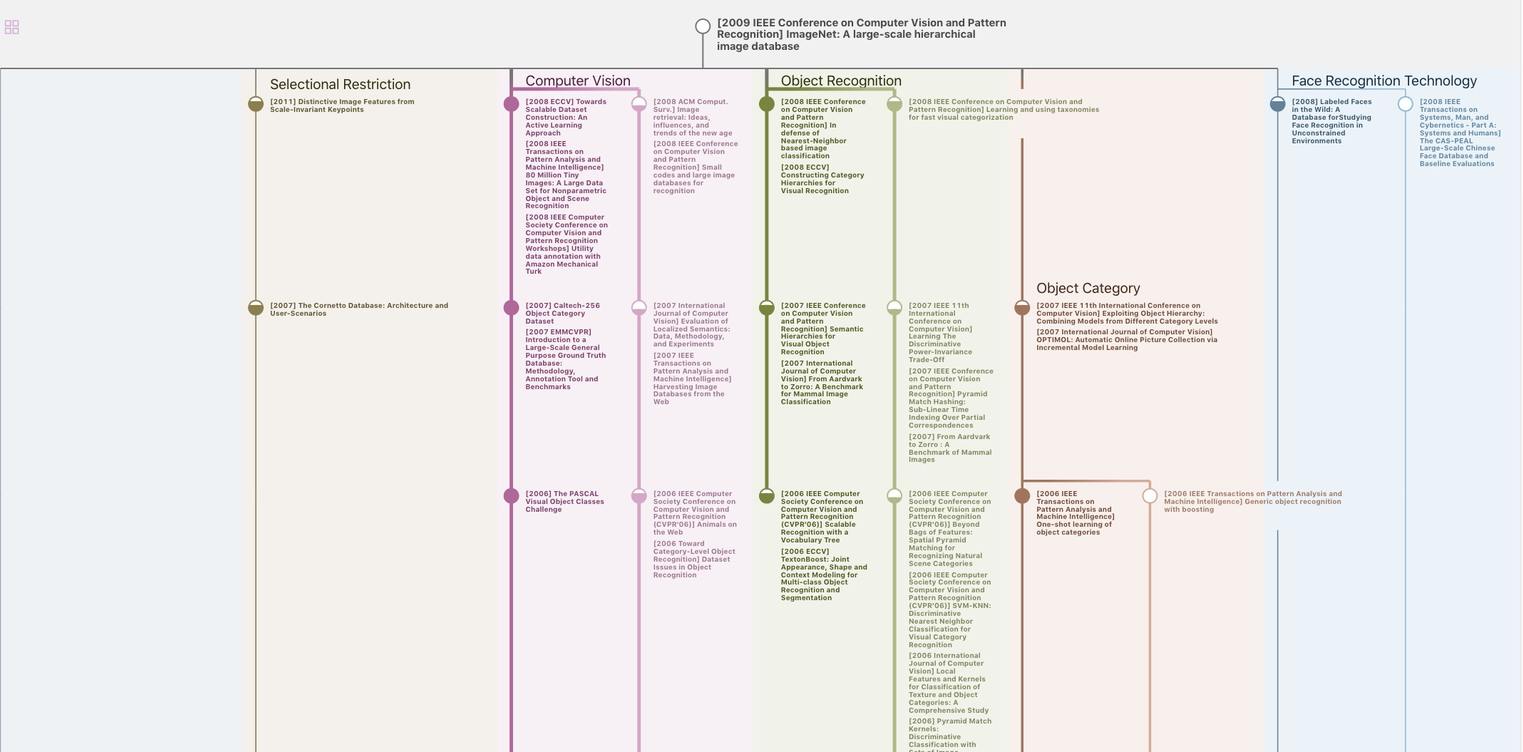
生成溯源树,研究论文发展脉络
Chat Paper
正在生成论文摘要