AlegAATr the Bandit
Frontiers in artificial intelligence and applications(2023)
摘要
One design strategy for developing intelligent agents is to create N distinct behaviors, each of which works effectively in particular tasks and circumstances. At each time step during task execution, the agent, or bandit, chooses which of the N behaviors to use. Traditional bandit algorithms for making this selection often (1) assume the environment is stationary, (2) focus on asymptotic performance, and (3) do not incorporate external information that is available to the agent. Each of these simplifications limits these algorithms such that they often cannot be used successfully in practice. In this paper, we propose a new bandit algorithm, called AlegAATr, as a step toward overcoming these deficiencies. AlegAATr leverages a technique called Assumption-Alignment Tracking (AAT), proposed previously in the robotics literature, to predict the performance of each behavior in each situation. It then uses these predictions to decide which behavior to use at any given time. We demonstrate the effectiveness of AlegAATr in selecting behaviors in three problem domains: repeated games, ad hoc teamwork, and a human-robot pick-n-place task.
更多查看译文
AI 理解论文
溯源树
样例
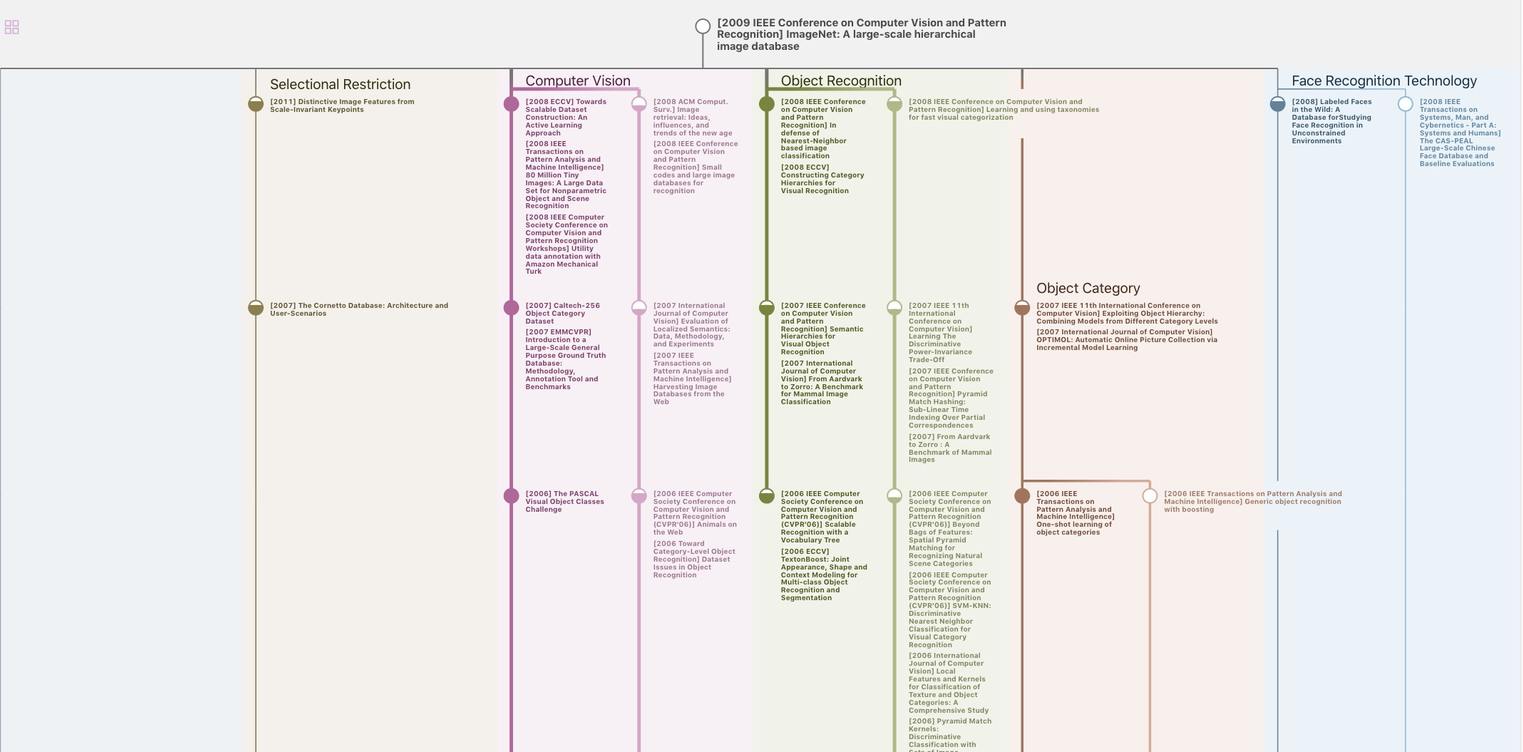
生成溯源树,研究论文发展脉络
Chat Paper
正在生成论文摘要