Ten Machine Learning Algorithms for Short-Term Forecasting: A Comparative Study in Gas Warning Systems
Research Square (Research Square)(2023)
摘要
Abstract This research aims to explore more efficient machine learning (ML) algorithms with better prediction assessments for short-term forecasting. Up-to-date literature shows a lack of research on selecting practical ML algorithms for short-term forecasting in real-time industrial applications. The literature reviews the top-tier publications on ML algorithms used in China’s industrial applications and finds 29 algorithms. Among them, ten widely used ML algorithms are tested, including ARIMA, BP_Resilient, BP_SOG, KNN, LP, LSTM, Perceptron, RF, RNN, and SVM. A case study is conducted to compare the prediction error and predictive performance assessments. The top two ranked algorithms for prediction error assessment are ARIMA and LR. RF, SVM, BP_SOG, KNN, RNN, and BP_Resilient follow. Perceptron is the last ranked algorithm. For predictive performance assessment, ten algorithms are divided into five clusters: the best (KNN) with the shortest computational time (0.41683s), better (RF, LR, and SVM) with between 1s and 2s, good (Perceptron, BP_SOG, and BP_Resilient) with between 2s and 3s, worse (ARIMA and RNN) with more than 3s, and the worst (LSTM) with the longest computational time (145.19s). Based on the outcomes of comparative analysis, ten ML algorithms can be finally classified into four categories: optimal, efficient, suboptimal, and inefficient. LR and RF are optimal algorithms. Efficient algorithms include SVM, KNN, and ARIMA. Suboptimal algorithms include BP_SOG, BP_Resilient, RNN, and Perceptron. LSTM is an inefficient algorithm. This research finds different results from previous studies between LSTM, KNN, and SVM. The research outcomes significantly explore different views on the performance of ARIMA, LR, KNN, RF, RNN, and SVM compared to previous studies. They are valuable for further research. Finally, this research contributes 20 research questions for further investigation.
更多查看译文
关键词
forecasting,gas warning systems,machine learning algorithms,warning systems,short-term
AI 理解论文
溯源树
样例
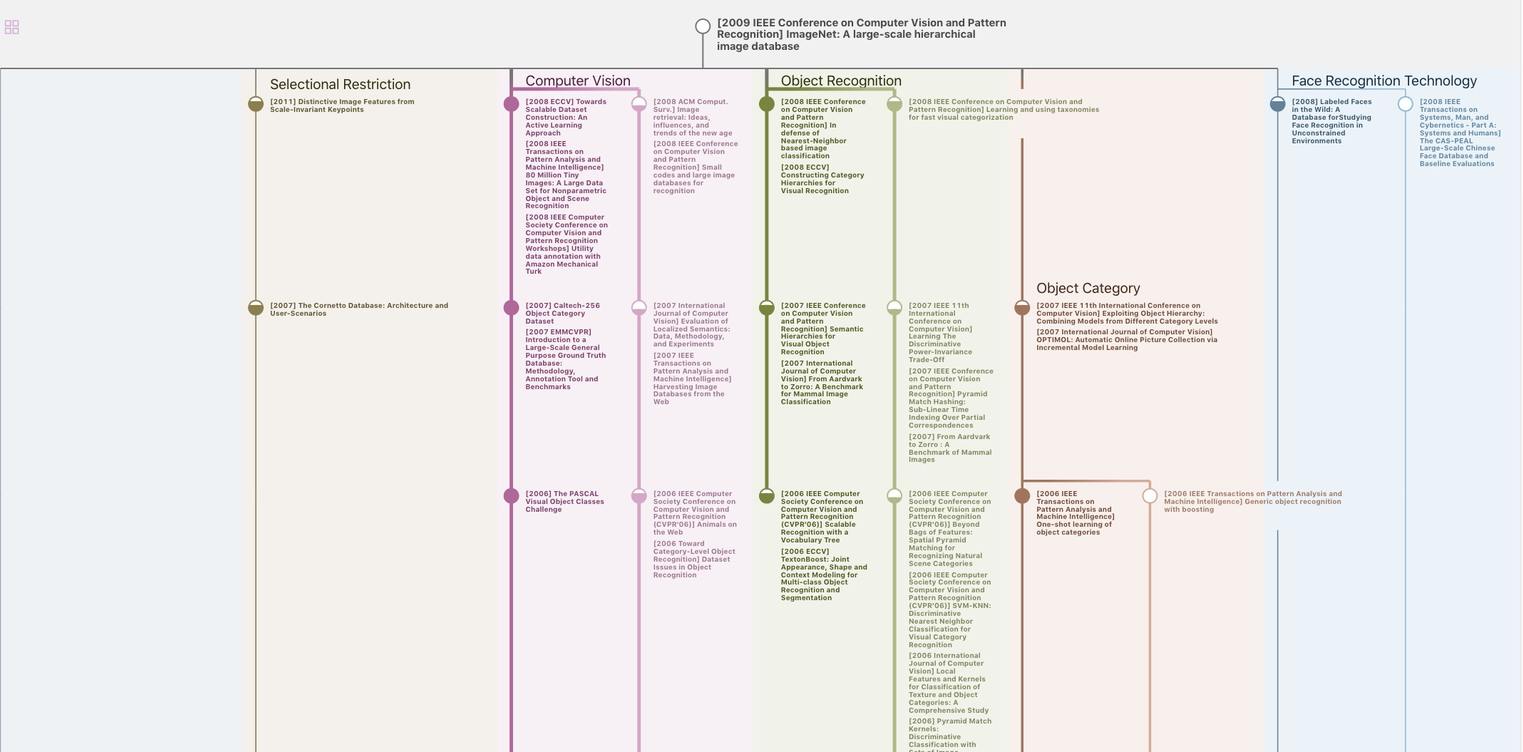
生成溯源树,研究论文发展脉络
Chat Paper
正在生成论文摘要