CTA-Net: A gaze estimation network based on dual feature aggregation and attention cross fusion
Computer Science and Information Systems(2024)
摘要
Recent work has demonstrated the Transformer model is effective for computer vision tasks. However, the global self-attention mechanism utilized in Transformer models does not adequately consider the local structure and details of images, which may result in the loss of information and local details, causing decreased estimation accuracy in gaze estimation tasks when compared to convolution or sequential stacking methods. To address this issue, we propose a parallel CNNs-Transformer aggregation network (CTA-Net) for gaze estimation, which fully leverages the advantages of the Transformer model in modeling global context while the convolutional neural networks (CNNs) model in retaining local details. Specifically, Transformer and ResNet are deployed to extract facial and eye information, respectively. Additionally, an attention cross fusion (ACFusion) Block is embedded with CNN branch, which decomposes features in space and channels to supplement lost features, suppress noise, and help extract eye features more effectively. Finally, a dual-feature aggregation (DFA) module is proposed to effectively fuse the output features of both branches with the help feature a selection mechanism and a residual structure. Experimental results on the MPIIGaze and Gaze360 datasets demonstrate that our CTA-Net achieves state-of-the-art results.
更多查看译文
关键词
gaze estimation network,dual feature aggregation,attention,cta-net
AI 理解论文
溯源树
样例
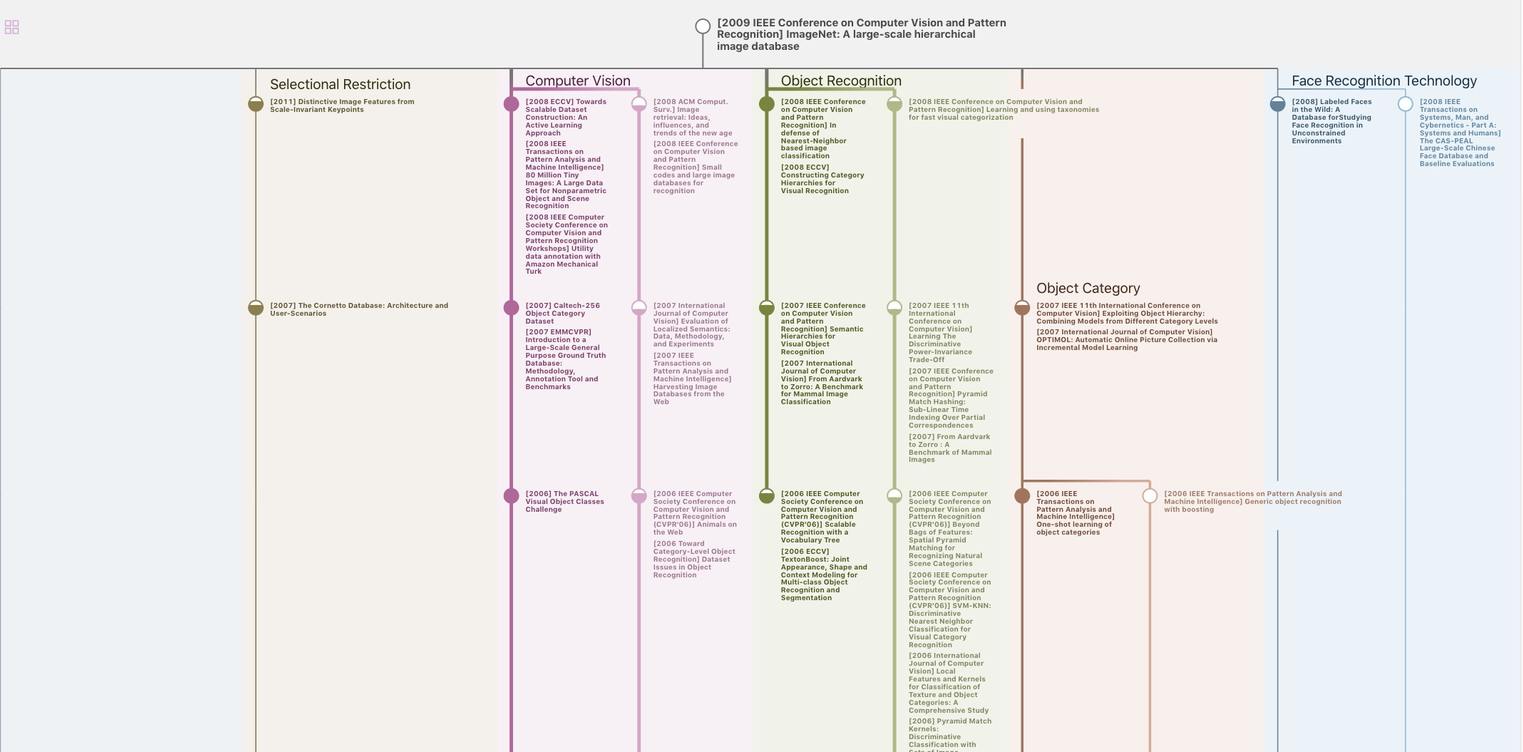
生成溯源树,研究论文发展脉络
Chat Paper
正在生成论文摘要