Deep neural network potential for simulating hydrogen blistering in tungsten
Physical Review Materials(2023)
摘要
Tungsten is a promising candidate for the plasma-facing material in fusion energy facilities, however, the low-energy, high-flux hydrogen plasma causes severe blistering in tungsten, which gives rise to safety concerns. By far, the formation mechanism of intragranular hydrogen blisters is still unclear. Large-scale atomistic simulations are crucial for improving the understanding, however, the available empirical interatomic potentials are mostly defective in predicting the formation of hydrogen self-clusters in tungsten, thus may lead to wrong blister formation mechanisms. In this work, we develop a machine-learning potential, DP-WH, for the tungsten-hydrogen binary system based on the Deep Potential method. We demonstrate that the DP-WH potential is able to describe, as accurately as ab initio calculations, the basic properties of bcc tungsten, the solute hydrogen properties in tungsten, adsorption and migration of hydrogen on tungsten free surfaces, interactions between hydrogen atoms and vacancy, dislocations, the interaction between neighboring interstitial hydrogen atoms, and the formation energy of H self-clusters. By using DP-WH, we perform nanosecond-long molecular dynamics simulations and report the formation of planar self-cluster of tetrahedral-interstitial-site hydrogen atoms normal to ${001}$ tungsten planes at a hydrogen concentration of $\ensuremath{\approx}10$ at.%. This form of the H self-cluster is highly possible to be the early nucleates of the crack-shaped H blisters observed in recent experiments. The DP-WH is thus proven as a good candidate potential for the atomistic simulations to unveil the formation mechanisms of the intragranular hydrogen blisters in tungsten under the relevant working conditions.
更多查看译文
关键词
hydrogen blistering,tungsten,deep neural network potential,neural network
AI 理解论文
溯源树
样例
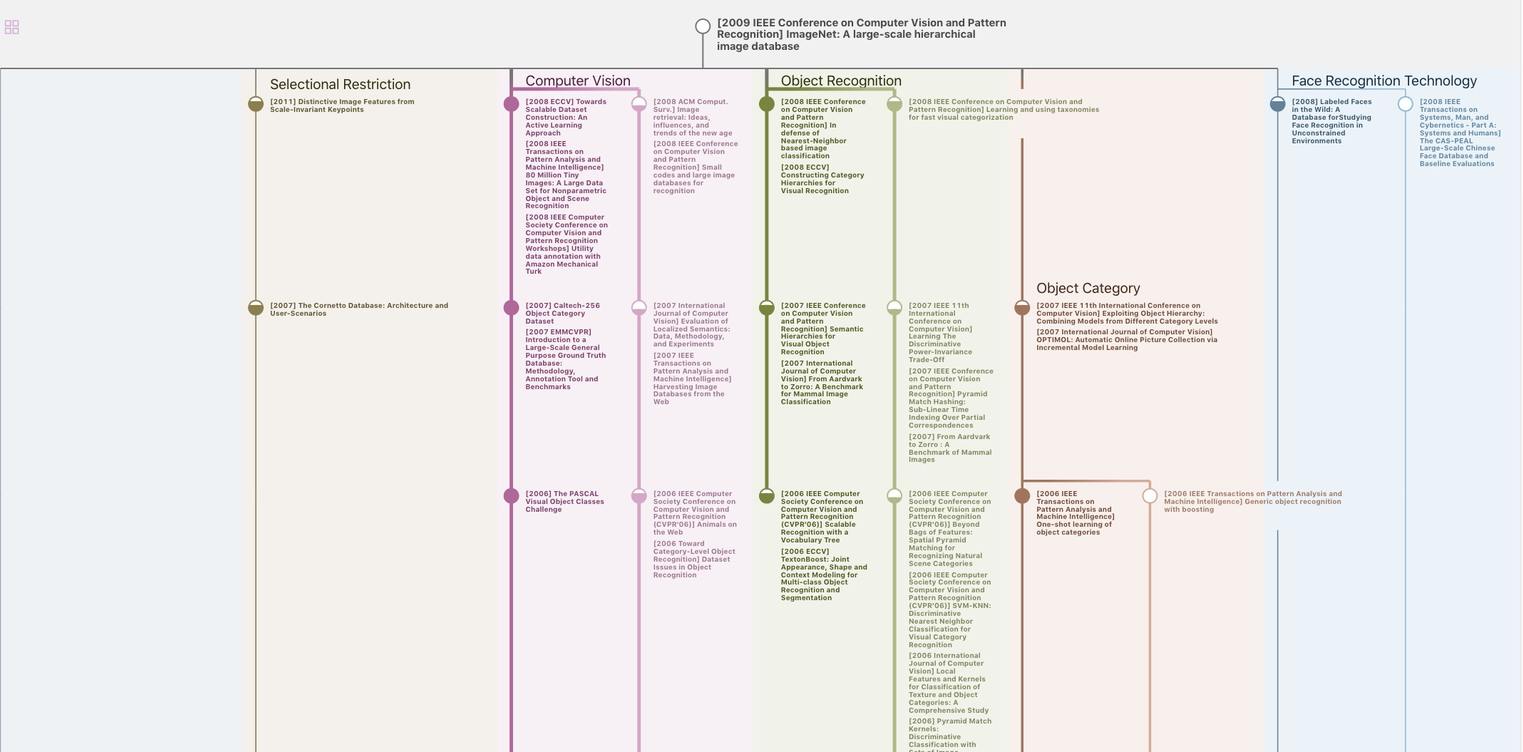
生成溯源树,研究论文发展脉络
Chat Paper
正在生成论文摘要