Empirical modelling and prediction of neuronal dynamics
Research Square (Research Square)(2023)
摘要
Abstract Mathematical modelling of neuronal dynamics has experienced a fast growing in the last decades thanks to the biophysical formalism introduced by Hodgkin and Huxley in the 1950's. Other types of models (for instance, integrate and fire models), although less realistic, have also contributed to understand population dynamics. However, there is still a vast volume of data orphan of model, mainly because data is acquired more rapidly than it can be analyzed or because it is difficult to analyze (for instance, if the number of ionic channels involved is huge). Therefore, developing new methodologies to obtain mathematical or computational models associated to data (even without previous knowledge of the source) can be helpful to make future predictions. Here, we explore the identification of neuronal (single-cell) voltage traces with artificial neural networks (ANN). We present an optimized computational scheme that trains the ANN with biologically plausible input currents. We obtain successful identification for data generated from four different neuron models. We also show that the empiric model obtained is able to generalize and predict the neuronal dynamics generated by variable input currents different from those used to train the artificial network. The resulting software (publicly available) can be used to obtain empiric models from experimental voltage traces obtained from known input current time traces.
更多查看译文
关键词
empirical modelling,dynamics,prediction
AI 理解论文
溯源树
样例
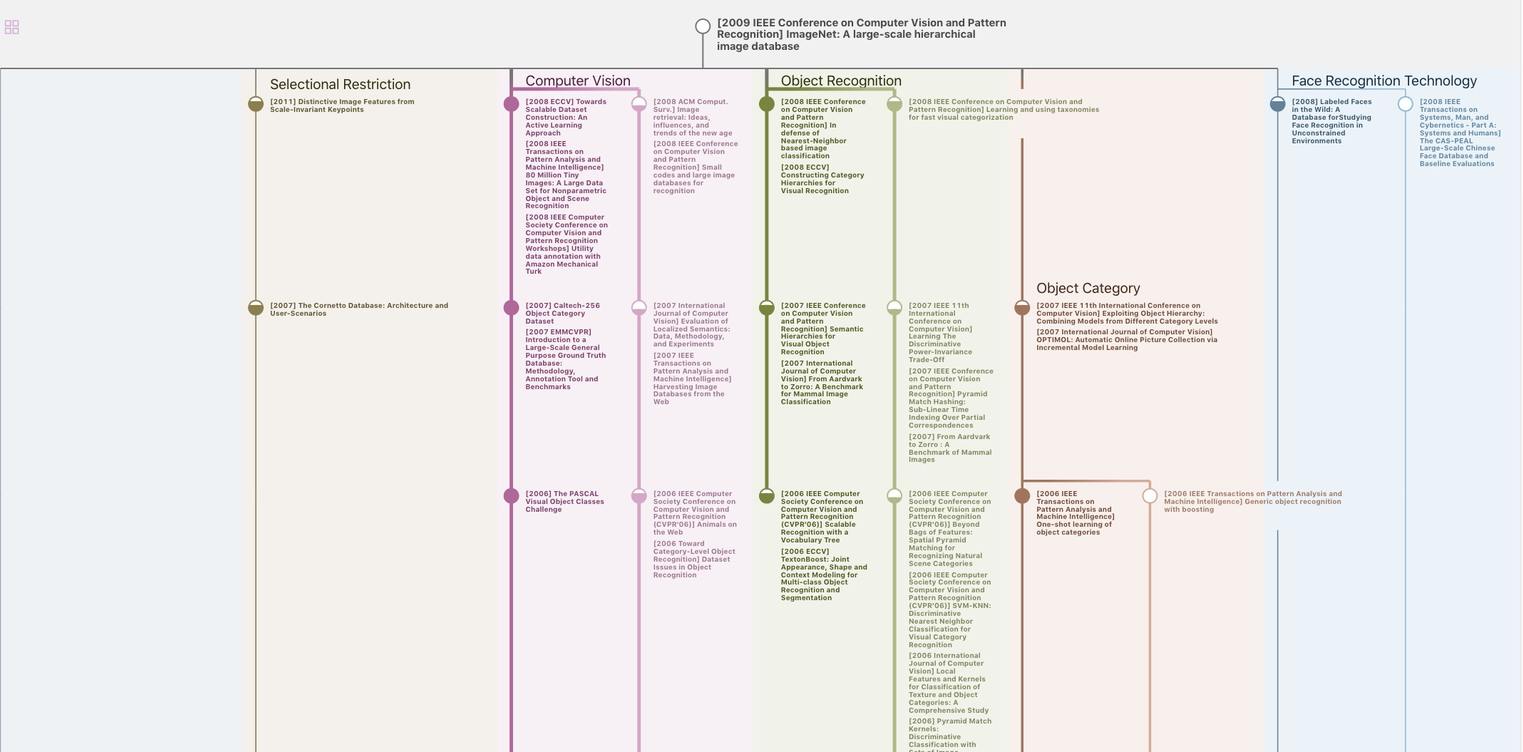
生成溯源树,研究论文发展脉络
Chat Paper
正在生成论文摘要