ChatENT: Augmented Large Language Model for Expert Knowledge Retrieval in Otolaryngology - Head and Neck Surgery
medRxiv (Cold Spring Harbor Laboratory)(2023)
摘要
Abstract Background The recent surge in popularity of Large Language Models (LLMs), such as ChatGPT, has showcased their proficiency in medical examinations and potential contributions to medical education. However, LLMs possess inherent limitations, including inconsistent accuracy, specific prompting requirements, and the risk of generating harmful hallucinations. A domain-specific, fine-tuned model would address these limitations effectively. Methods OHNS-relevant data was systematically gathered from open-access internet sources and indexed into a database. We leveraged Retrieval-Augmented Language Modeling (RALM) to recall this information and used it for pre-training, which was then integrated into ChatGPT 4·0, creating a OHNS specific knowledge Q&A platform known as ChatENT. Findings ChatENT showed enhanced performance in the analysis and interpretation of OHNS information, outperforming ChatGPT 4.0 in both the Canadian Royal College OHNS sample examination questions challenge and the US board practice questions challenge, with a 58.4% and 26·0% error reduction, respectively. ChatENT generated fewer hallucinations and demonstrated greater consistency. Interpretation To the best of our knowledge, ChatENT is the first specialty-specific LLM in the medical field. It appears to have considerable promise in areas such as medical education, patient education, and clinical decision support. The fine-tuned model has demonstrated the capacity to overcome the limitations of existing LLMs, thereby signaling a future of more precise, safe, and user-friendly applications in the realm of OHNS. Funding The authors received no financial support for the research, authorship, and/or publication of this project.
更多查看译文
关键词
expert knowledge retrieval,augmented large language model,otolaryngology,neck surgery
AI 理解论文
溯源树
样例
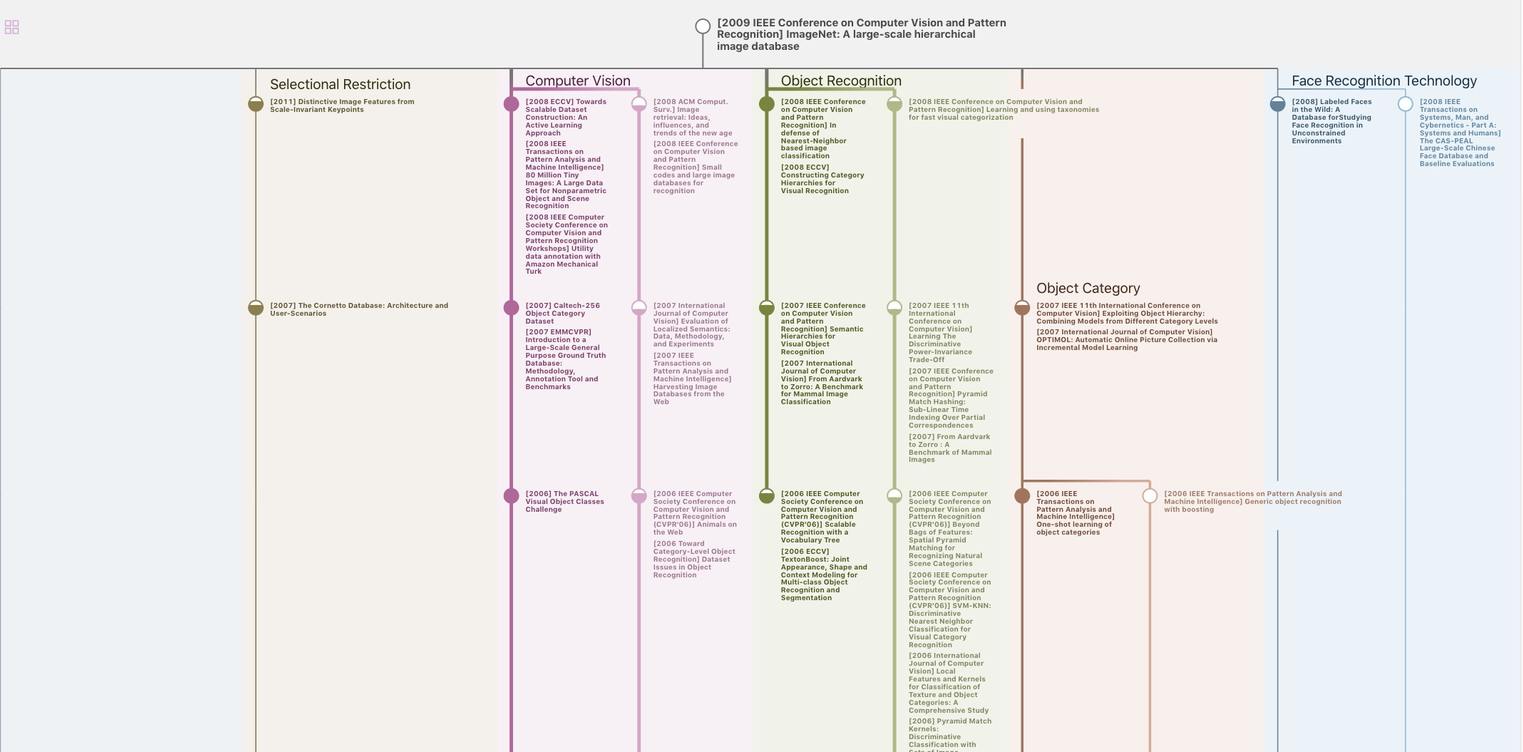
生成溯源树,研究论文发展脉络
Chat Paper
正在生成论文摘要